Personalized prediction of transcranial magnetic stimulation clinical response in patients with treatment-refractory depression using neuroimaging biomarkers and machine learning.
Journal of affective disorders(2021)
摘要
BACKGROUND:Functional connectivity between the left dorsolateral prefrontal cortex (DLPFC) and subgenual cingulate (sgACC) may serve as a biomarker for transcranial magnetic stimulation (rTMS) treatment response. The first aim was to establish whether this finding is veridical or artifactually induced by the pre-processing method. Furthermore, alternative biomarkers were identified and the clinical utility for personalized medicine was examined.
METHODS:Resting-state fMRI data were collected in medication-refractory depressed patients (n = 70, 16 males) before undergoing neuronavigated left DLPFC rTMS. Seed-based analyses were performed with and without global signal regression pre-processing to identify biomarkers of short-term and long-term treatment response. Receiver Operating Characteristic curve and supervised machine learning analyses were applied to assess the clinical utility of these biomarkers for the classification of categorical rTMS response.
RESULTS:Regardless of the pre-processing method, DLPFC-sgACC connectivity was not associated with treatment outcome. Instead, poorer connectivity between the sgACC and three clusters (peak locations: frontal pole, superior parietal lobule, occipital cortex) and DLPFC-central opercular cortex were observed in long-term nonresponders. The identified connections could serve as acceptable to excellent markers. Combining the features using supervised machine learning reached accuracy rates of 95.35% (CI=82.94-100.00) and 88.89% (CI=63.96-100.00) in the cross-validation and test dataset, respectively.
LIMITATIONS:The sample size was moderate, and features for machine learning were based on group differences.
CONCLUSIONS:Long-term nonresponders showed greater disrupted connectivity in regions involving the central executive network. Our findings may aid the development of personalized medicine for medication-refractory depression.
更多查看译文
AI 理解论文
溯源树
样例
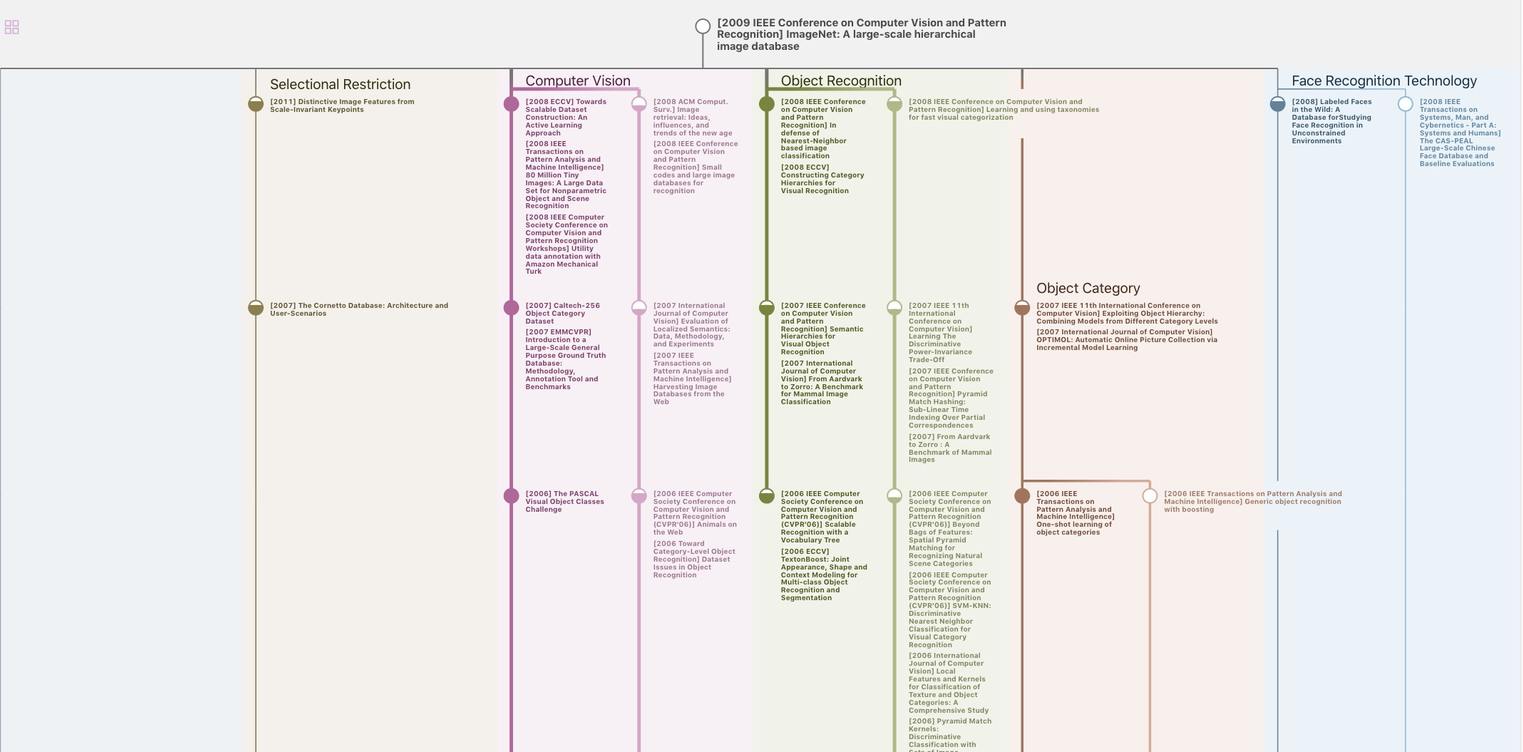
生成溯源树,研究论文发展脉络
Chat Paper
正在生成论文摘要