Dynamic Probabilistic Pruning: A General Framework for Hardware-Constrained Pruning at Different Granularities
IEEE TRANSACTIONS ON NEURAL NETWORKS AND LEARNING SYSTEMS(2024)
摘要
Unstructured neural network pruning algorithms have achieved impressive compression ratios. However, the resulting-typically irregular-sparse matrices hamper efficient hardware implementations, leading to additional memory usage and complex control logic that diminishes the benefits of unstructured pruning. This has spurred structured coarse-grained pruning solutions that prune entire feature maps or even layers, enabling efficient implementation at the expense of reduced flexibility. Here, we propose a flexible new pruning mechanism that facilitates pruning at different granularities (weights, kernels, and feature maps) while retaining efficient memory organization (e.g., pruning exactly k-out-of- n weights for every output neuron or pruning exactly k-out-of-n kernels for every feature map). We refer to this algorithm as dynamic probabilistic pruning (DPP). DPP leverages the Gumbel-softmax relaxation for differentiable k-out-of-n sampling, facilitating end-to-end optimization. We show that DPP achieves competitive compression ratios and classification accuracy when pruning common deep learning models trained on different benchmark datasets for image classification. Relevantly, the dynamic masking of DPP facilitates for joint optimization of pruning and weight quantization in order to even further compress the network, which we show as well. Finally, we propose novel information-theoretic metrics that show the confidence and pruning diversity of pruning masks within a layer.
更多查看译文
关键词
Deep learning (DL),hardware-oriented pruning,model compression
AI 理解论文
溯源树
样例
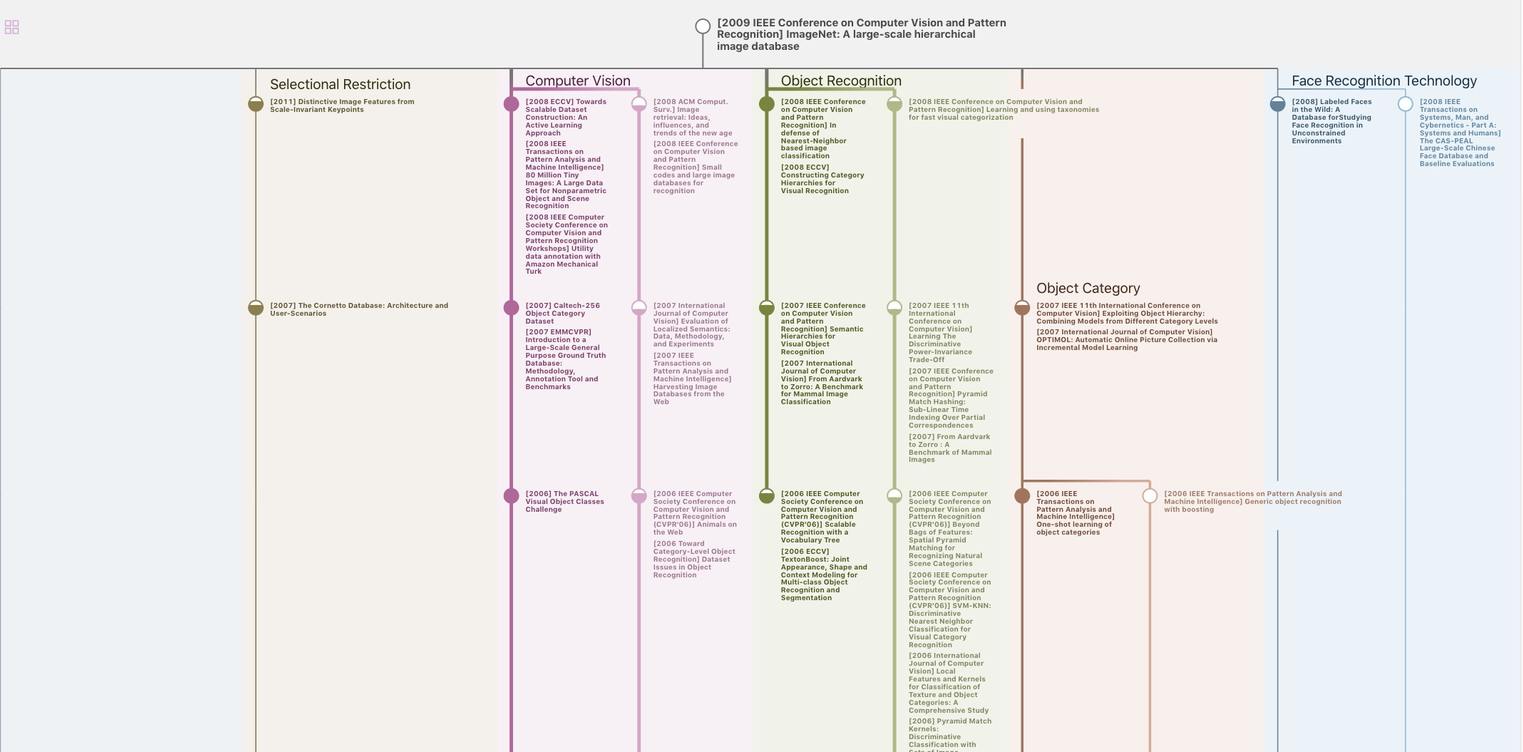
生成溯源树,研究论文发展脉络
Chat Paper
正在生成论文摘要