Spatial-Temporal Conv-Sequence Learning With Accident Encoding for Traffic Flow Prediction
IEEE Transactions on Network Science and Engineering(2022)
摘要
In an intelligent transportation system, the key problem of traffic forecasting is how to extract periodic temporal dependencies and complex spatial correlations. Current state-of-the-art methods for predicting traffic flow are based on graph architectures and sequence learning models, but they do not fully exploit dynamic spatial-temporal information in the traffic system. Specifically, the temporal dependencies in the short-range are diluted by recurrent neural networks. Moreover, local spatial information is also ignored by existing sequence models, because their convolution operation uses global average pooling. Besides, accidents may occur during object transition, which will cause congestion in the real world and further decrease prediction accuracy. To overcome these challenges, we propose Spatial-Temporal Conv-sequence Learning (STCL), where a focused temporal block uses unidirectional convolution to capture short-term periodic temporal dependencies effectively, and a spatial-temporal fusion module is responsible for extracting dependencies of interactions and decreasing the feature dimensions. Moreover, as the accidents features have an impact on local traffic congestion, we employ position encoding to detect anomalies in complex traffic situations. We have conducted a large number of experiments on real-world tasks and verified the effectiveness of our proposed method.
更多查看译文
关键词
Sequence learning,spatial-temporal,accident,traffic prediction
AI 理解论文
溯源树
样例
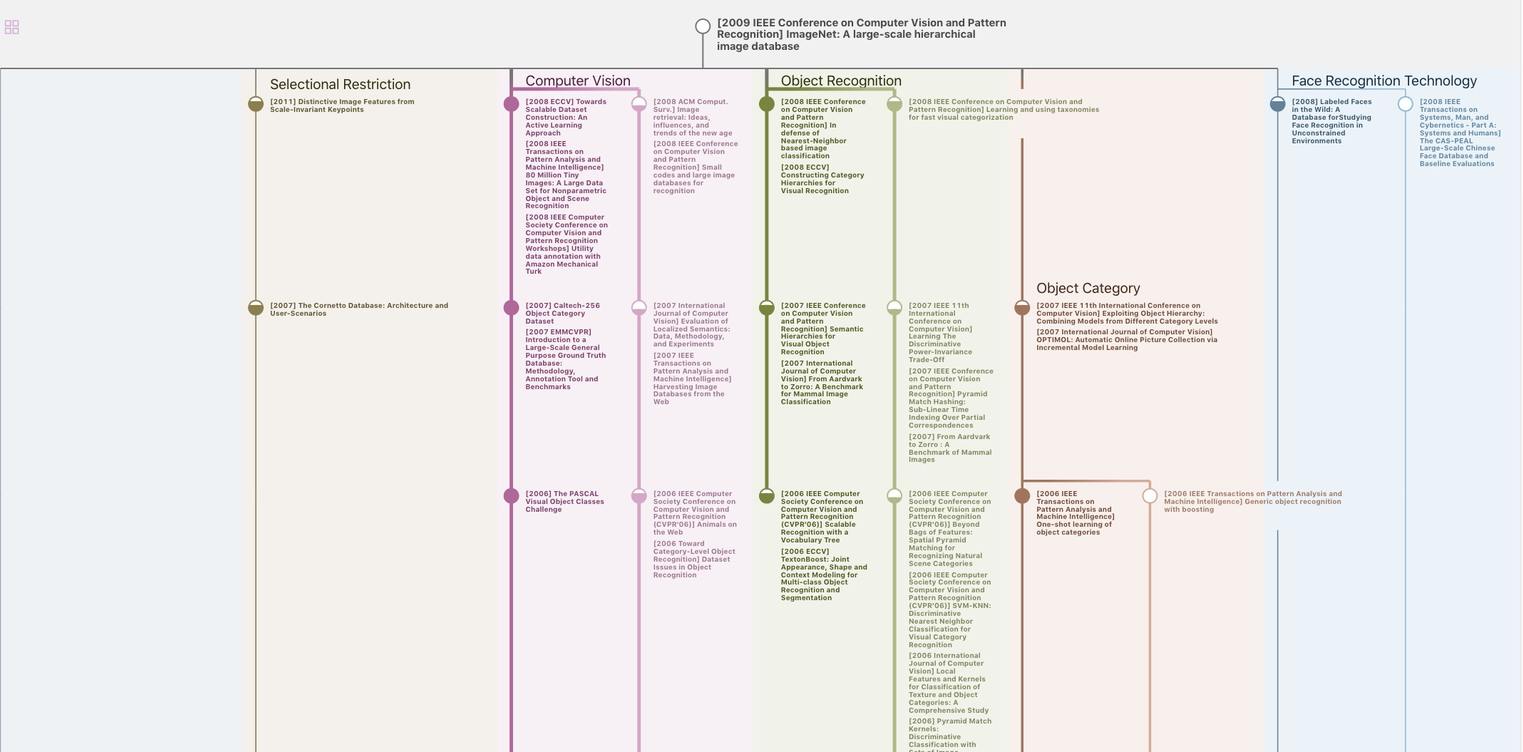
生成溯源树,研究论文发展脉络
Chat Paper
正在生成论文摘要