Uncertainty-aware Safe Exploratory Planning using Gaussian Process and Neural Control Contraction Metric
L4DC(2021)
摘要
In this paper, we consider the problem of using a robot to explore an environment with an unknown, state-dependent disturbance function while avoiding some forbidden areas. The goal of the robot is to safely collect observations of the disturbance and construct an accurate estimate of the underlying disturbance function. We use Gaussian Process (GP) to get an estimate of the disturbance from data with a high-confidence bound on the regression error. Furthermore, we use neural Contraction Metrics to derive a tracking controller and the corresponding high-confidence uncertainty tube around the nominal trajectory planned for the robot, based on the estimate of the disturbance. From the robustness of the Contraction Metric, error bound can be pre-computed and used by the motion planner such that the actual trajectory is guaranteed to be safe. As the robot collects more and more observations along its trajectory, the estimate of the disturbance becomes more and more accurate, which in turn improves the performance of the tracking controller and enlarges the free space that the robot can safely explore. We evaluate the proposed method using a carefully designed environment with a ground vehicle. Results show that with the proposed method the robot can thoroughly explore the environment safely and quickly.
更多查看译文
关键词
planning,neural control contraction metric,gaussian process,uncertain-aware
AI 理解论文
溯源树
样例
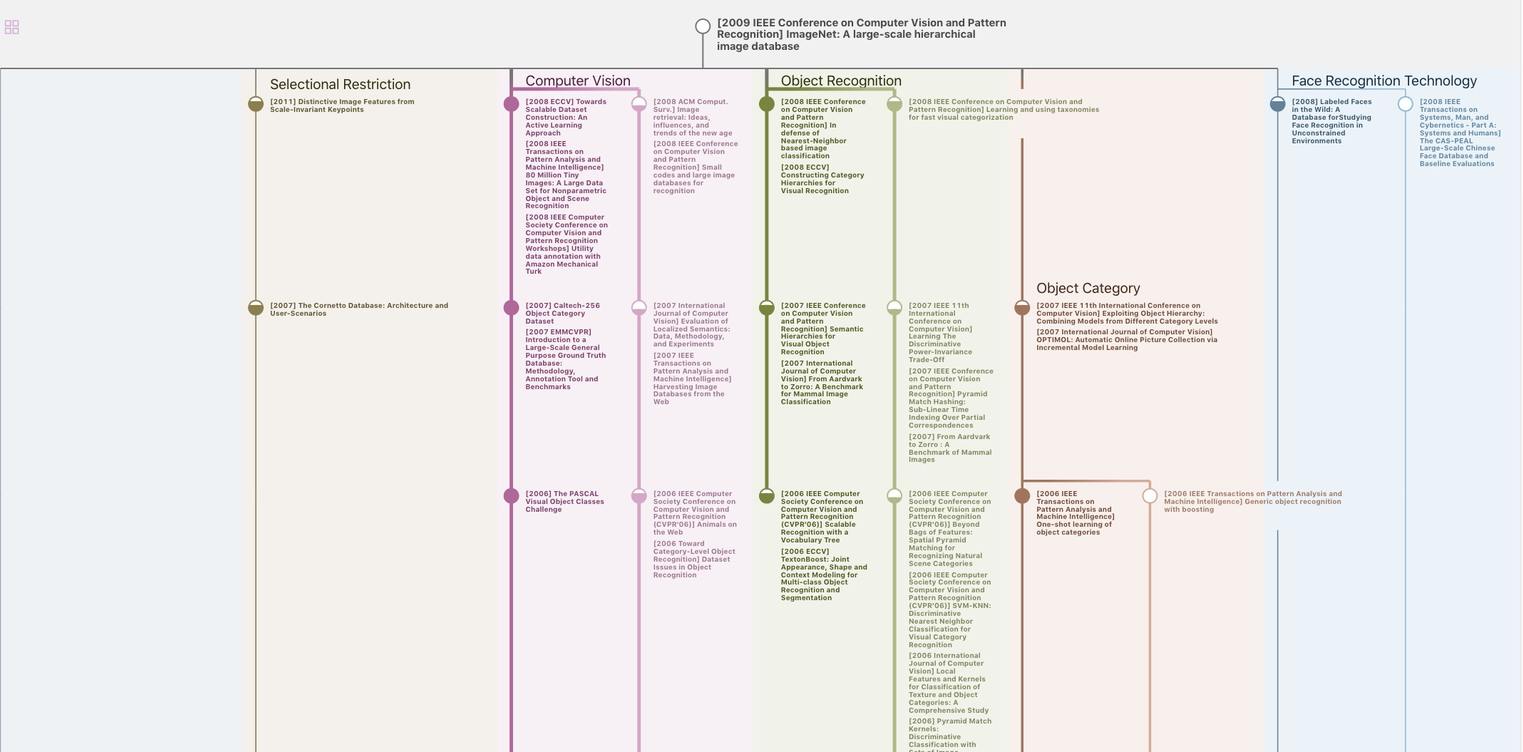
生成溯源树,研究论文发展脉络
Chat Paper
正在生成论文摘要