A Multiparametric Mri-Based Radiomics Analysis To Efficiently Classify Tumor Subregions Of Glioblastoma: A Pilot Study In Machine Learning
JOURNAL OF CLINICAL MEDICINE(2021)
摘要
Glioblastoma multiforme (GBM) carries a poor prognosis and usually presents with heterogenous regions of a necrotic core, solid part, peritumoral tissue, and peritumoral edema. Accurate demarcation on magnetic resonance imaging (MRI) between the active tumor region and perifocal edematous extension is essential for planning stereotactic biopsy, GBM resection, and radiotherapy. We established a set of radiomics features to efficiently classify patients with GBM and retrieved cerebral multiparametric MRI, including contrast-enhanced T1-weighted (T1-CE), T2-weighted, T2-weighted fluid-attenuated inversion recovery, and apparent diffusion coefficient images from local patients with GBM. A total of 1316 features on the raw MR images were selected for each annotated area. A leave-one-out cross-validation was performed on the whole dataset, the different machine learning and deep learning techniques tested; random forest achieved the best performance (average accuracy: 93.6% necrosis, 90.4% solid part, 95.8% peritumoral tissue, and 90.4% peritumoral edema). The features from the enhancing tumor and the tumor shape elongation of peritumoral edema region for high-risk groups from T1-CE. The multiparametric MRI-based radiomics model showed the efficient classification of tumor subregions of GBM and suggests that prognostic radiomic features from a routine MRI exam may also be significantly associated with key biological processes that affect the response to chemotherapy in GBM.
更多查看译文
关键词
glioblastoma, MRI, quantitative imaging, oncologic imaging, radiomics, texture analysis, ground truth, machine learning, precision medicine
AI 理解论文
溯源树
样例
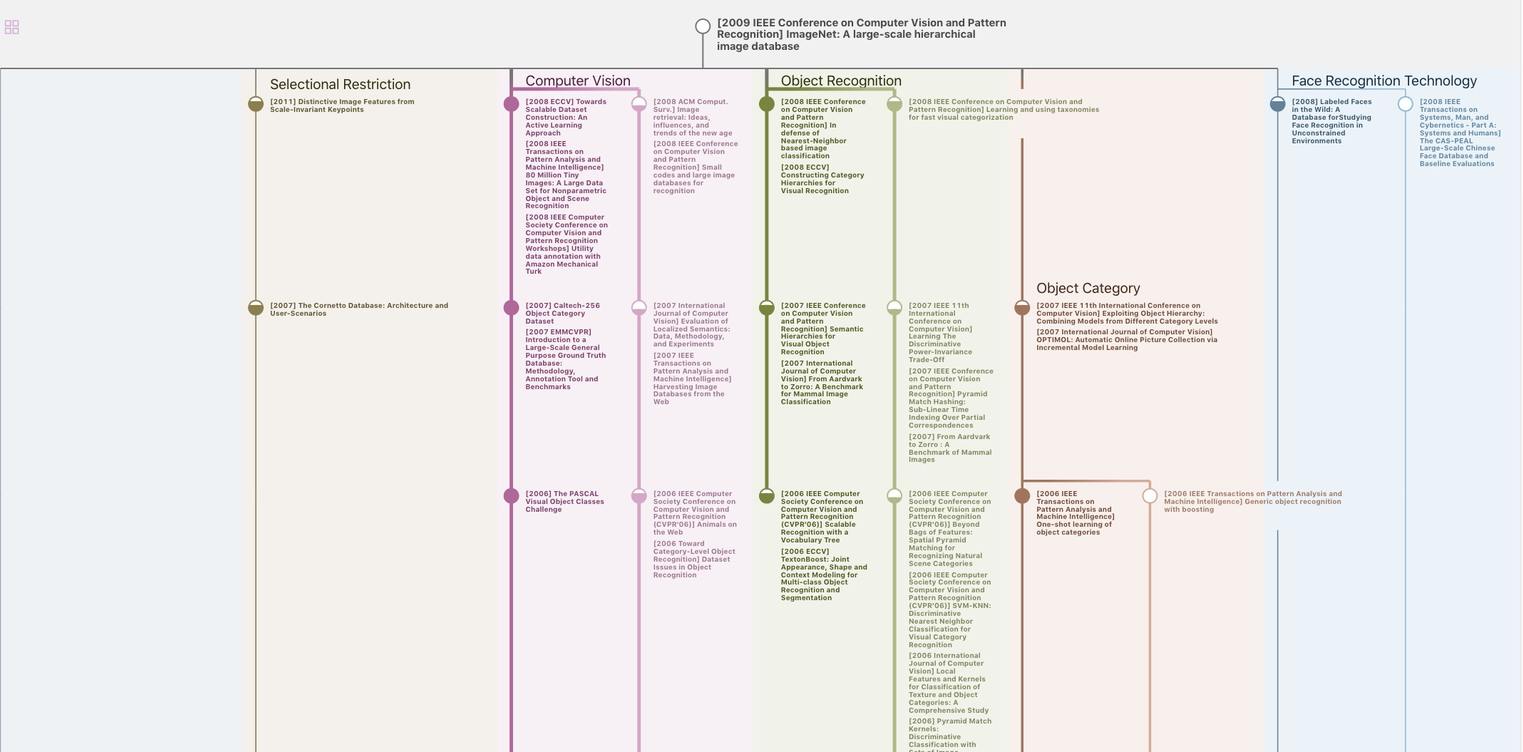
生成溯源树,研究论文发展脉络
Chat Paper
正在生成论文摘要