Generalized Few-Shot Object Detection without Forgetting
2021 IEEE/CVF CONFERENCE ON COMPUTER VISION AND PATTERN RECOGNITION, CVPR 2021(2021)
摘要
Recently few-shot object detection is widely adopted to deal with data-limited situations. While most previous works merely focus on the performance on few-shot categories, we claim that detecting all classes is crucial as test samples may contain any instances in realistic applications, which requires the few-shot detector to learn new concepts without forgetting. Through analysis on transfer learning based methods, some neglected but beneficial properties are utilized to design a simple yet effective few-shot detector, Retentive R-CNN. It consists of Bias-Balanced RPN to debias the pretrained RPN and Re-detector to find few-shot class objects without forgetting previous knowledge. Extensive experiments on few-shot detection benchmarks show that Retentive R-CNN significantly outperforms state-of-the-art methods on overall performance among all settings as it can achieve competitive results on few-shot classes and does not degrade the base class performance at all. Our approach has demonstrated that the long desired never-forgetting learner is available in object detection.
更多查看译文
关键词
object detection,few-shot
AI 理解论文
溯源树
样例
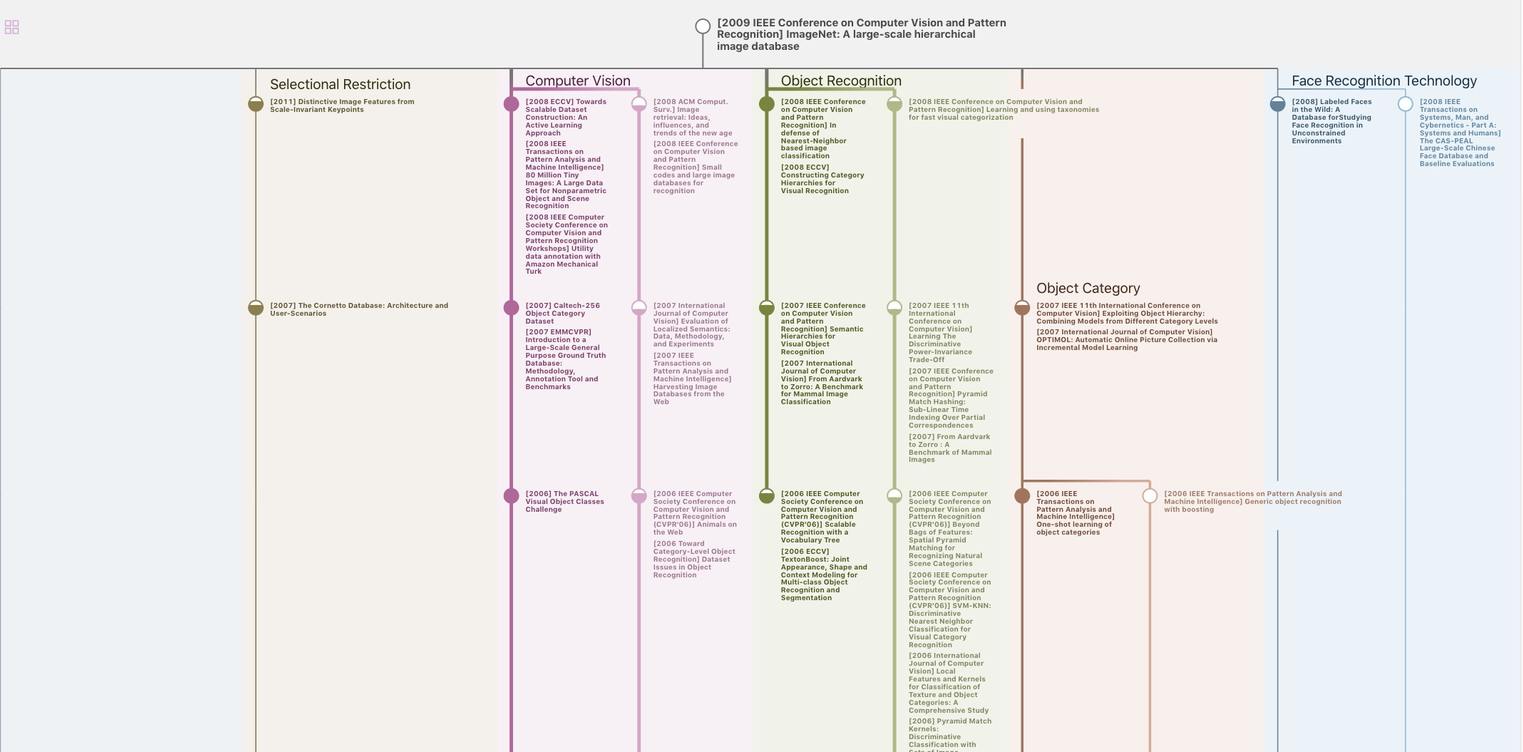
生成溯源树,研究论文发展脉络
Chat Paper
正在生成论文摘要