Learning dynamics of gradient descent optimization in deep neural networks
SCIENCE CHINA-INFORMATION SCIENCES(2021)
摘要
Stochastic gradient descent (SGD)-based optimizers play a key role in most deep learning models, yet the learning dynamics of the complex model remain obscure. SGD is the basic tool to optimize model parameters, and is improved in many derived forms including SGD momentum and Nesterov accelerated gradient (NAG). However, the learning dynamics of optimizer parameters have seldom been studied. We propose to understand the model dynamics from the perspective of control theory. We use the status transfer function to approximate parameter dynamics for different optimizers as the first- or second-order control system, thus explaining how the parameters theoretically affect the stability and convergence time of deep learning models, and verify our findings by numerical experiments.
更多查看译文
关键词
learning dynamics, deep neural networks, gradient descent, control model, transfer function
AI 理解论文
溯源树
样例
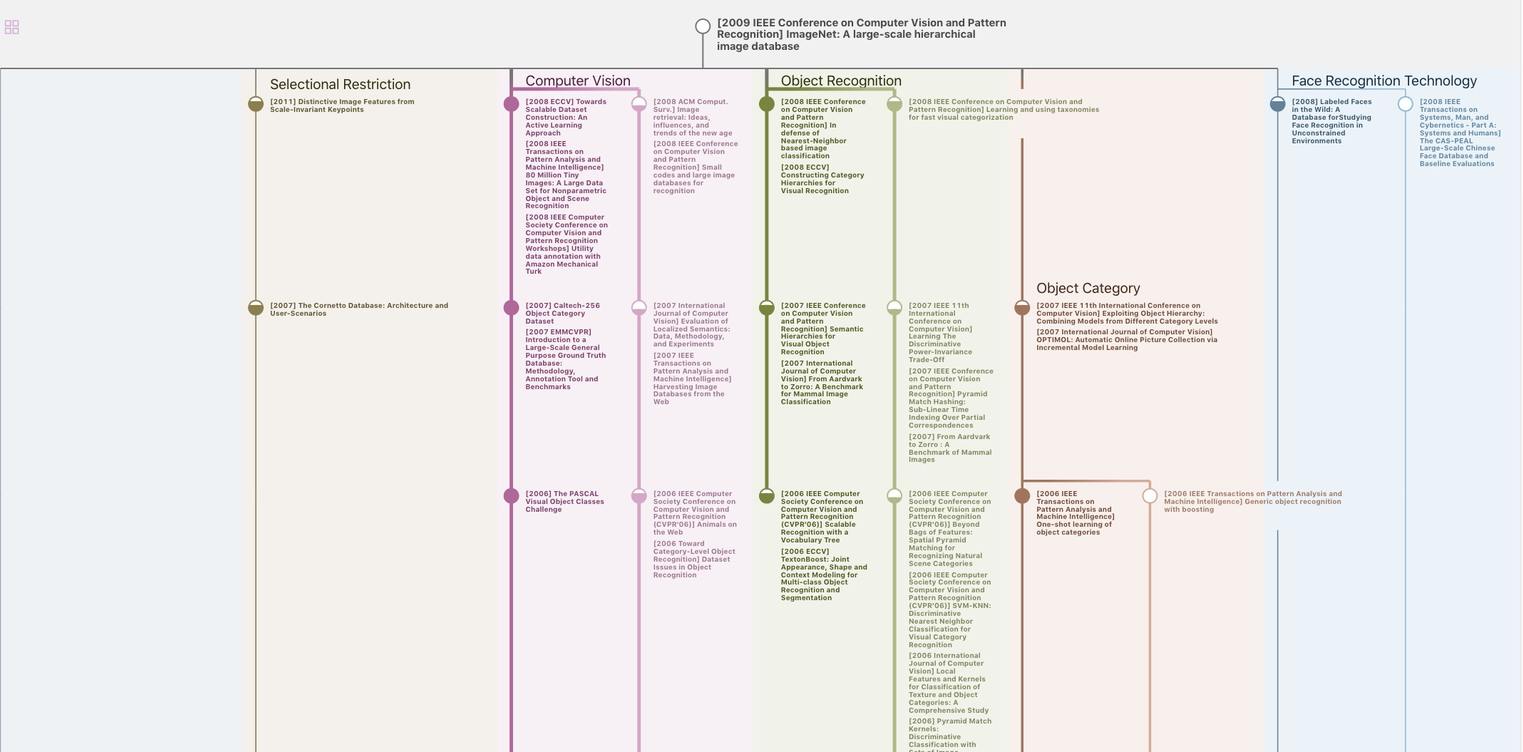
生成溯源树,研究论文发展脉络
Chat Paper
正在生成论文摘要