Accuracy of Support-Vector Machines for Diagnosis of Alzheimer's Disease, Using Volume of Brain Obtained by Structural MRI at Siriraj Hospital
FRONTIERS IN NEUROLOGY(2021)
摘要
Background: The determination of brain volumes using visual ratings is associated with an inherently low accuracy for the diagnosis of Alzheimer's disease (AD). A support-vector machine (SVM) is one of the machine learning techniques, which may be utilized as a classifier for various classification problems. This study exploratorily investigated the accuracy of SVM classification models for AD subjects using brain volume and various clinical data as features. Methods: The study was designed as a retrospective chart review. A total of 201 eligible subjects were recruited from the Memory Clinic at Siriraj Hospital, Thailand. Eighteen cases were excluded due to incomplete MRI data. Subjects were randomly assigned to a training group (AD = 46, normal = 46) and testing group (AD = 45, normal = 46) for SVM modeling and validation, respectively. The results in terms of accuracy and a receiver operating characteristic curve analysis are reported. Results: The highest accuracy for brain volumetry (62.64%) was found using the hippocampus as a single feature. A combination of clinical parameters as features provided accuracy ranging between 83 and 90%. However, a combination of brain volumetry and clinical parameters as features to the SVM models did not improve the accuracy of the result. Conclusions: In our study, the use of brain volumetry as SVM features provided low classification accuracy with the highest accuracy of 62.64% using the hippocampus volume alone. In contrast, the use of clinical parameters [Thai mental state examination score, controlled oral word association tests (animals; and letters K, S, and P), learning memory, clock-drawing test, and construction-praxis] as features for SVM models provided good accuracy between 83 and 90%.
更多查看译文
关键词
Alzheimer disease,support vector machine,machine learning,volumetric MRI,Thailand
AI 理解论文
溯源树
样例
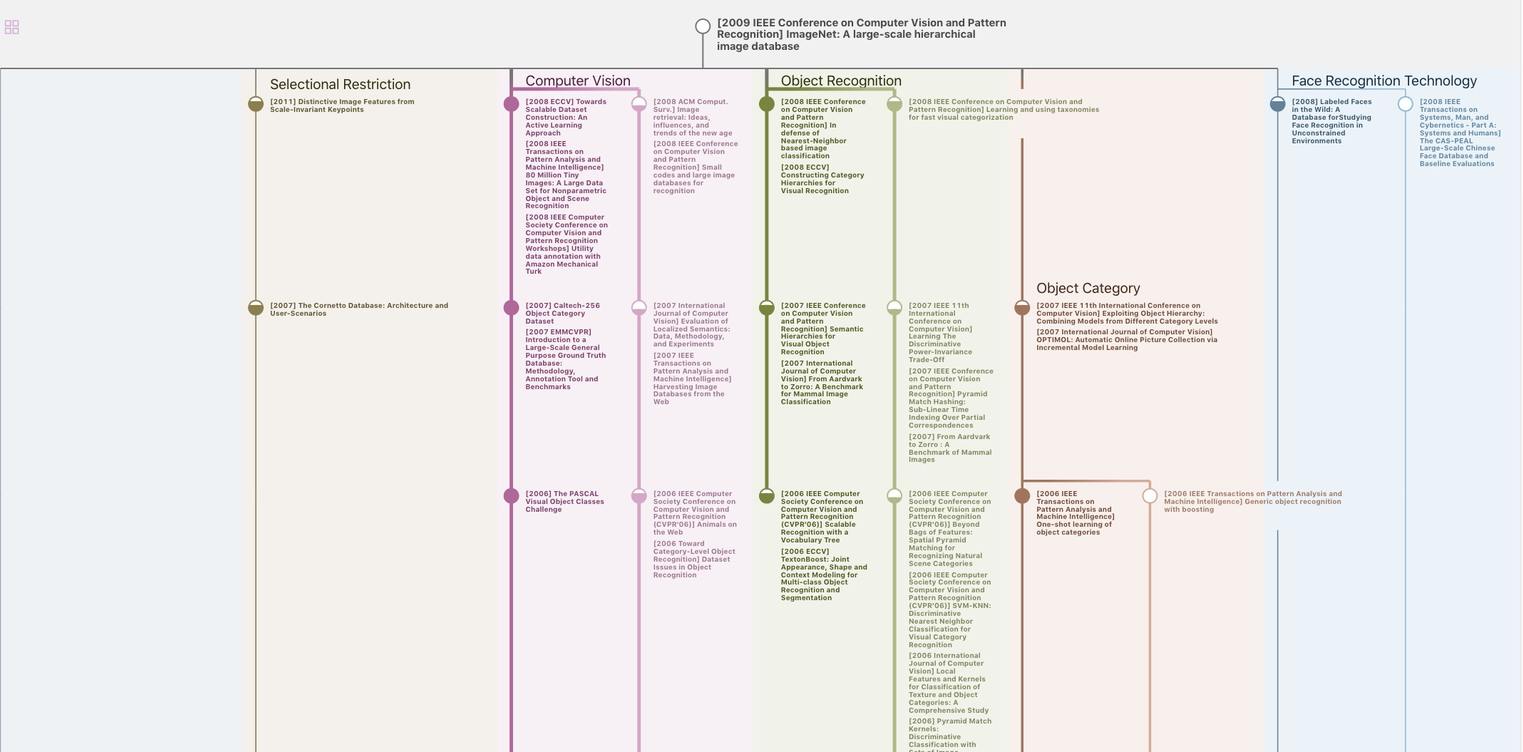
生成溯源树,研究论文发展脉络
Chat Paper
正在生成论文摘要