Deep regression for uncertainty-aware and interpretable analysis of large-scale body MRI
arxiv(2021)
摘要
Large-scale medical studies such as the UK Biobank examine thousands of volunteer participants with medical imaging techniques. Combined with the vast amount of collected metadata, anatomical information from these images has the potential for medical analyses at unprecedented scale. However, their evaluation often requires manual input and long processing times, limiting the amount of reference values for biomarkers and other measurements available for research. Recent approaches with convolutional neural networks for regression can perform these evaluations automatically. On magnetic resonance imaging (MRI) data of more than 40,000 UK Biobank subjects, these systems can estimate human age, body composition and more. This style of analysis is almost entirely data-driven and no manual intervention or guidance with manually segmented ground truth images is required. The networks often closely emulate the reference method that provided their training data and can reach levels of agreement comparable to the expected variability between established medical gold standard techniques. The risk of silent failure can be individually quantified by predictive uncertainty obtained from a mean-variance criterion and ensembling. Saliency analysis furthermore enables an interpretation of the underlying relevant image features and showed that the networks learned to correctly target specific organs, limbs, and regions of interest.
更多查看译文
关键词
deep regression,mri,uncertainty-aware,large-scale
AI 理解论文
溯源树
样例
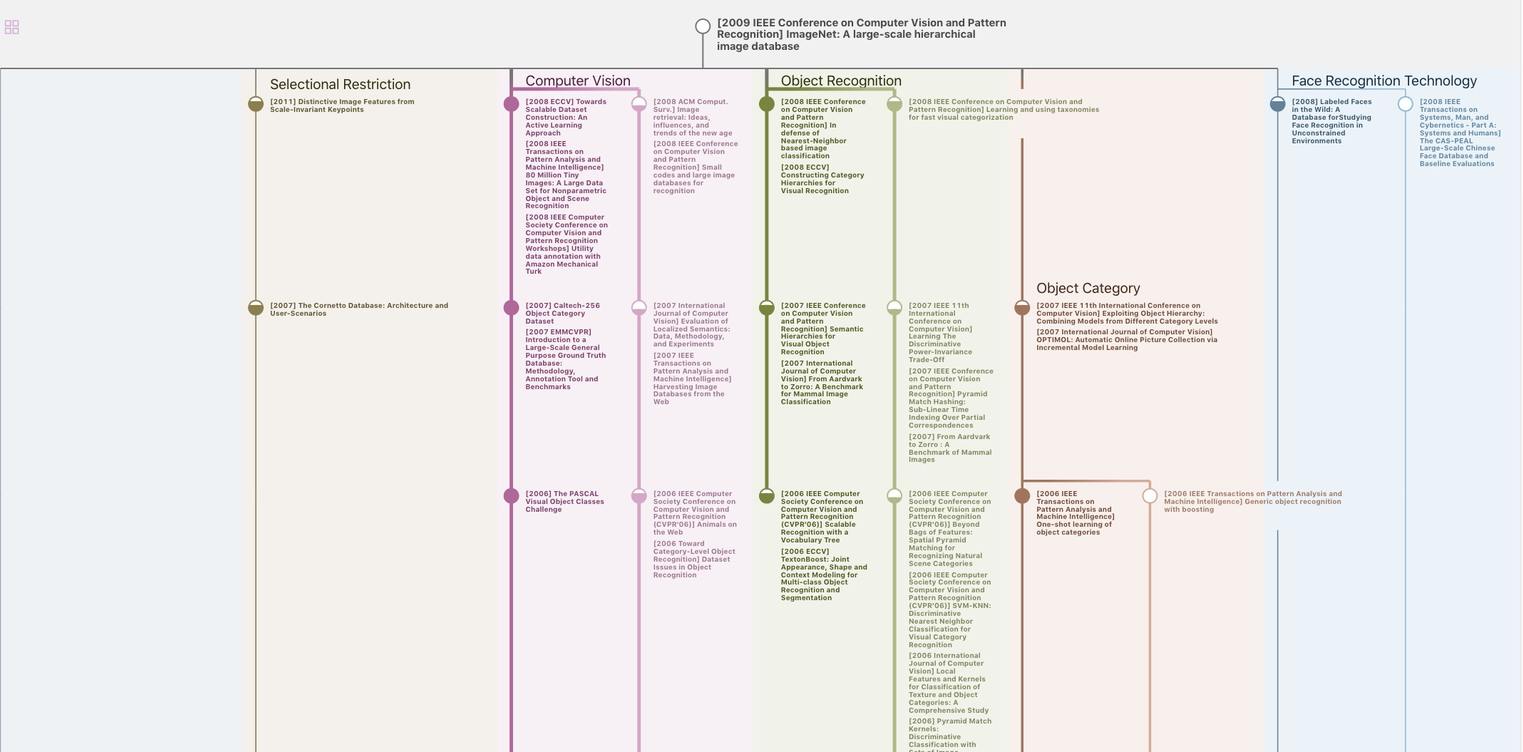
生成溯源树,研究论文发展脉络
Chat Paper
正在生成论文摘要