Thematic fit bits: Annotation quality and quantity for event participant representation
arxiv(2021)
摘要
Modeling thematic fit (a verb--argument compositional semantics task) currently requires a very large burden of data. We take a high-performing neural approach to modeling verb--argument fit, previously trained on a linguistically machine-annotated large corpus, and replace corpus layers with output from higher-quality taggers. Contrary to popular beliefs that, in the deep learning era, more data is as effective as higher quality annotation, we discover that higher annotation quality dramatically reduces our data requirement while demonstrating better supervised predicate-argument classification. But in applying the model to a psycholinguistic task outside the training objective, we saw only small gains in one of two thematic fit estimation tasks, and none in the other. We replicate previous studies while modifying certain role representation details, and set a new state-of-the-art in event modeling, using a fraction of the data.
更多查看译文
关键词
participant representation,annotation quality,event
AI 理解论文
溯源树
样例
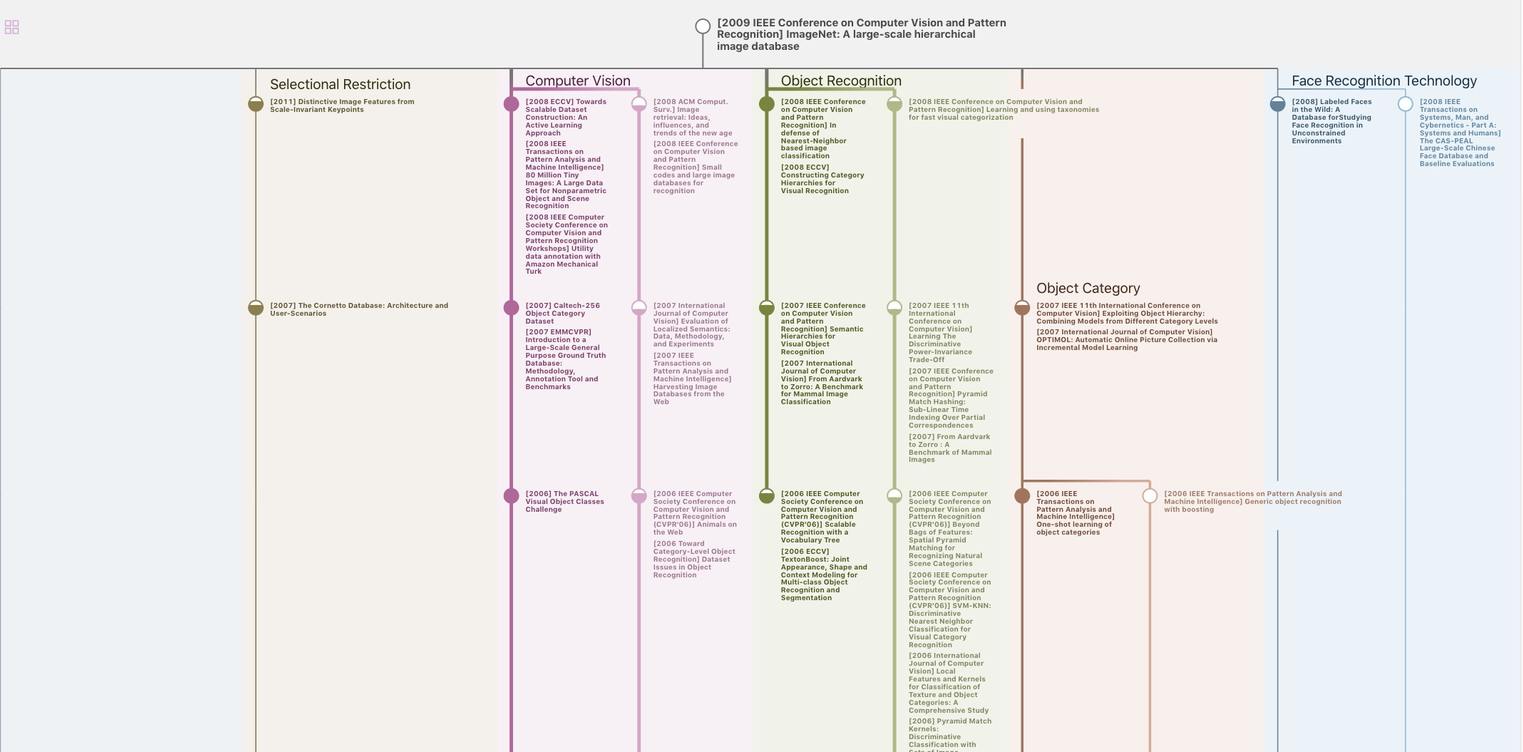
生成溯源树,研究论文发展脉络
Chat Paper
正在生成论文摘要