REAL-TIME COARSE-TO-FINE DEPTH ESTIMATION ON STEREO ENDOSCOPIC IMAGES WITH SELF-SUPERVISED LEARNING
2021 IEEE 18TH INTERNATIONAL SYMPOSIUM ON BIOMEDICAL IMAGING (ISBI)(2021)
摘要
Fast and accurate depth estimation is an essential task in computer-assisted surgery and robotics, especially for endoscopic and microscopic procedures. We propose a real-time stereo matching model using a staged, coarse-to-fine architecture to estimate disparity from medical stereo camera data with self-supervised learning. Our model processes images with a resolution of 1280 x 1024 pixels beyond 60 fps, with similar accuracy to the semi-global matching algorithm, and does not require any ground truth depth for training. We evaluated our model on two stereo endoscopic datasets from the literature. A mean absolute error below 1.5 mm and root mean square error below 1.9 mm were identified.
更多查看译文
关键词
Stereo Endoscopy, Disparity Estimation, Unsupervised Learning, Stereo Matching, Neural Networks
AI 理解论文
溯源树
样例
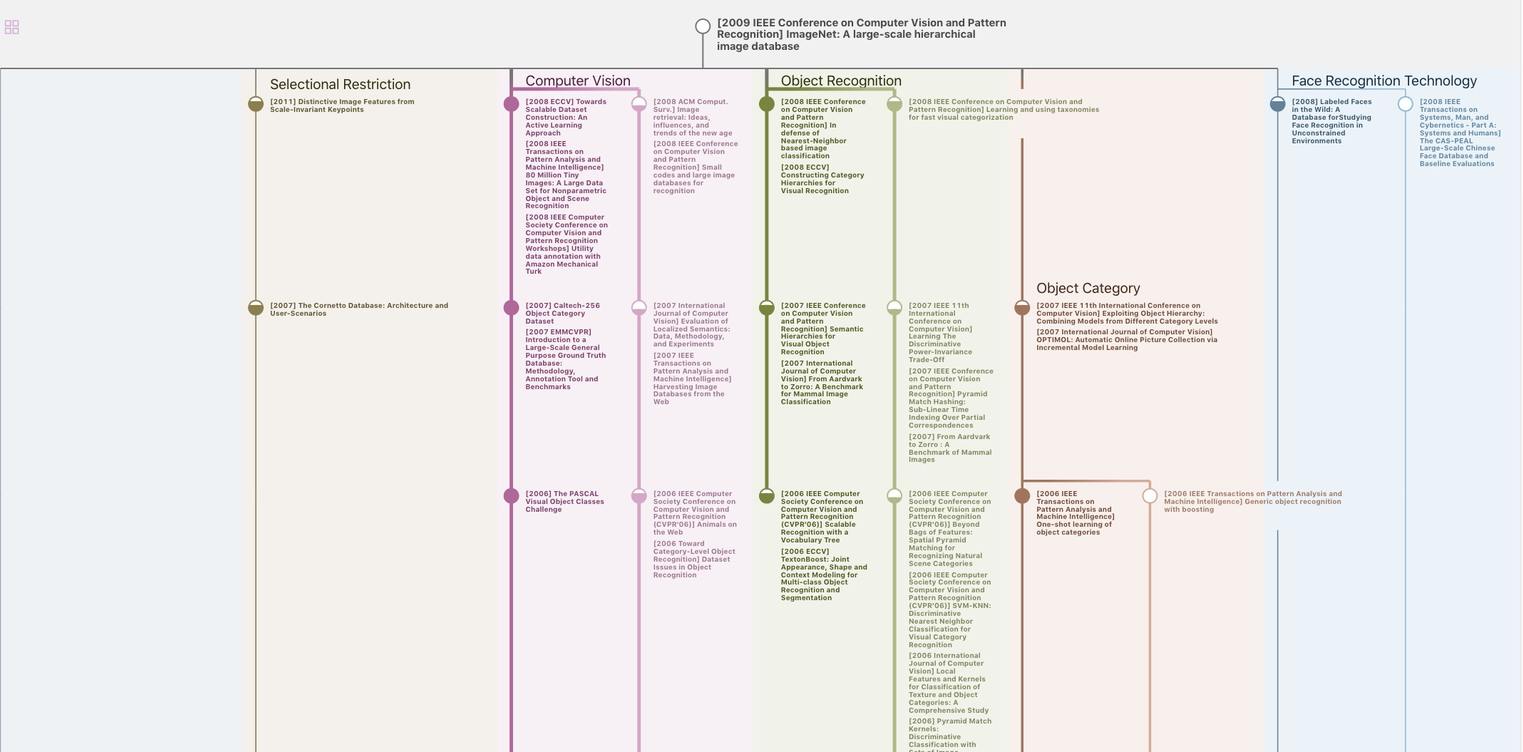
生成溯源树,研究论文发展脉络
Chat Paper
正在生成论文摘要