Differentiation of lacrimal gland tumors using the multi-model MRI: classification and regression tree (CART)-based analysis
Acta radiologica(2022)
Abstract
Background Little is known about the value of dynamic contrast-enhanced (DCE) in combination with diffusion-weighted imaging (DWI) for the differentiation of lacrimal gland tumors. Purpose To evaluate the ability of DCE and DWI in differentiating lacrimal gland tumors. Material and Methods DCE and DWI were performed in 72 patients with lacrimal gland tumors. Time-intensity curve (TIC) patterns were categorized as type A, type B, type C, and type D. Apparent diffusion coefficient (ADC) was measured on DWI. Then, the diagnostic effectiveness of TIC in conjunction with ADC was assessed using classification and regression tree (CART) analysis. Results Type A tumors were all epithelial; they could be further separated into pleomorphic adenoma sand carcinomas. Type B tumors were all non-epithelial tumors, which could be further separated into benign inflammatory infiltrates (BIIs) and lymphomas. Type C tumors contained both carcinomas and non-epithelial tumors, which could be diagnosed into carcinomas, BIIs and lymphomas. Type D tumors were all PAs. The mean ADC of epithelial tumors was significantly higher than that of non-epithelial tumors, and the mean ADC values were significantly different between PAs and carcinomas. Besides, the mean ADC value of BIIs was higher than that of lymphomas. Therefore, the CART decision tree made by ADC and TIC had a predictive accuracy of 86.1%, differentiating lacrimal gland tumors effectively. Conclusion Combined DCE and DWI-MRI can efficiently differentiate lacrimal gland tumors which can be of help to ophthalmologists in the diagnosis and treatment of these tumors.
MoreTranslated text
Key words
Eye neoplasms,lacrimal apparatus diseases,dynamic contrast-enhanced,diffusion-weighted imaging,classification and regression tree,CART
AI Read Science
Must-Reading Tree
Example
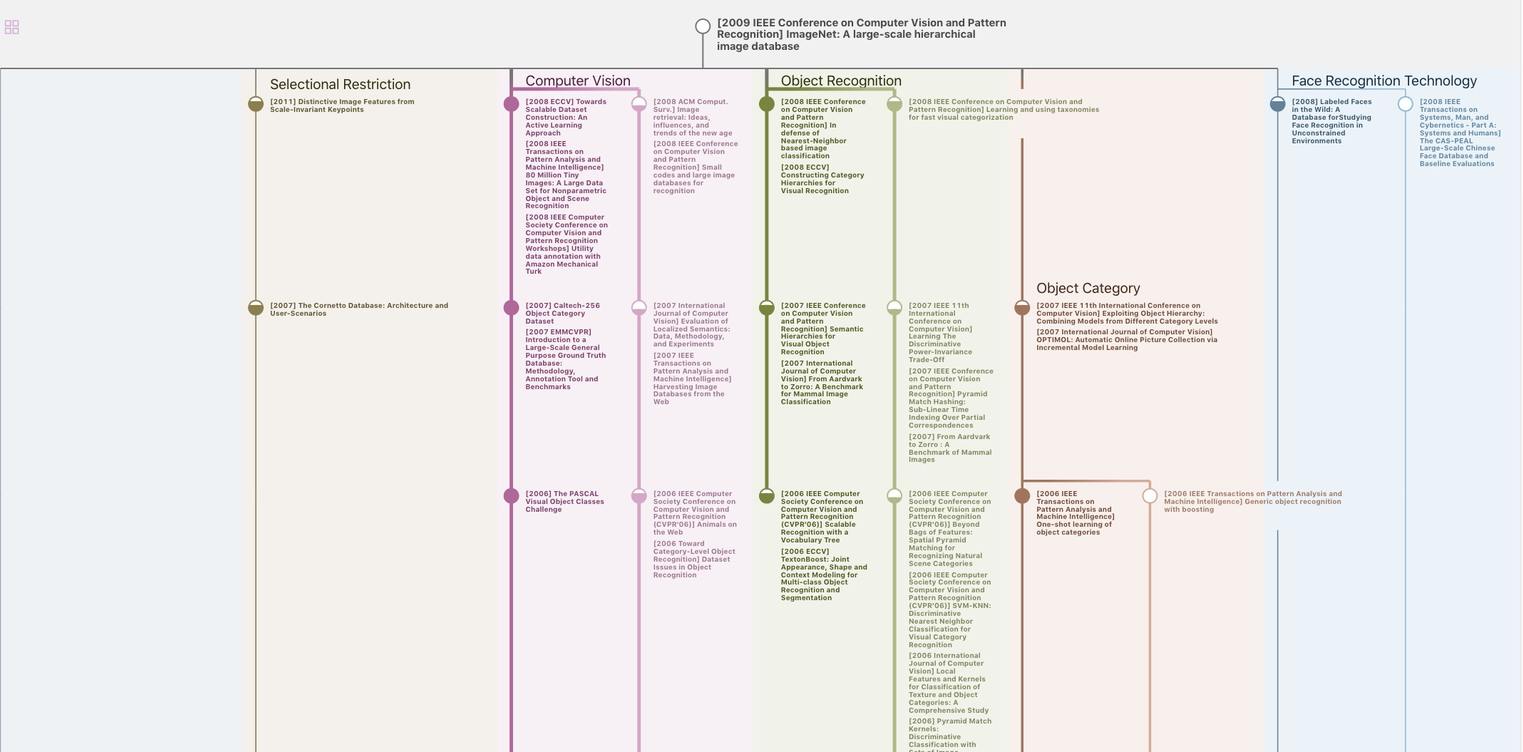
Generate MRT to find the research sequence of this paper
Chat Paper
Summary is being generated by the instructions you defined