Quantifying patient and neighborhood risks for stillbirth and preterm birth in Philadelphia with a Bayesian spatial model
arXiv (Cornell University)(2021)
摘要
Stillbirth and preterm birth are major public health challenges. Using a
Bayesian spatial model, we quantified patient-specific and neighborhood risks
of stillbirth and preterm birth in the city of Philadelphia. We linked birth
data from electronic health records at Penn Medicine hospitals from 2010 to
2017 with census-tract-level data from the United States Census Bureau. We
found that both patient-level characteristics (e.g. self-identified
race/ethnicity) and neighborhood-level characteristics (e.g. violent crime)
were significantly associated with patients' risk of stillbirth or preterm
birth. Our neighborhood analysis found that higher-risk census tracts had 2.68
times the average risk of stillbirth and 2.01 times the average risk of preterm
birth compared to lower-risk census tracts. Higher neighborhood rates of women
in poverty or on public assistance were significantly associated with greater
neighborhood risk for these outcomes, whereas higher neighborhood rates of
college-educated women or women in the labor force were significantly
associated with lower risk. Several of these neighborhood associations were
missed by the patient-level analysis. These results suggest that
neighborhood-level analyses of adverse pregnancy outcomes can reveal nuanced
relationships and, thus, should be considered by epidemiologists. Our findings
can potentially guide place-based public health interventions to reduce
stillbirth and preterm birth rates.
更多查看译文
AI 理解论文
溯源树
样例
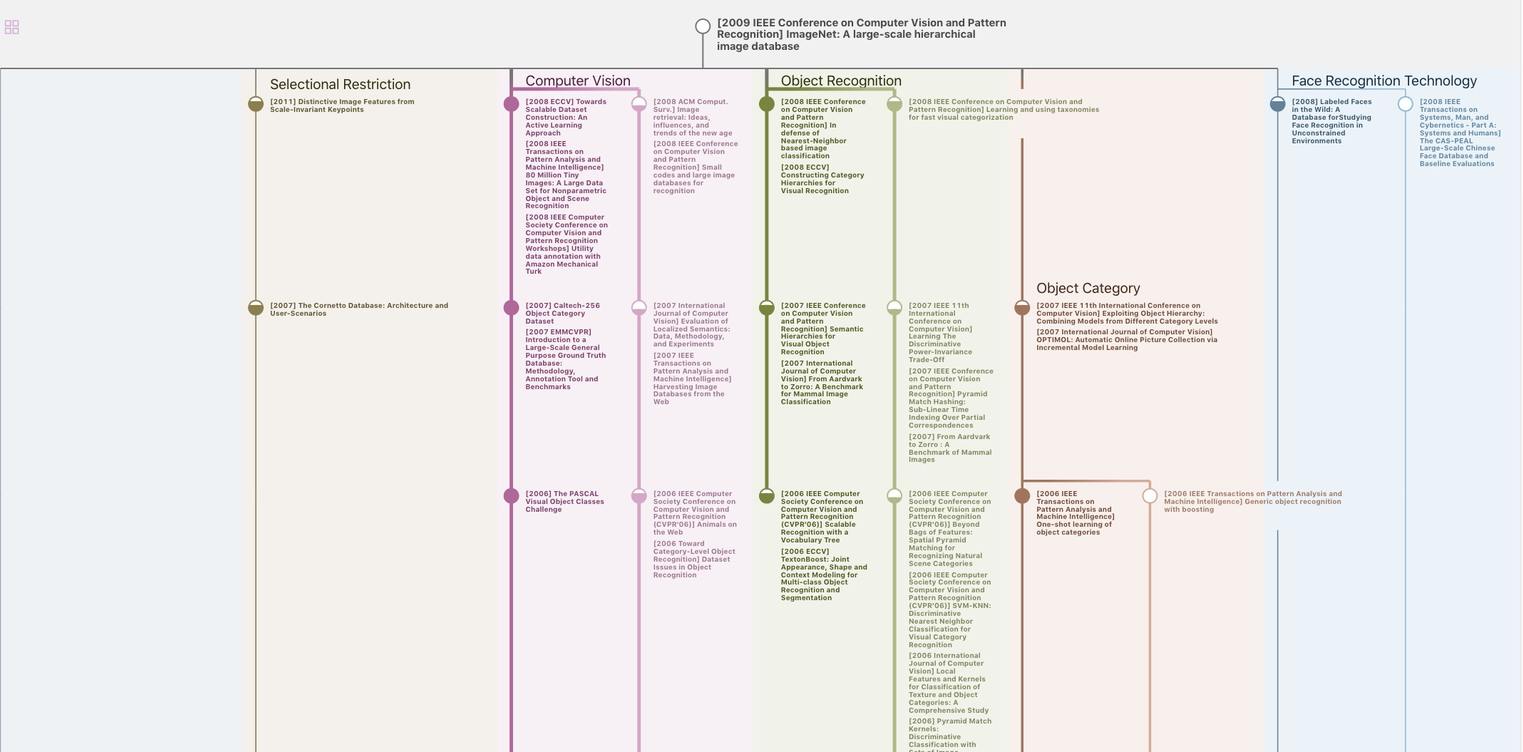
生成溯源树,研究论文发展脉络
Chat Paper
正在生成论文摘要