A Feature Fusion Framework And Its Application To Automatic Seizure Detection
IEEE SIGNAL PROCESSING LETTERS(2021)
摘要
Automatic analysis of biomedical signals plays an important role in the auxiliary diagnosis of diseases. Traditional methods extract hand-crafted features by imitating doctors' experience, while recent methods focus on extracting deep features automatically by designing the architectures of deep neural networks (DNNs). Combining these two kinds of features can not only take advantage of doctors' experience but also mine the hidden information in the raw data. But directly combining these features by fully connected layers may cause complex optimization hyper-planes. To better integrate doctors' experience and deep features that doctors can hardly describe, we propose a feature fusion framework named hybrid plus framework (HPF) and apply this framework to seizure detection. HPF mainly consists of two parts: (1) the FET module, where hand-crafted features are extracted and transformed to sparse categorical features; (2) the enhanced DNN, which contains a carefully designed neural network structure with the input being original signals and sparse categorical features. Experiments on the dataset of CHB-MIT show that HPF outperforms the state-of-the-art methods. Further experiments indicate that HPF is very flexible as many of its modules can be replaced.
更多查看译文
关键词
Feature extraction, Medical services, Transforms, Training, Optimization, Indexes, Deep learning, Feature fusion, hand-crafted features, deep features, seizure detection
AI 理解论文
溯源树
样例
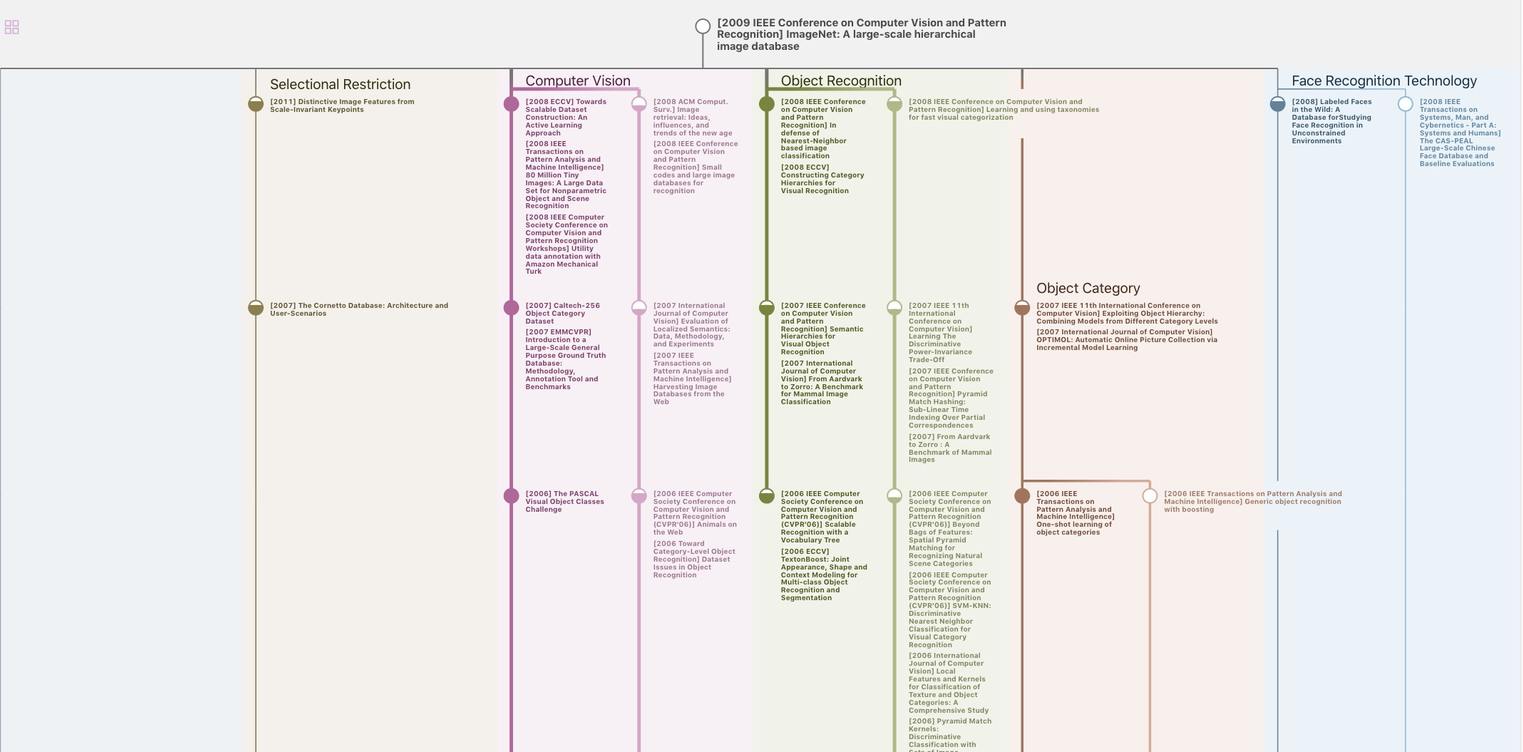
生成溯源树,研究论文发展脉络
Chat Paper
正在生成论文摘要