FrequencySpace-Dependent Smoothing Regularized Nonstationary Predictive Filtering
IEEE TRANSACTIONS ON GEOSCIENCE AND REMOTE SENSING(2022)
摘要
Predictive filtering is one of the most widely used denoising algorithms in the seismic data processing community because of its high efficiency and stability in different situations. The traditional predictive filtering, however, is not able to deal with structurally complex data set unless applied in local windows. We develop a novel noncausal predictive filtering method that is free of the windowing step but is able to denoise complicated data set. We extend the stationary predictive filtering method to its nonstationary version, where the predictive filter coefficients vary across the frequency-space domain. The nonstationary predictive filtering (NPF) model requires solving a highly underdetermined inverse problem using an iterative shaping regularization method. The traditional shaping regularization method solves an inverse problem by applying a constant smoothing operator and thus does not consider the heterogeneity of the filter coefficients in the frequency-space domain. We propose to apply a nonstationary smoothing operator to constrain the model in the shaping regularization framework. The smoothing radius in the nonstationary smoothing operator is chosen based on a priori information of the model, e.g., the nonstationarity of the data in the frequency-space domain. The proposed NPF method offers the flexibility in controlling the smoothness and sharpness of the calculated filter coefficients in both frequency and space dimensions. Several synthetic data sets and complicated real data examples are used to demonstrate the advantages of the new method.
更多查看译文
关键词
Smoothing methods, Transforms, Noise reduction, Mathematical model, Frequency-domain analysis, Predictive models, Inverse problems, Predictive filtering, seismic data, signa processing
AI 理解论文
溯源树
样例
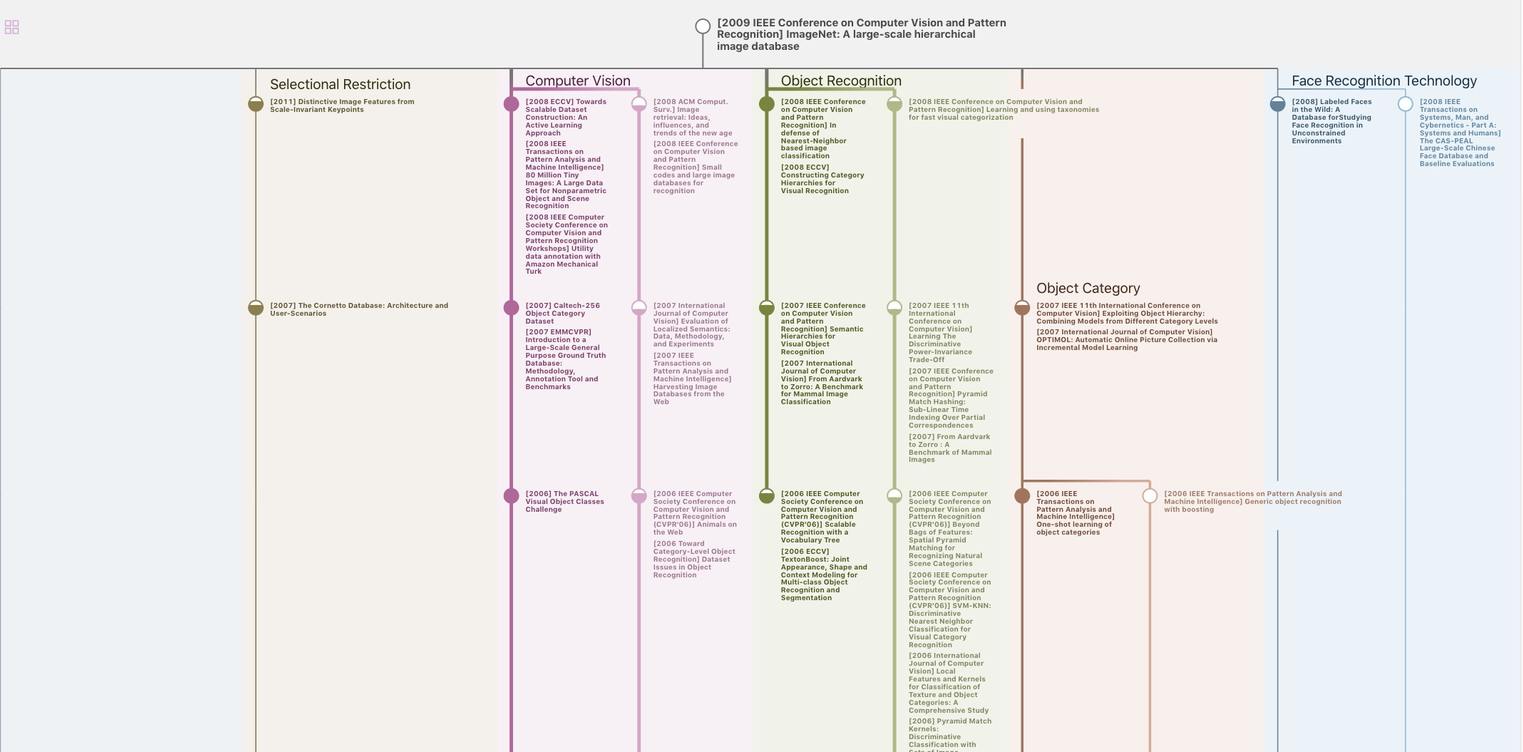
生成溯源树,研究论文发展脉络
Chat Paper
正在生成论文摘要