Multi-modal Semantic Inconsistency Detection in Social Media News Posts
MULTIMEDIA MODELING, MMM 2022, PT II(2022)
摘要
As computer-generated content and deepfakes make steady improvements, semantic approaches to multimedia forensics will become more important. In this paper, we introduce a novel classification architecture for identifying semantic inconsistencies between video appearance and text caption in social media news posts. While similar systems exist for text and images, we aim to detect inconsistencies in a more ambiguous setting, as videos can be long and contain several distinct scenes, in addition to adding audio as an extra modality. We develop a multi-modal fusion framework to identify mismatches between videos and captions in social media posts by leveraging an ensemble method based on textual analysis of the caption, automatic audio transcription, semantic video analysis, object detection, named entity consistency, and facial verification. To train and test our approach, we curate a new video-based dataset of 4,000 real-world Facebook news posts for analysis. Our multi-modal approach achieves 60.5% classification accuracy on random mismatches between caption and appearance, compared to accuracy below 50% for uni-modal models. Further ablation studies confirm the necessity of fusion across modalities for correctly identifying semantic inconsistencies.
更多查看译文
关键词
Multi-modal, Social media, Forensics, Fusion
AI 理解论文
溯源树
样例
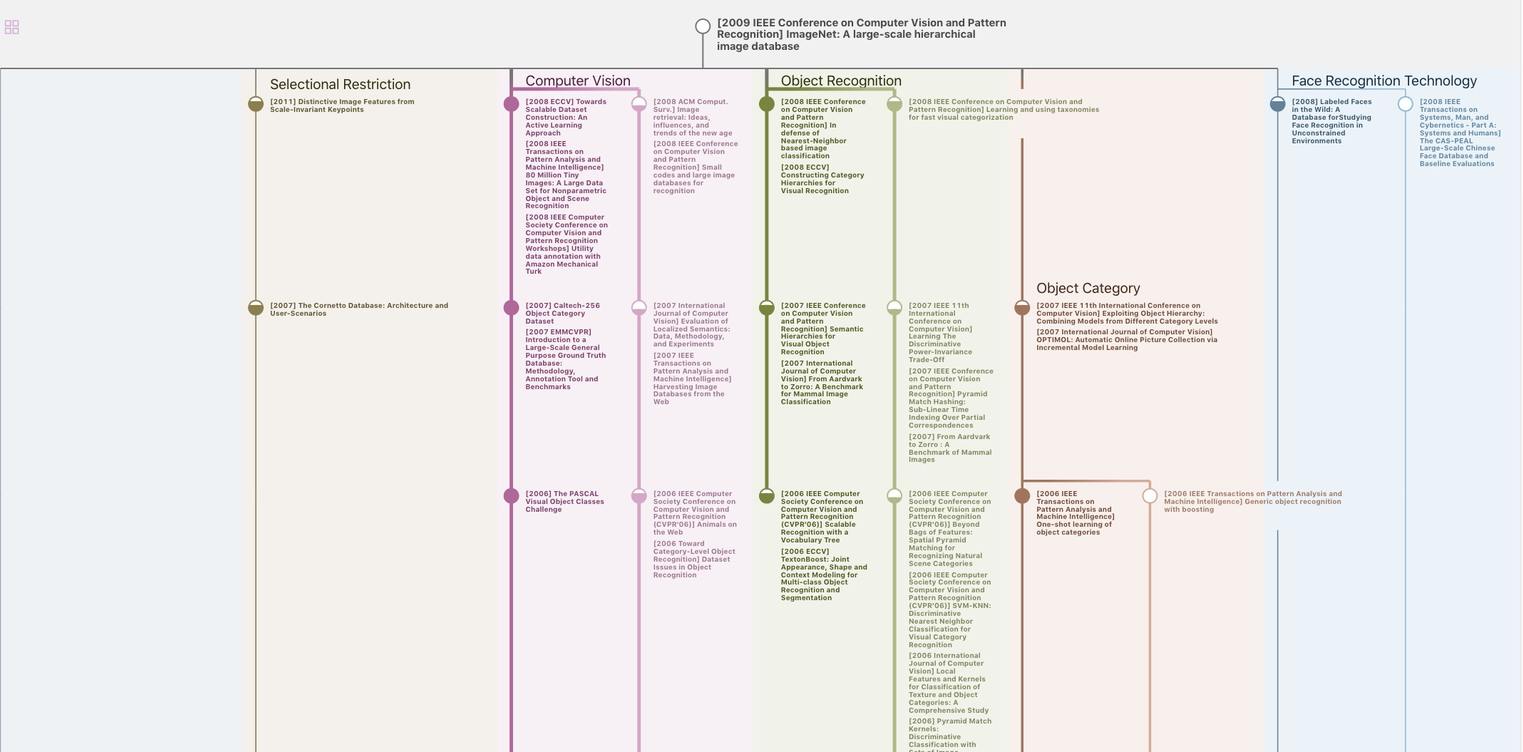
生成溯源树,研究论文发展脉络
Chat Paper
正在生成论文摘要