Uncertainty-Aware Signal Temporal Logic Inference
arxiv(2022)
摘要
Temporal logic inference is the process of extracting formal descriptions of system behaviors from data in the form of temporal logic formulas. The existing temporal logic inference methods mostly neglect uncertainties in the data, which results in the limited applicability of such methods in real-world deployments. In this paper, we first investigate the uncertainties associated with trajectories of a system and represent such uncertainties in the form of interval trajectories. We then propose two uncertainty-aware signal temporal logic (STL) inference approaches to classify the undesired behaviors and desired behaviors of a system. Instead of classifying finitely many trajectories, we classify infinitely many trajectories within the interval trajectories. In the first approach, we incorporate robust semantics of STL formulas with respect to an interval trajectory to quantify the margin at which an STL formula is satisfied or violated by the interval trajectory. The second approach relies on the first learning algorithm and exploits the decision trees to infer STL formulas to classify behaviors of a given system. The proposed approaches also work for non-separable data by optimizing the worstcase robustness margin in inferring an STL formula. Finally, we evaluate the performance of the proposed algorithms and present the obtained numerical results, where the proposed algorithms show reduction in the computation time by up to the factor of 95 on average, while the worstcase robustness margins are improved by up to 330% in comparison with the sampling-based baseline algorithms.
更多查看译文
关键词
Temporal logic inference, Uncertainties, Decision trees
AI 理解论文
溯源树
样例
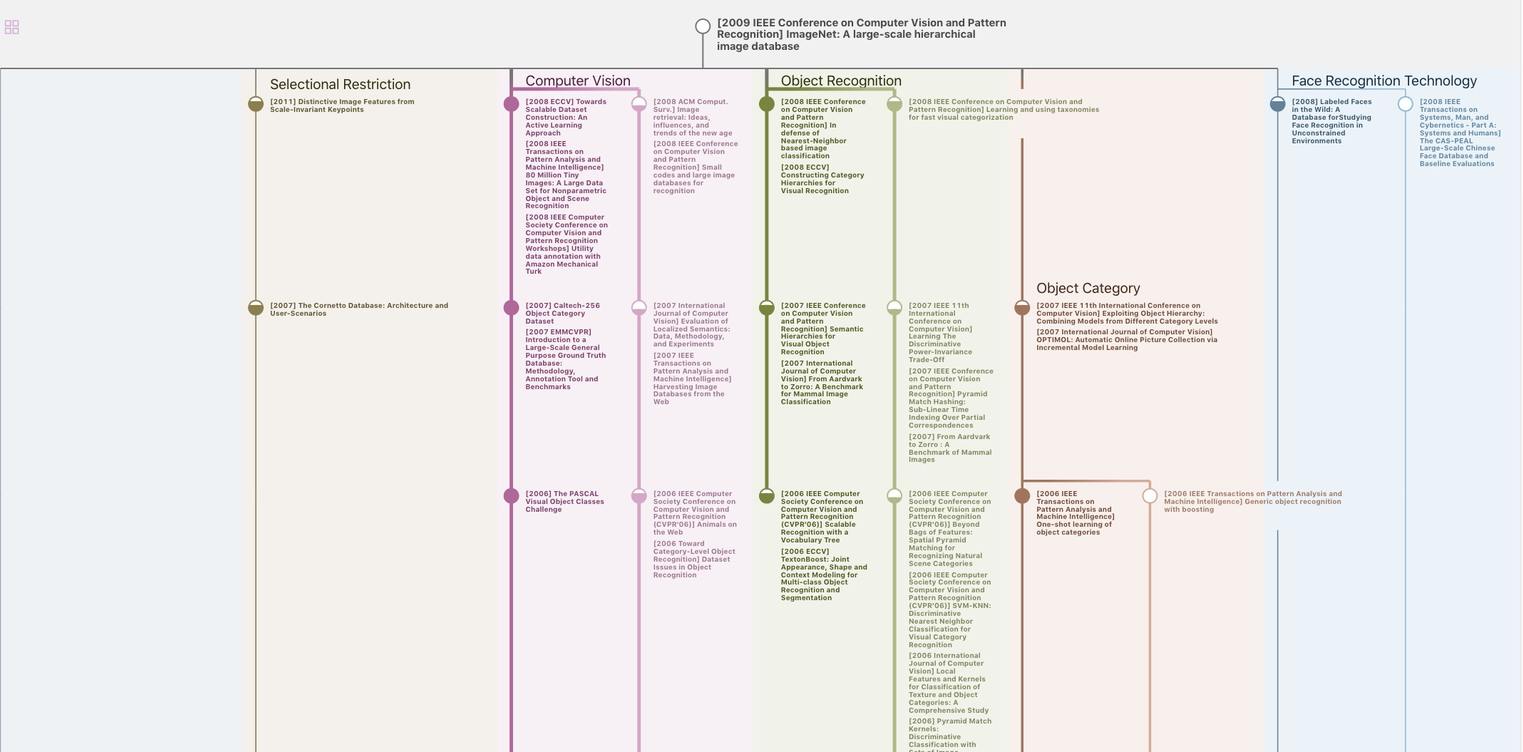
生成溯源树,研究论文发展脉络
Chat Paper
正在生成论文摘要