Gradient-based Data Subversion Attack Against Binary Classifiers
CoRR(2021)
摘要
Machine learning based data-driven technologies have shown impressive performances in a variety of application domains. Most enterprises use data from multiple sources to provide quality applications. The reliability of the external data sources raises concerns for the security of the machine learning techniques adopted. An attacker can tamper the training or test datasets to subvert the predictions of models generated by these techniques. Data poisoning is one such attack wherein the attacker tries to degrade the performance of a classifier by manipulating the training data. In this work, we focus on label contamination attack in which an attacker poisons the labels of data to compromise the functionality of the system. We develop Gradient-based Data Subversion strategies to achieve model degradation under the assumption that the attacker has limited-knowledge of the victim model. We exploit the gradients of a differentiable convex loss function (residual errors) with respect to the predicted label as a warm-start and formulate different strategies to find a set of data instances to contaminate. Further, we analyze the transferability of attacks and the susceptibility of binary classifiers. Our experiments show that the proposed approach outperforms the baselines and is computationally efficient.
更多查看译文
关键词
data subversion attack,binary classifiers,gradient-based
AI 理解论文
溯源树
样例
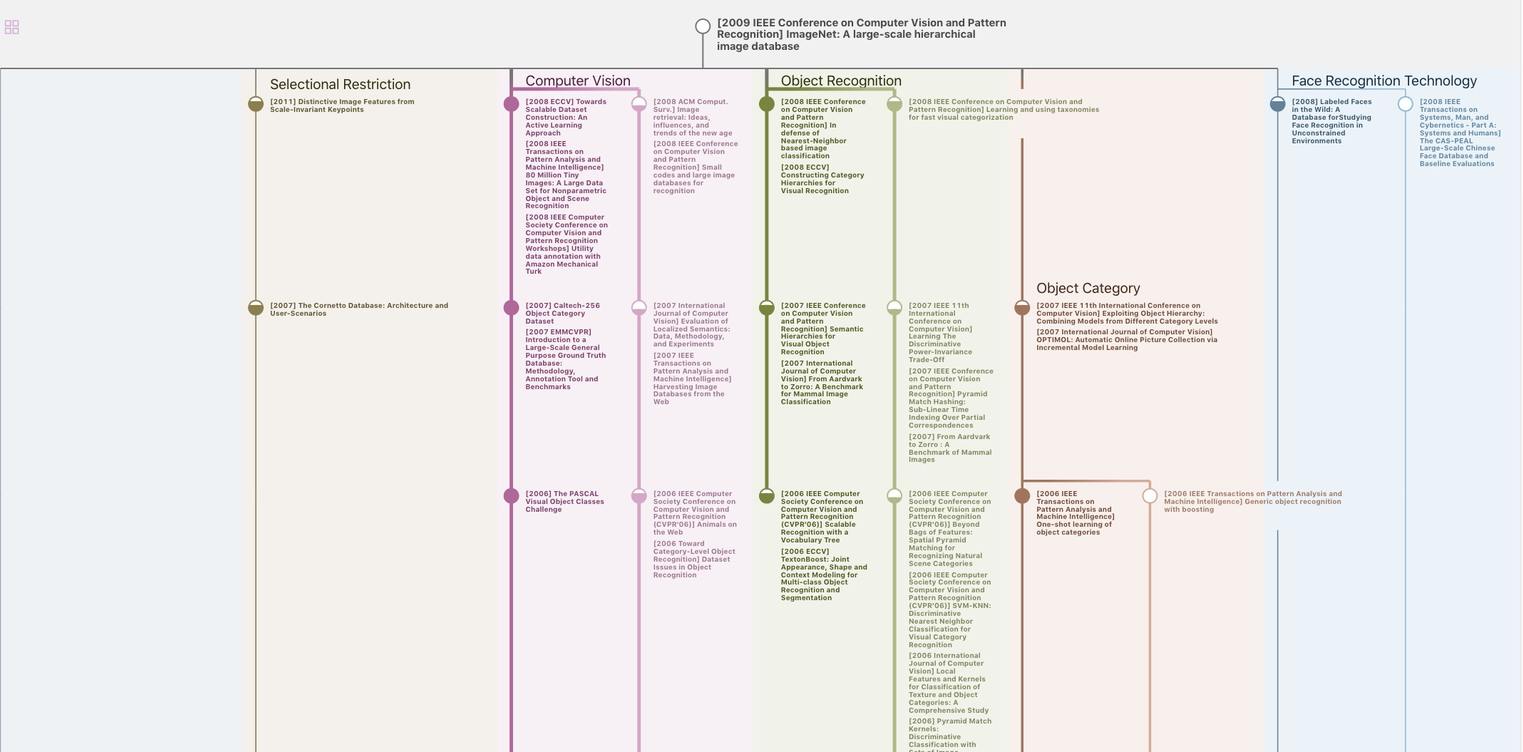
生成溯源树,研究论文发展脉络
Chat Paper
正在生成论文摘要