Relation Matters in Sampling: A Scalable Multi-Relational Graph Neural Network for Drug-Drug Interaction Prediction
CoRR(2021)
摘要
Sampling is an established technique to scale graph neural networks to large graphs. Current approaches however assume the graphs to be homogeneous in terms of relations and ignore relation types, critically important in biomedical graphs. Multi-relational graphs contain various types of relations that usually come with variable frequency and have different importance for the problem at hand. We propose an approach to modeling the importance of relation types for neighborhood sampling in graph neural networks and show that we can learn the right balance: relation-type probabilities that reflect both frequency and importance. Our experiments on drug-drug interaction prediction show that state-of-the-art graph neural networks profit from relation-dependent sampling in terms of both accuracy and efficiency.
更多查看译文
关键词
neural network,prediction,interaction,multi-relational,drug-drug
AI 理解论文
溯源树
样例
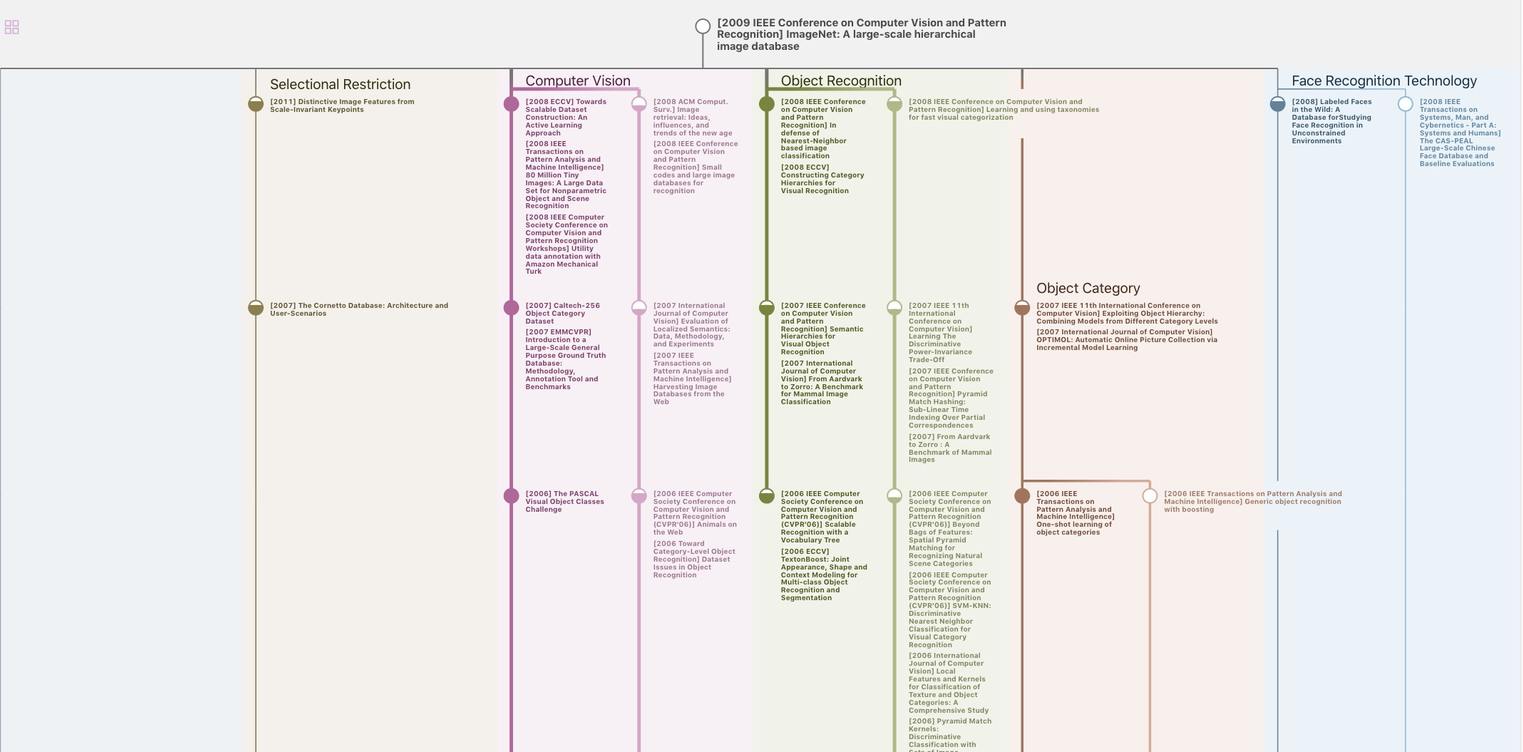
生成溯源树,研究论文发展脉络
Chat Paper
正在生成论文摘要