On Computing the Diameter of (Weighted) Link Streams
SEA(2022)
摘要
A weighted link stream is a pair ( V , 𝔼) comprising V , the set of nodes, and 𝔼, the list of temporal edges ( u,v,t ,λ) , where u,v are two nodes in V , t is the starting time of the temporal edge, and λ is its travel time. By making use of this model, different notions of diameter can be defined, which refer to the following distances: earliest arrival time, latest departure time, fastest time, and shortest time. After proving that any of these diameters cannot be computed in time sub-quadratic with respect to the number of temporal edges, we propose different algorithms (inspired by the approach used for computing the diameter of graphs) that allow us to compute, in practice very efficiently, the diameter of quite large real-world weighted link stream for several definitions of the diameter. In the case of the fastest time distance and of the shortest time distance, we introduce the notion of pivot-diameter, to deal with the fact that temporal paths cannot be concatenated in general. The pivot-diameter is the diameter restricted to the set of pair of nodes connected by a path that passes through a pivot (that is, a node at a given time instant). We prove that the problem of finding an optimal set of pivots, in terms of the number of pairs connected, is NP-hard, and we propose and experimentally evaluate several simple and fast heuristics for computing “good” pivot sets. All the proposed algorithms (for computing either the diameter or the pivot-diameter) require very often a very low number of single source (or target) best path computations. We verify the effectiveness of our approaches by means of an extensive set of experiments on real-world link streams. We also experimentally prove that the temporal version of the well-known 2-sweep technique, for computing a lower bound on the diameter of a graph, is quite effective in the case of weighted link stream, by returning very often tight bounds.
更多查看译文
关键词
Diameter,link streams,time-dependent graphs
AI 理解论文
溯源树
样例
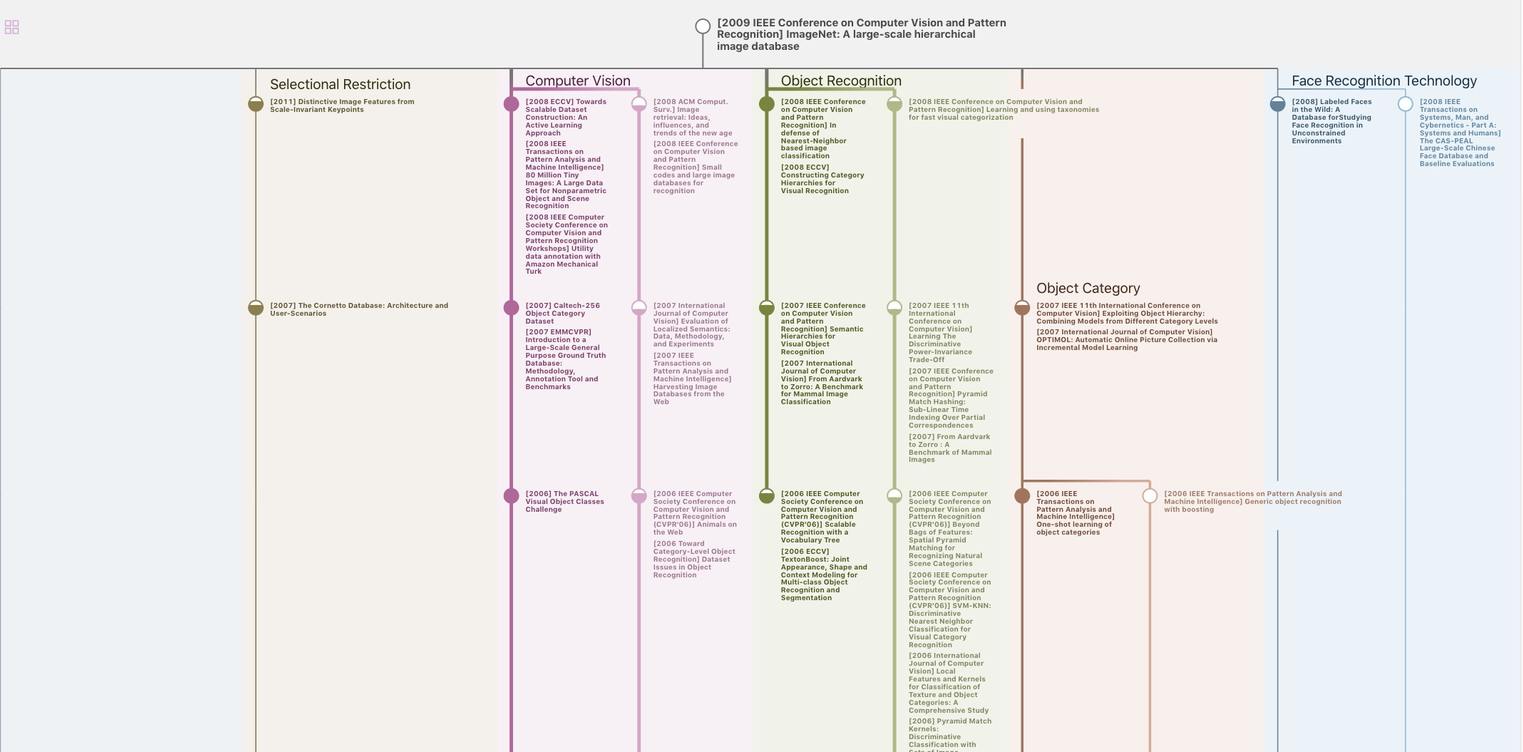
生成溯源树,研究论文发展脉络
Chat Paper
正在生成论文摘要