Predicting LncRNA-Disease Association Based on Generative Adversarial Network.
CURRENT GENE THERAPY(2022)
摘要
BACKGROUND:Increasing research reveals that long non-coding RNAs (lncRNAs) play an important role in various biological processes of human diseases. Nonetheless, only a handful of lncRNA-disease associations have been experimentally verified. The study of lncRNA-disease association prediction based on the computational model has provided a preliminary basis for biological experiments to a great degree so as to cut down the huge cost of wet lab experiments.OBJECTIVE:This study aims to learn the real distribution of lncRNA-disease association from a limited number of known lncRNA-disease association data. This paper proposes a new lncRNA-disease association prediction model called LDA-GAN based on a Generative Adversarial Network (GAN).METHODS:Aiming at the problems of slow convergence rate, training instabilities, and unavailability of discrete data in traditional GAN, LDA-GAN utilizes the Gumbel-softmax technology to construct a differentiable process for simulating discrete sampling. Meanwhile, the generator and the discriminator of LDA-GAN are integrated to establish the overall optimization goal based on the pairwise loss function.RESULTS:Experiments on standard datasets demonstrate that LDA-GAN achieves not only high stability and high efficiency in the process of confrontation learning but also gives full play to the semisupervised learning advantage of generative adversarial learning framework for unlabeled data, which further improves the prediction accuracy of lncRNA-disease association. Besides, case studies show that LDA-GAN can accurately generate potential diseases for several lncRNAs.CONCLUSION:We introduce a generative adversarial model to identify lncRNA-disease associations.
更多查看译文
关键词
Competing Endogenous RNA,Long Noncoding RNAs
AI 理解论文
溯源树
样例
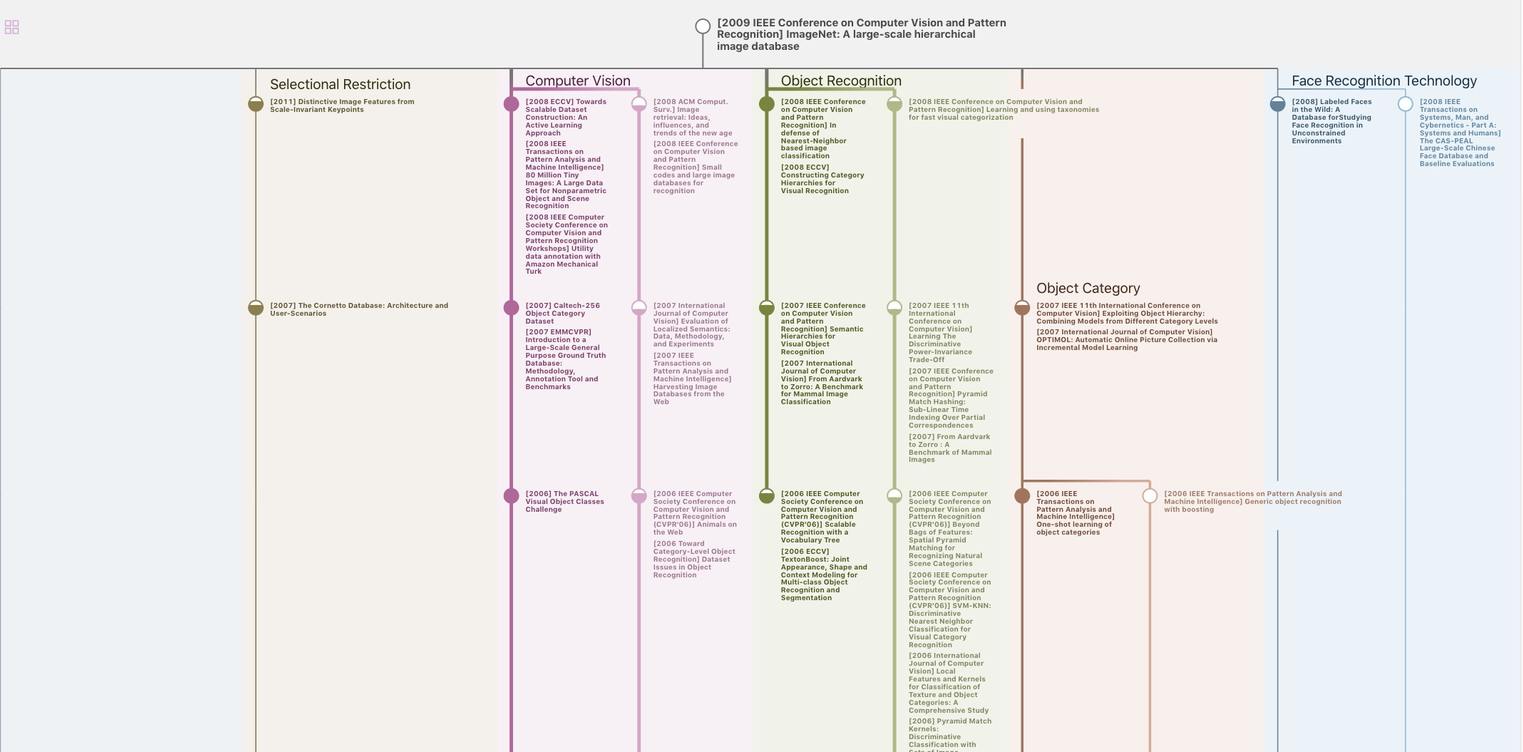
生成溯源树,研究论文发展脉络
Chat Paper
正在生成论文摘要