Multilevel Feature Fusion Dilated Convolutional Network For Semantic Segmentation
INTERNATIONAL JOURNAL OF ADVANCED ROBOTIC SYSTEMS(2021)
摘要
Recently, convolutional neural network (CNN) has led to significant improvement in the field of computer vision, especially the improvement of the accuracy and speed of semantic segmentation tasks, which greatly improved robot scene perception. In this article, we propose a multilevel feature fusion dilated convolution network (Refine-DeepLab). By improving the space pyramid pooling structure, we propose a multiscale hybrid dilated convolution module, which captures the rich context information and effectively alleviates the contradiction between the receptive field size and the dilated convolution operation. At the same time, the high-level semantic information and low-level semantic information obtained through multi-level and multi-scale feature extraction can effectively improve the capture of global information and improve the performance of large-scale target segmentation. The encoder-decoder gradually recovers spatial information while capturing high-level semantic information, resulting in sharper object boundaries. Extensive experiments verify the effectiveness of our proposed Refine-DeepLab model, evaluate our approaches thoroughly on the PASCAL VOC 2012 data set without MS COCO data set pretraining, and achieve a state-of-art result of 81.73% mean interaction-over-union in the validate set.
更多查看译文
关键词
Semantic segmentation, convolutional neural network, deep learning, computer vision, robot vision
AI 理解论文
溯源树
样例
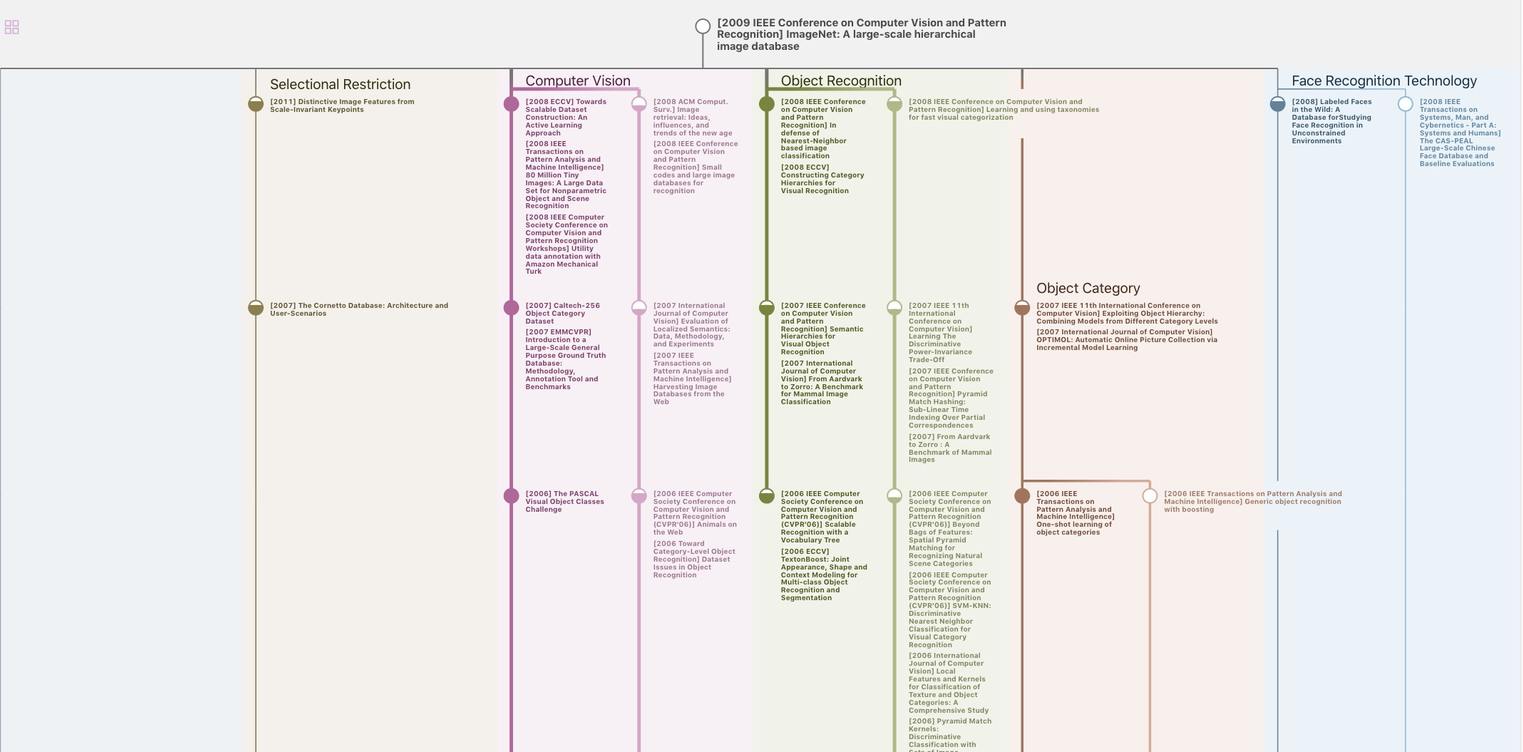
生成溯源树,研究论文发展脉络
Chat Paper
正在生成论文摘要