A Comparative Study On Handcrafted Features V/S Deep Features For Open-Set Fingerprint Liveness Detection & Nbsp;
PATTERN RECOGNITION LETTERS(2021)
摘要
A fingerprint liveness detector is a pattern classifier that is used to distinguish a live finger from a fake (spoof) one in the context of an automated fingerprint recognition system. As liveness detectors or presentation attack detectors are vulnerable to presentation attacks, the security and reliability of fingerprint recognition are compromised. Presentation attack detection mechanisms rely on handcrafted or deep features to classify an image as live or spoof. In addition, to strengthen the security, fingerprint liveness detectors should be robust to presentation attacks fabricated using unknown fabrication materials or fingerprint sensors. In this paper, we conduct a comprehensive study on the impact of handcrafted and deep features from fingerprint images on the classification error rate of the fingerprint liveness detection task. We use LBP, LPQ and BSIF as handcrafted features and VGG-19 and Residual CNN as deep feature extractors for this study. As the problem is targeted as an open-set problem, the emphasis is on achieving better robustness and generalization capability. In our observation, handcrafted features outperformed their deep counterparts in two of the three cases under the within-dataset environment. In the cross-sensor environment, deep features obtained a better accuracy, and in the cross-dataset environment, handcrafted features obtained a lower classification error rate. (c) 2021 Elsevier B.V. All rights reserved.
更多查看译文
关键词
Liveness detection, Handcrafted v, s deep features, Open-set classification
AI 理解论文
溯源树
样例
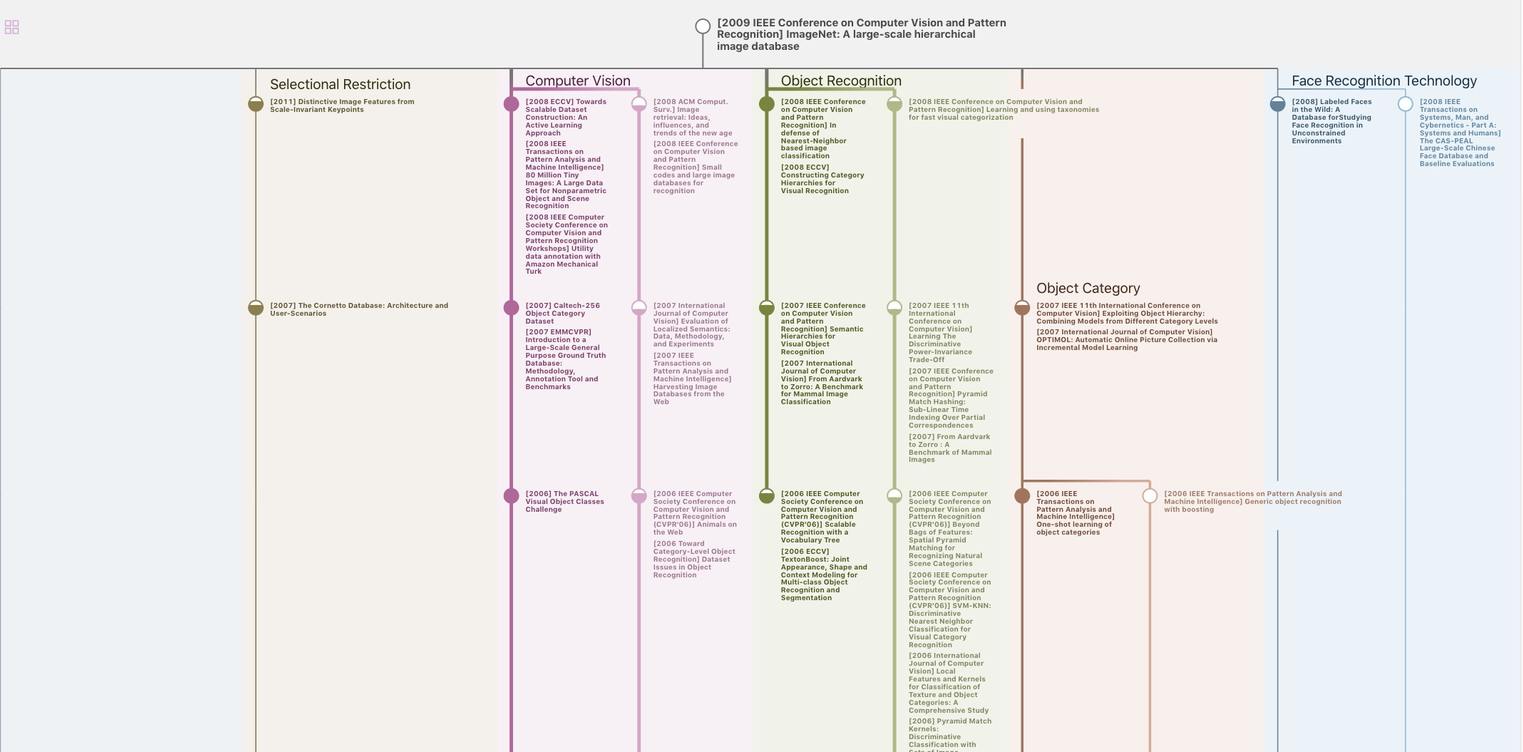
生成溯源树,研究论文发展脉络
Chat Paper
正在生成论文摘要