High-Dimensional Interaction Detection With False Sign Rate Control
JOURNAL OF BUSINESS & ECONOMIC STATISTICS(2022)
摘要
Identifying interaction effects is fundamentally important in many scientific discoveries and contemporary applications, but it is challenging since the number of pairwise interactions increases quadratically with the number of covariates and that of higher-order interactions grows even faster. Although there is a growing literature on interaction detection, little work has been done on the prediction and false sign rate on interaction detection in ultrahigh-dimensional regression models. This article fills such a gap. More specifically, in this article we establish some theoretical results on interaction selection for ultrahigh-dimensional quadratic regression models under random designs. We prove that the examined method enjoys the same oracle inequalities as the lasso estimator and further admits an explicit bound on the false sign rate. Moreover, the false sign rate can be asymptotically vanishing. These new theoretical characterizations are confirmed by simulation studies. The performance of our proposed approach is further illustrated through a real data application.
更多查看译文
关键词
False sign rate, High dimensionality, Interaction screening, Interaction selection, Nonconvex learning, Sure independence screening
AI 理解论文
溯源树
样例
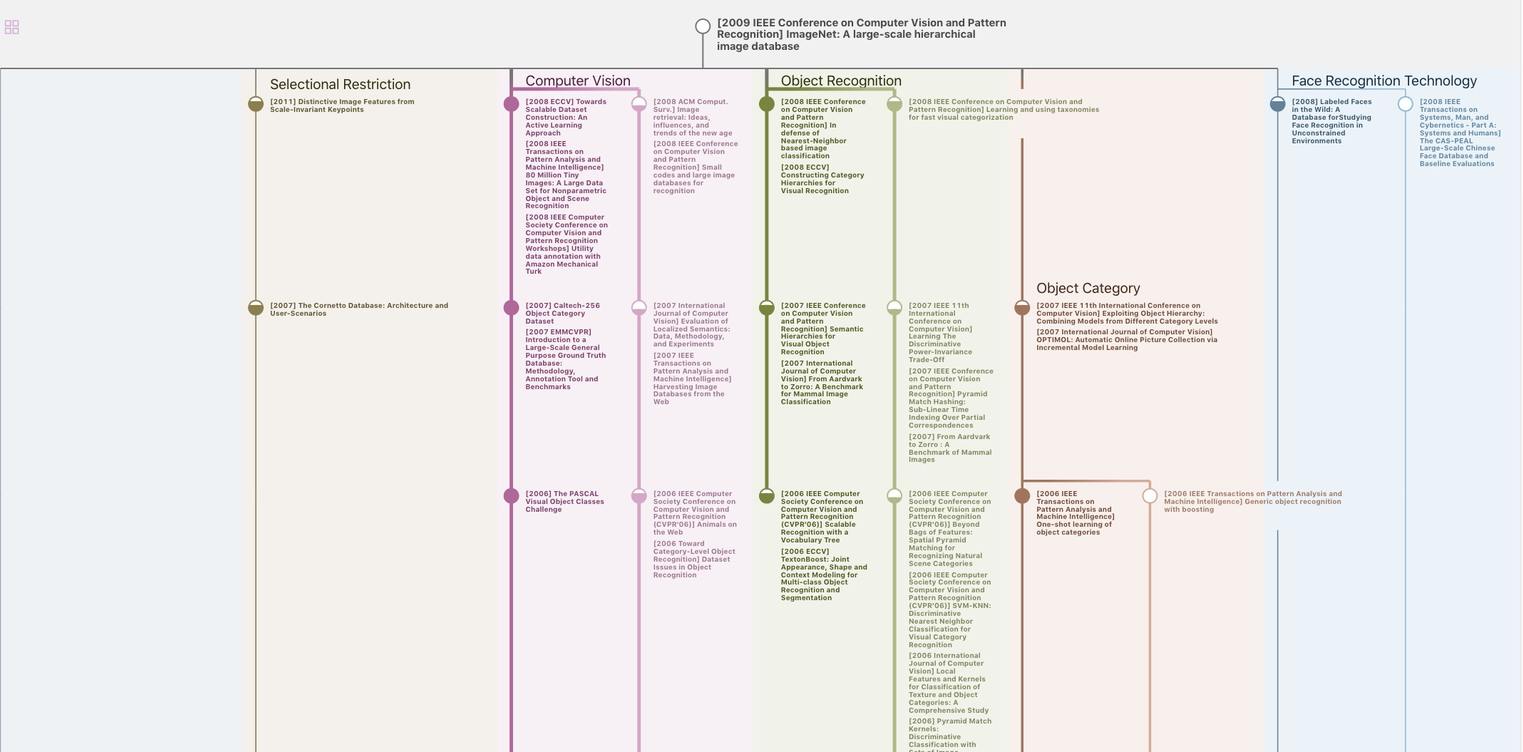
生成溯源树,研究论文发展脉络
Chat Paper
正在生成论文摘要