The Role of Entropy in Guiding a Connection Prover
AUTOMATED REASONING WITH ANALYTIC TABLEAUX AND RELATED METHODS, TABLEAUX 2021(2021)
摘要
In this work we study how to learn good algorithms for selecting reasoning steps in theorem proving. We explore this in the connection tableau calculus implemented by leanCoP where the partial tableau provides a clean and compact notion of a state to which a limited number of inferences can be applied. We start by incorporating a state-of-the-art learning algorithm - a graph neural network (GNN) into the plCoP theorem prover. Then we use it to observe the system's behavior in a reinforcement learning setting, i.e., when learning inference guidance from successful Monte-Carlo tree searches on many problems. Despite its better pattern matching capability, the GNN initially performs worse than a simpler previously used learning algorithm. We observe that the simpler algorithm is less confident, i.e., its recommendations have higher entropy. This leads us to explore how the entropy of the inference selection implemented via the neural network influences the proof search. This is related to research in human decision-making under uncertainty, and in particular the probability matching theory. Our main result shows that a proper entropy regularization, i.e., training the GNN not to be overconfident, greatly improves plCoP's performance on a large mathematical corpus.
更多查看译文
关键词
Automated theorem proving, Machine learning, Reinforcement learning, Graph neural networks, Connection calculus, Entropy regularization
AI 理解论文
溯源树
样例
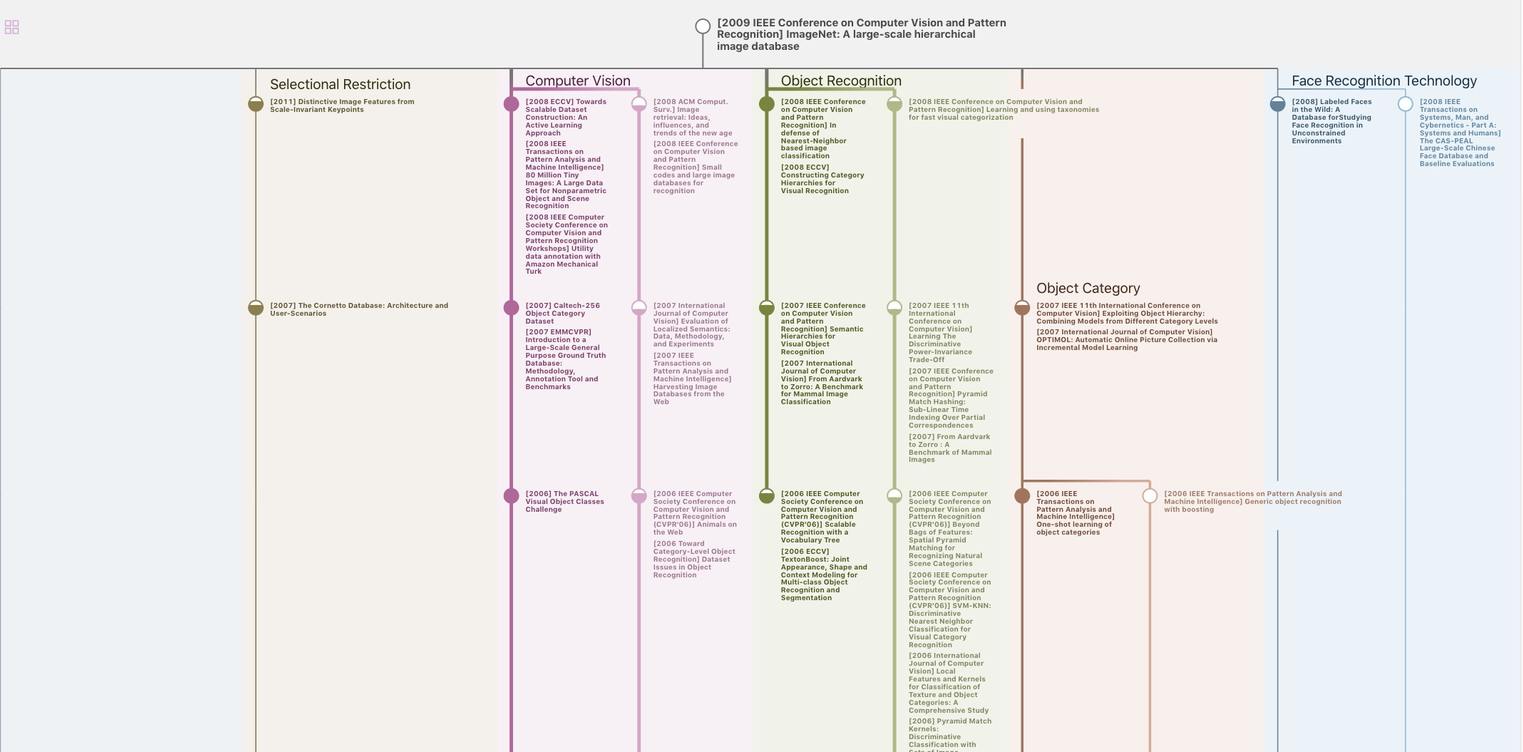
生成溯源树,研究论文发展脉络
Chat Paper
正在生成论文摘要