Combinatorial Data Augmentation: A Key Enabler to Bridge Geometry- and Data-Driven WiFi Positioning
arxiv(2023)
摘要
Due to the emergence of various wireless sensing technologies, numerous positioning algorithms have been introduced in the literature, categorized into \emph{geometry-driven positioning} (GP) and \emph{data-driven positioning} (DP). These approaches have respective limitations, e.g., a non-line-of-sight issue for GP and the lack of a labeled dataset for DP, which can be complemented by integrating both methods. To this end, this paper aims to introduce a novel principle called \emph{combinatorial data augmentation} (CDA), a catalyst for the two approaches' seamless integration. Specifically, GP-based datasets augmented from different combinations of positioning entities, called \emph{preliminary estimate locations} (PELs), can be used as DP's inputs. We confirm the CDA's effectiveness from field experiments based on WiFi \emph{round-trip times} (RTTs) and \emph{inertial measurement units} (IMUs) by designing several CDA-based positioning algorithms. First, we show that CDA offers various metrics quantifying each PEL's reliability, thereby filtering out unreliable PELs for WiFi RTT positioning. Second, CDA helps compute the measurement covariance matrix of a Kalman filter for fusing two position estimates derived by WiFi RTT and IMUs. Third, we use the above position estimate as the corresponding PEL's real-time label for fingerprint-based positioning as a representative DP algorithm. It provides accurate and reliable positioning results, says an average positioning error of $1.51$ (m) with a standard deviation of $0.88$~(m).
更多查看译文
关键词
combinatorial data augmentation,data-driven
AI 理解论文
溯源树
样例
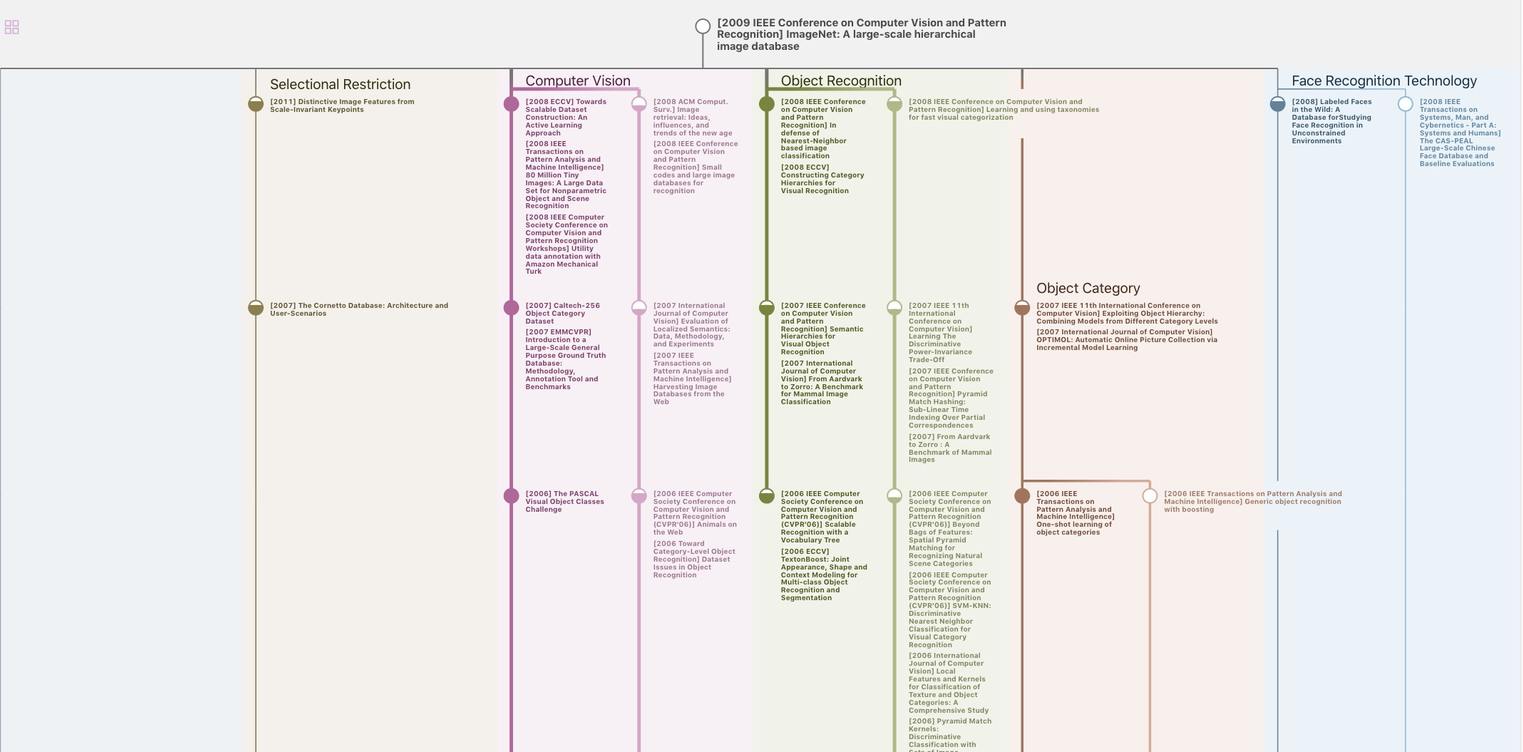
生成溯源树,研究论文发展脉络
Chat Paper
正在生成论文摘要