A novel method to correct repolarization time estimation from unipolar electrograms distorted by standard filtering.
Medical image analysis(2021)
摘要
Reliable patient-specific ventricular repolarization times (RTs) can identify regions of functional block or afterdepolarizations, indicating arrhythmogenic cardiac tissue and the risk of sudden cardiac death. Unipolar electrograms (UEs) record electric potentials, and the Wyatt method has been shown to be accurate for estimating RT from a UE. High-pass filtering is an important step in processing UEs, however, it is known to distort the T-wave phase of the UE, which may compromise the accuracy of the Wyatt method. The aim of this study was to examine the effects of high-pass filtering, and improve RT estimates derived from filtered UEs. We first generated a comprehensive set of UEs, corresponding to early and late activation and repolarization, that were then high-pass filtered with settings that mimicked the CARTO filter. We trained a deep neural network (DNN) to output a probabilistic estimation of RT and a measure of confidence, using the filtered synthetic UEs and their true RTs. Unfiltered ex-vivo human UEs were also filtered and the trained DNN used to estimate RT. Even a modest 2 Hz high-pass filter imposes a significant error on RT estimation using the Wyatt method. The DNN outperformed the Wyatt method in 62.75% of cases, and produced a significantly lower absolute error (p=8.99E-13), with a median of 16.91 ms, on 102 ex-vivo UEs. We also applied the DNN to patient UEs from CARTO, from which an RT map was computed. In conclusion, DNNs trained on synthetic UEs improve the RT estimation from filtered UEs, which leads to more reliable repolarization maps that help to identify patient-specific repolarization abnormalities.
更多查看译文
AI 理解论文
溯源树
样例
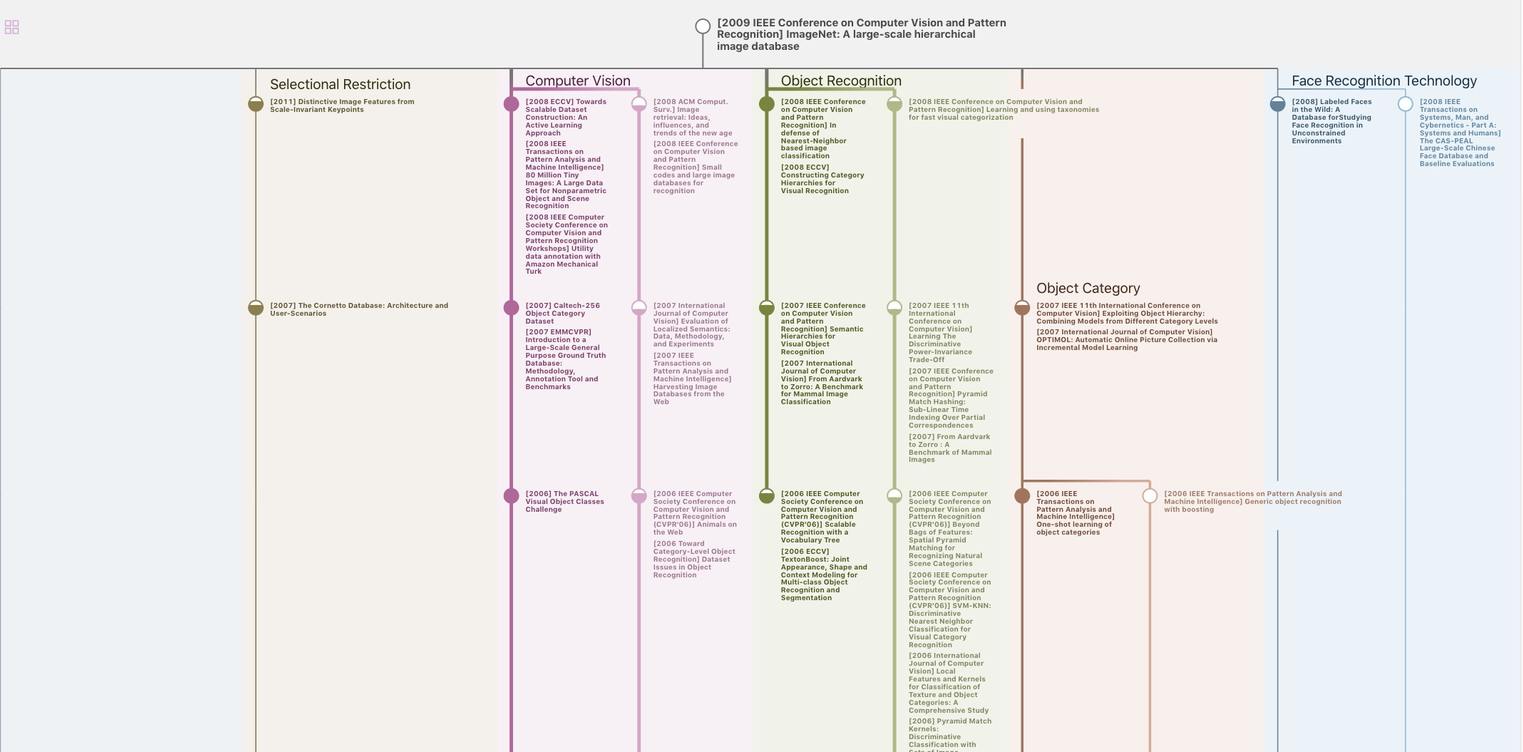
生成溯源树,研究论文发展脉络
Chat Paper
正在生成论文摘要