Deep-IRTarget: An Automatic Target Detector in Infrared Imagery Using Dual-Domain Feature Extraction and Allocation
IEEE TRANSACTIONS ON MULTIMEDIA(2022)
摘要
Recently, convolutional neural networks (CNNs) have brought impressive improvements for object detection. However, detecting targets in infrared images still remains challenging, because the poor texture information, low resolution and high noise levels of the thermal imagery restrict the feature extraction ability of CNNs. In order to deal with these difficulties in the feature extraction, we propose a novel backbone network named Deep-IRTarget, composing of a frequency feature extractor, a spatial feature extractor and a dual-domain feature resource allocation model. Hypercomplex Infrared Fourier Transform is developed to calculate the infrared intensity saliency by designing hypercomplex representations in the frequency domain, while a convolutional neural network is invoked to extract feature maps in the spatial domain. Features from the frequency domain and spatial domain are stacked to construct Dual-domain features. To efficiently integrate and recalibrate them, we propose a Resource Allocation model for Features (RAF). The well-designed channel attention block and position attention block are used in RAF to respectively extract interdependent relationships among channel and position dimensions, and capture channel-wise and position-wise contextual information. Extensive experiments are conducted on three challenging infrared imagery databases. We achieve 10.14%, 9.1% and 8.05% improvement on mAP scores, compared to the current state of the art method on MWIR, BITIR and WCIR respectively.
更多查看译文
关键词
Convolutional neural networks, feature extraction, infrared imagery, object detection
AI 理解论文
溯源树
样例
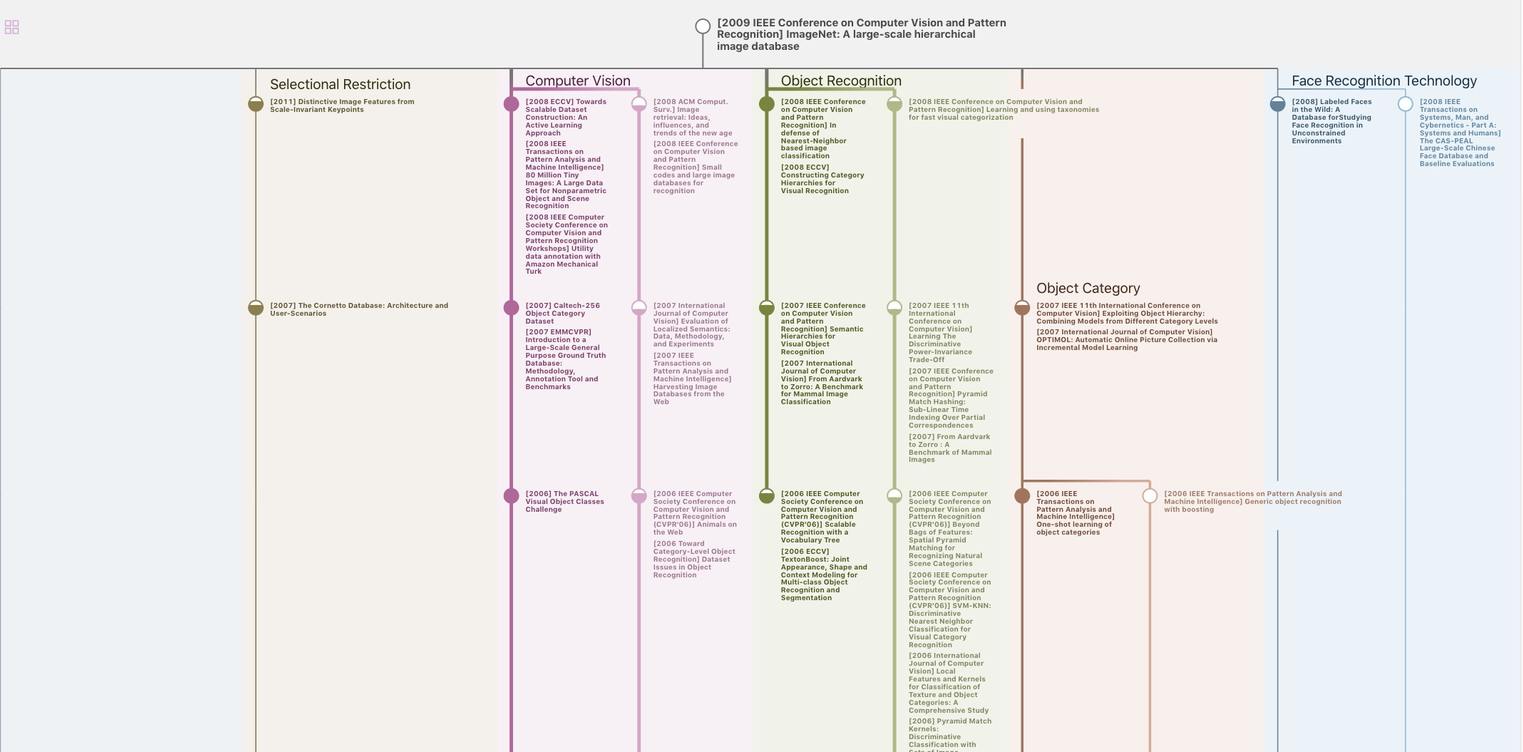
生成溯源树,研究论文发展脉络
Chat Paper
正在生成论文摘要