Gradient Estimation Of Information Measures In Deep Learning
KNOWLEDGE-BASED SYSTEMS(2021)
摘要
Information measures including entropy and mutual information (MI) have been widely applied in deep learning. Despite the successes, exiting estimation methods suffer from either high variance or high bias. This may lead to unstable training or poor performance in deep learning. Since estimating information measures in themselves is very difficult, we explore an alternative appealing strategy, by directly estimating the gradients of information measures with respect to model parameters. We propose a general gradient estimation method for information measures based on the score estimation. In detail, we establish the Entropy Gradient Estimator (EGE) and the Mutual Information Gradient Estimator (MIGE) to estimate the gradient of entropy and mutual information with respect to model parameters, respectively. For dealing with the optimization of entropy and mutual information, we can directly plug in their gradient approximation with relevant parameters to enable stochastic backpropagation for stability and efficiency. Our proposed method exhibits higher accuracy and lower variance for gradient estimation of information measures. Extensive experiments on various deep learning tasks have demonstrated the superiority of our method. (C) 2021 Elsevier B.V. All rights reserved.
更多查看译文
关键词
Entropy, Mutual information, Score estimation, Gradient estimation
AI 理解论文
溯源树
样例
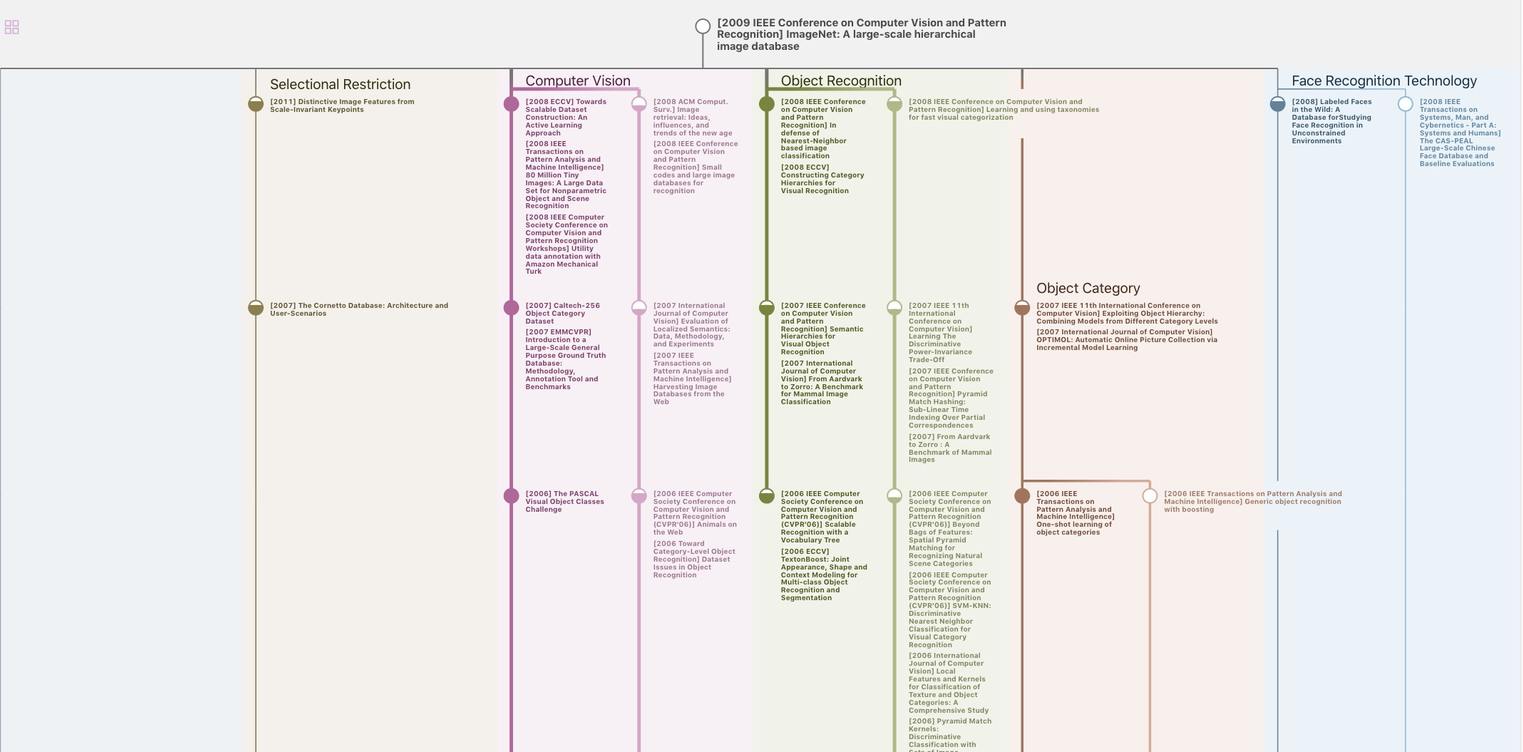
生成溯源树,研究论文发展脉络
Chat Paper
正在生成论文摘要