Adaptive Test-Time Augmentation for Low-Power CPU
CoRR(2021)
摘要
Convolutional Neural Networks (ConvNets) are trained offline using the few available data and may therefore suffer from substantial accuracy loss when ported on the field, where unseen input patterns received under unpredictable external conditions can mislead the model. Test-Time Augmentation (TTA) techniques aim to alleviate such common side effect at inference-time, first running multiple feed-forward passes on a set of altered versions of the same input sample, and then computing the main outcome through a consensus of the aggregated predictions. Unfortunately, the implementation of TTA on embedded CPUs introduces latency penalties that limit its adoption on edge applications. To tackle this issue, we propose AdapTTA, an adaptive implementation of TTA that controls the number of feed-forward passes dynamically, depending on the complexity of the input. Experimental results on state-of-the-art ConvNets for image classification deployed on a commercial ARM Cortex-A CPU demonstrate AdapTTA reaches remarkable latency savings, from 1.49X to 2.21X, and hence a higher frame rate compared to static TTA, still preserving the same accuracy gain.
更多查看译文
关键词
cpu,test-time,low-power
AI 理解论文
溯源树
样例
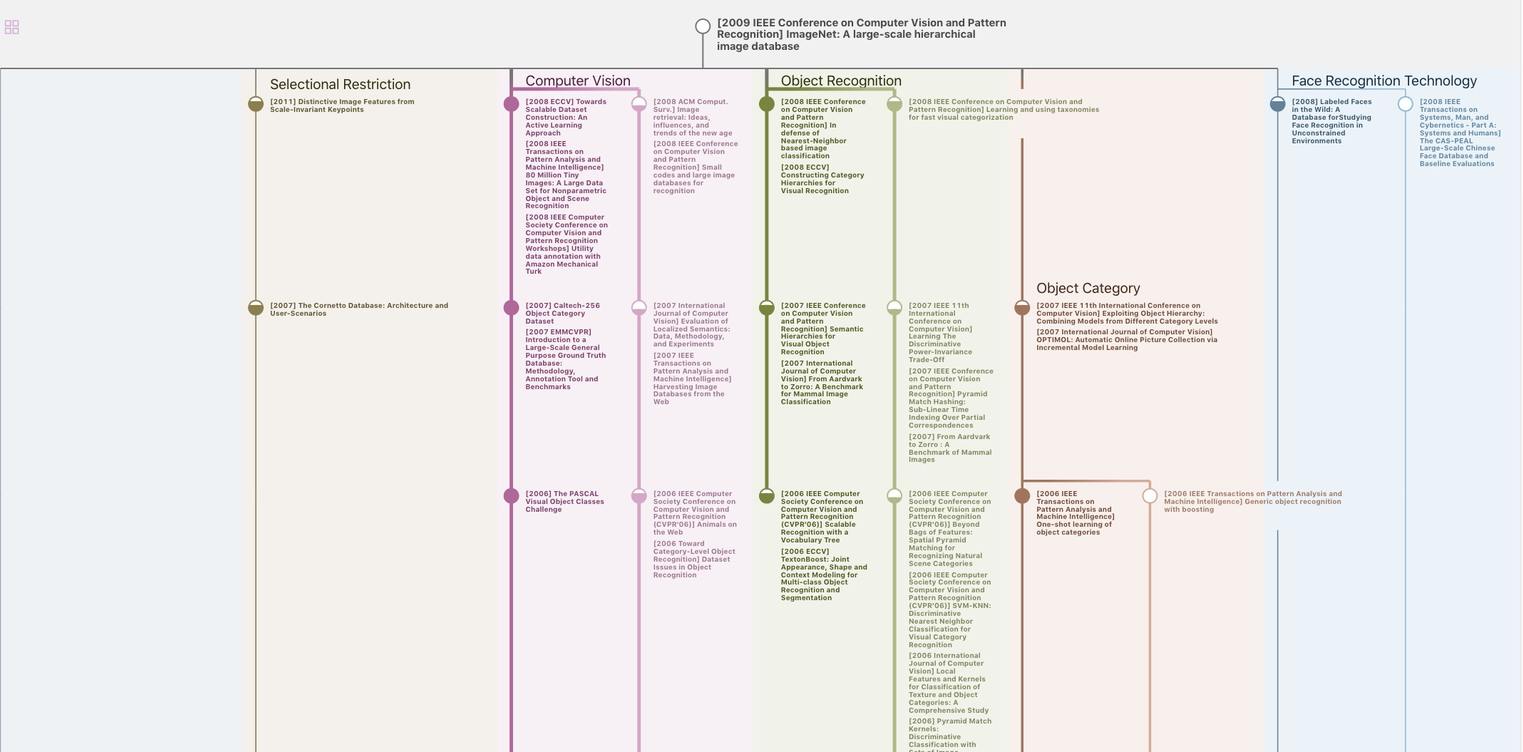
生成溯源树,研究论文发展脉络
Chat Paper
正在生成论文摘要