TRACE: A Differentiable Approach to Line-Level Stroke Recovery for Offline Handwritten Text
DOCUMENT ANALYSIS AND RECOGNITION, ICDAR 2021, PT III(2021)
摘要
Stroke order and velocity are helpful features in the fields of signature verification, handwriting recognition, and handwriting synthesis. Recovering these features from offline handwritten text is a challenging and well-studied problem. We propose a new model called TRACE (Trajectory Recovery by an Adaptively-trained Convolutional Encoder). TRACE is a differentiable approach that uses a convolutional recurrent neural network (CRNN) to infer temporal stroke information from long lines of offline handwritten text with many characters and dynamic time warping (DTW) to align predictions and ground truth points. TRACE is perhaps the first system to be trained end-to-end on entire lines of text of arbitrary width and does not require the use of dynamic exemplars. Moreover, the system does not require images to undergo any preprocessing, nor do the predictions require any post-processing. Consequently, the recovered trajectory is differentiable and can be used as a loss function for other tasks, including synthesizing offline handwritten text. We demonstrate that temporal stroke information recovered by TRACE from offline data can be used for handwriting synthesis and establish the first benchmarks for a stroke trajectory recovery system trained on the IAM online handwriting dataset.
更多查看译文
关键词
Handwriting, Stroke recovery, Deep learning
AI 理解论文
溯源树
样例
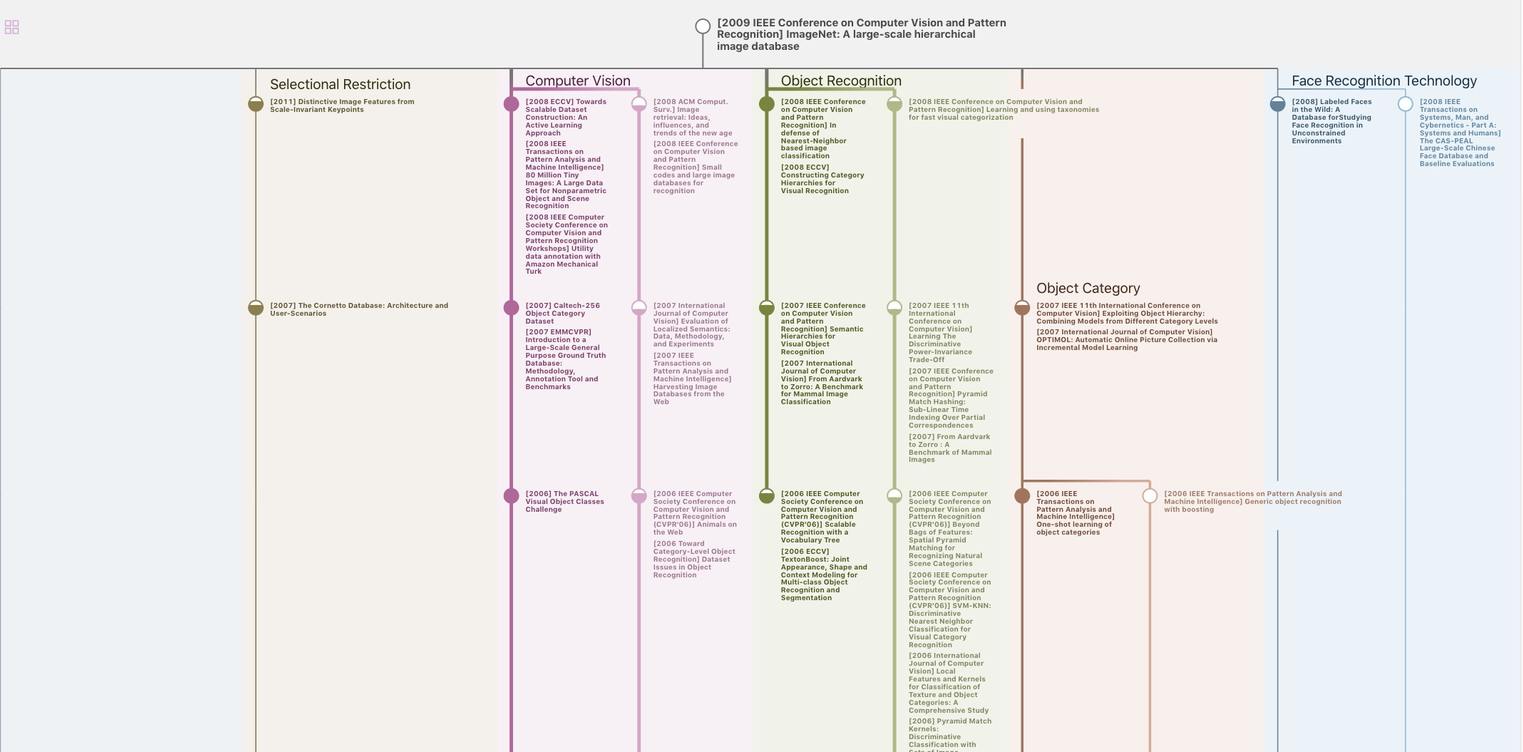
生成溯源树,研究论文发展脉络
Chat Paper
正在生成论文摘要