The Nearest Polyhedral Convex Conic Regions For High-Dimensional Classification
TURKISH JOURNAL OF ELECTRICAL ENGINEERING AND COMPUTER SCIENCES(2021)
摘要
In the nearest-convex-model type classifiers, each class in the training set is approximated with a convex class model, and a test sample is assigned to a class based on the shortest distance from the test sample to these class models. In this paper, we propose new methods for approximating the distances from test samples to the convex regions spanned by training samples of classes. To this end, we approximate each class region with a polyhedral convex conic region by utilizing polyhedral conic functions (PCFs) and its extension, extended PCFs. Then, we derive the necessary formulations for computing the distances from test samples to these new models. We tested the proposed methods on different high-dimensional classification tasks including face, digit, and generic object classification as well as on some lower-dimensional classification problems. The experimental results on different datasets show that the proposed classifiers achieve either the best or comparable results on high-dimensional classification problems compared to other nearest-convex-model classifiers, which shows the superiority of the proposed methods.
更多查看译文
关键词
Classification, polyhedral conic region, affine hull, convex hull, convex cone, face recognition
AI 理解论文
溯源树
样例
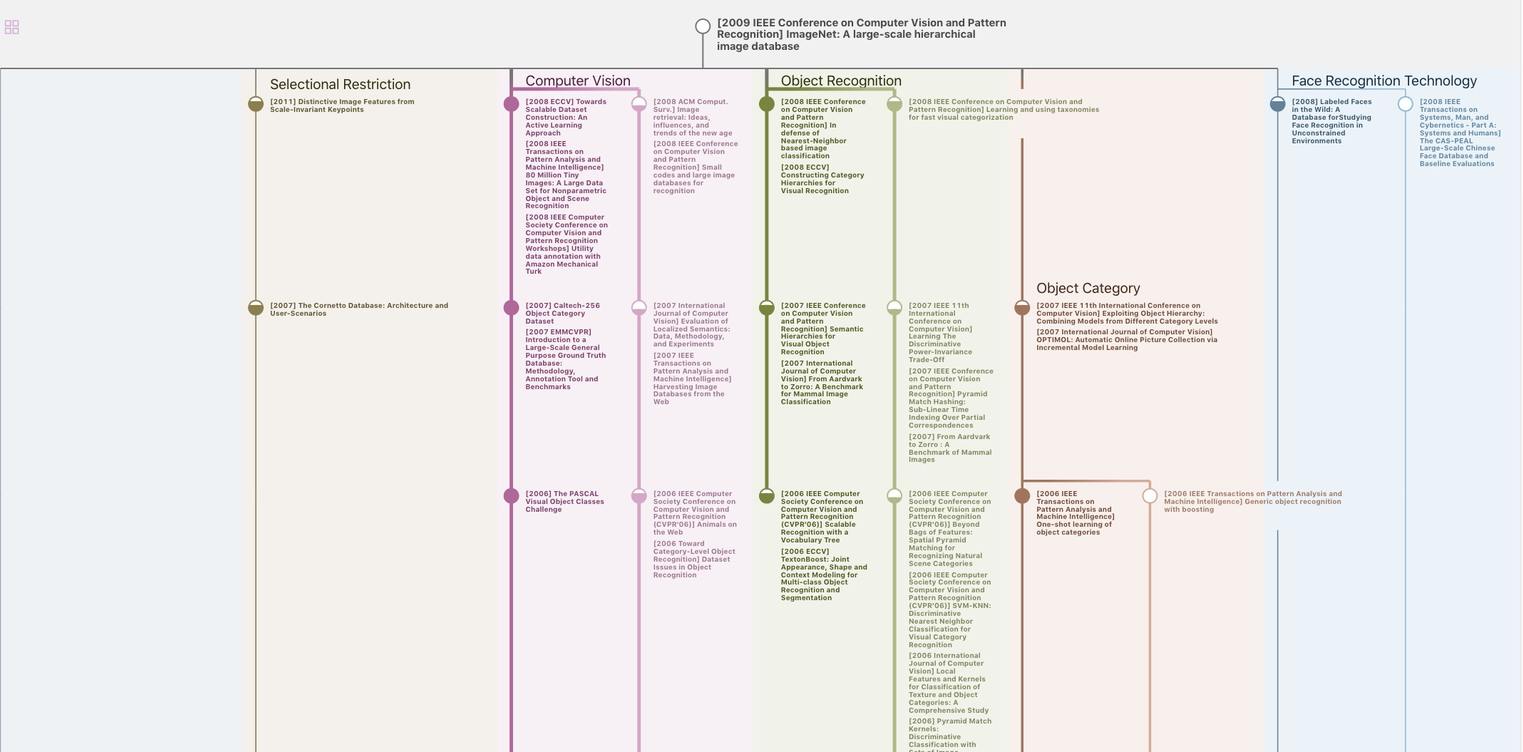
生成溯源树,研究论文发展脉络
Chat Paper
正在生成论文摘要