Improving Model Capacity Of Quantized Networks With Conditional Computation
ELECTRONICS(2021)
摘要
Network quantization becomes a crucial step when deploying deep models to the edge devices as it is hardware-friendly, offers memory and computational advantages, but it also suffers performance degradation as the result of limited representation capability. We address this issue by introducing conditional computing to low-bit quantized networks. Instead of using a fixed, single kernel for each layer, which usually does not generalize well across all input data, our proposed method tries to use multiple parallel kernels dynamically in conjunction with the winner-takes-all gating mechanism to select the best one to propagate information. Overall, our proposed method improves upon the prior work, without adding much computational overhead, results in better classification performance on the CIFAR-10 and CIFAR-100 datasets.
更多查看译文
关键词
quantized networks, model compression, dynamic neural network, conditional computing, model capacity, model representation, machine learning, VLSI
AI 理解论文
溯源树
样例
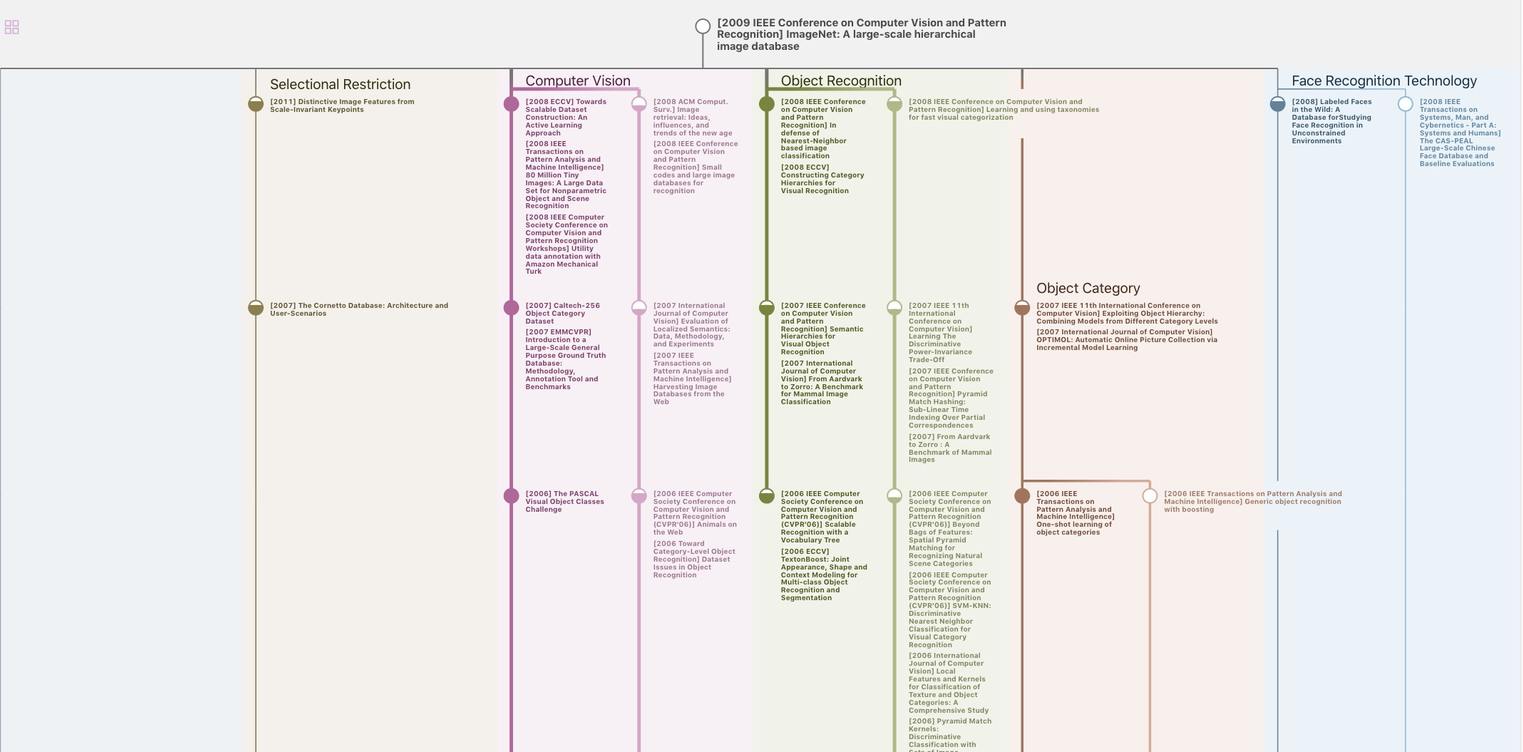
生成溯源树,研究论文发展脉络
Chat Paper
正在生成论文摘要