Streaming Transformer for Hardware Efficient Voice Trigger Detection and False Trigger Mitigation.
Interspeech(2021)
摘要
We present a unified and hardware efficient architecture for two stage voice trigger detection (VTD) and false trigger mitigation (FTM) tasks. Two stage VTD systems of voice assistants can get falsely activated to audio segments acoustically similar to the trigger phrase of interest. FTM systems cancel such activations by using post trigger audio context. Traditional FTM systems rely on automatic speech recognition lattices which are computationally expensive to obtain on device. We propose a streaming transformer (TF) encoder architecture, which progressively processes incoming audio chunks and maintains audio context to perform both VTD and FTM tasks using only acoustic features. The proposed joint model yields an average 18% relative reduction in false reject rate (FRR) for the VTD task at a given false alarm rate. Moreover, our model suppresses 95% of the false triggers with an additional one second of post-trigger audio. Finally, on-device measurements show 32% reduction in runtime memory and 56% reduction in inference time compared to non-streaming version of the model.
更多查看译文
关键词
Keyword Spotting,Speech Recognition,Acoustic Modeling,Neural Networks,Deep Learning,False Trigger Mitigation
AI 理解论文
溯源树
样例
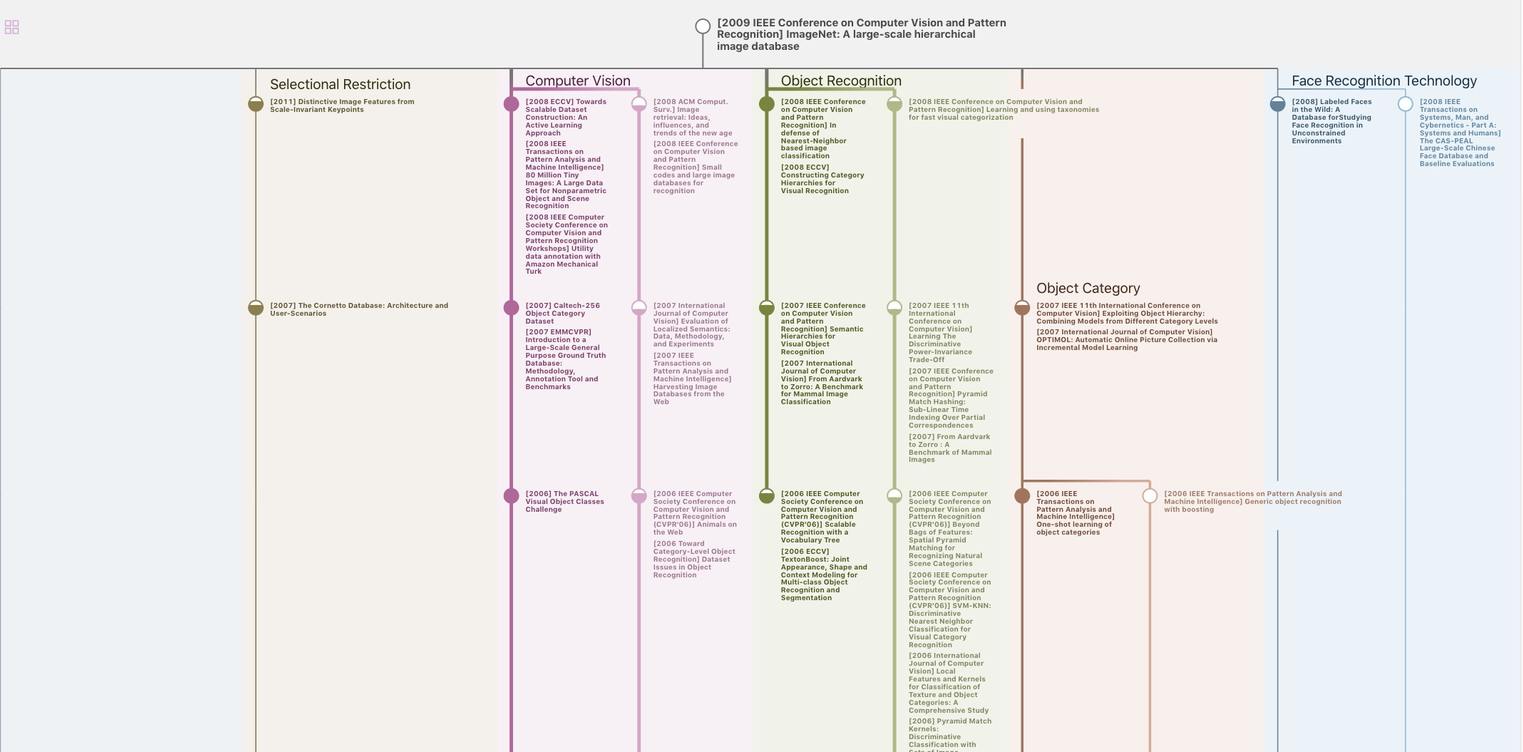
生成溯源树,研究论文发展脉络
Chat Paper
正在生成论文摘要