Dynamic Kernel CNN-LR model for people counting
APPLIED INTELLIGENCE(2021)
摘要
People Counting in images is a worthwhile task as it is widely used for public safety, emergency people planning, intelligent crowd flow, and countless other reasons. Counting the objects manually in images does not make practical sense, since it is very time-consuming, and it never gives accurate results for dense crowded images. In crowded images, as the density of the people increases, object appear to be partially encircling each other. This occlusion problem of objects limits the crowd counting ability of any traditional computer vision model. To overcome this problem, here we addressed a dynamic kernel convolution neural network-linear regression (DKCNN-LR) model for counting the exact number of people in image frames even if crowd is very dense and occlusion problem. The proposed model works in two phases, first a DKCNN model use convolution layers in such a fashion that the kernel weight of each subsequent successive layer is half of its previous convolution layer’s weight. The first three heavy kernel weight layers identify far camera regions (low-level) features, and the later light kernel weight layers help identify near-camera region (high-level) features. Second, a linear regression model is employed to perform parametric regression between the actual people count (ground truth) and the estimated count (predicted values). The performance of the proposed model tested on three challenging and different quality benchmark datasets in terms of MAE, RMSE, Pearson-R and R 2 . The DKCNN-LR model secured MAE, RMSE on Mall dataset is 1.65, 2.76, on Beijing-BRT 1.43, 1.87 and on SmartCity dataset it is 2.69 and 10.69. These results confirm that the proposed model is quite reliable, effective and robust for real situations.
更多查看译文
关键词
Crowd counting, Ground truth, Computer vision, Deep learning, R-Square, Pearson-R
AI 理解论文
溯源树
样例
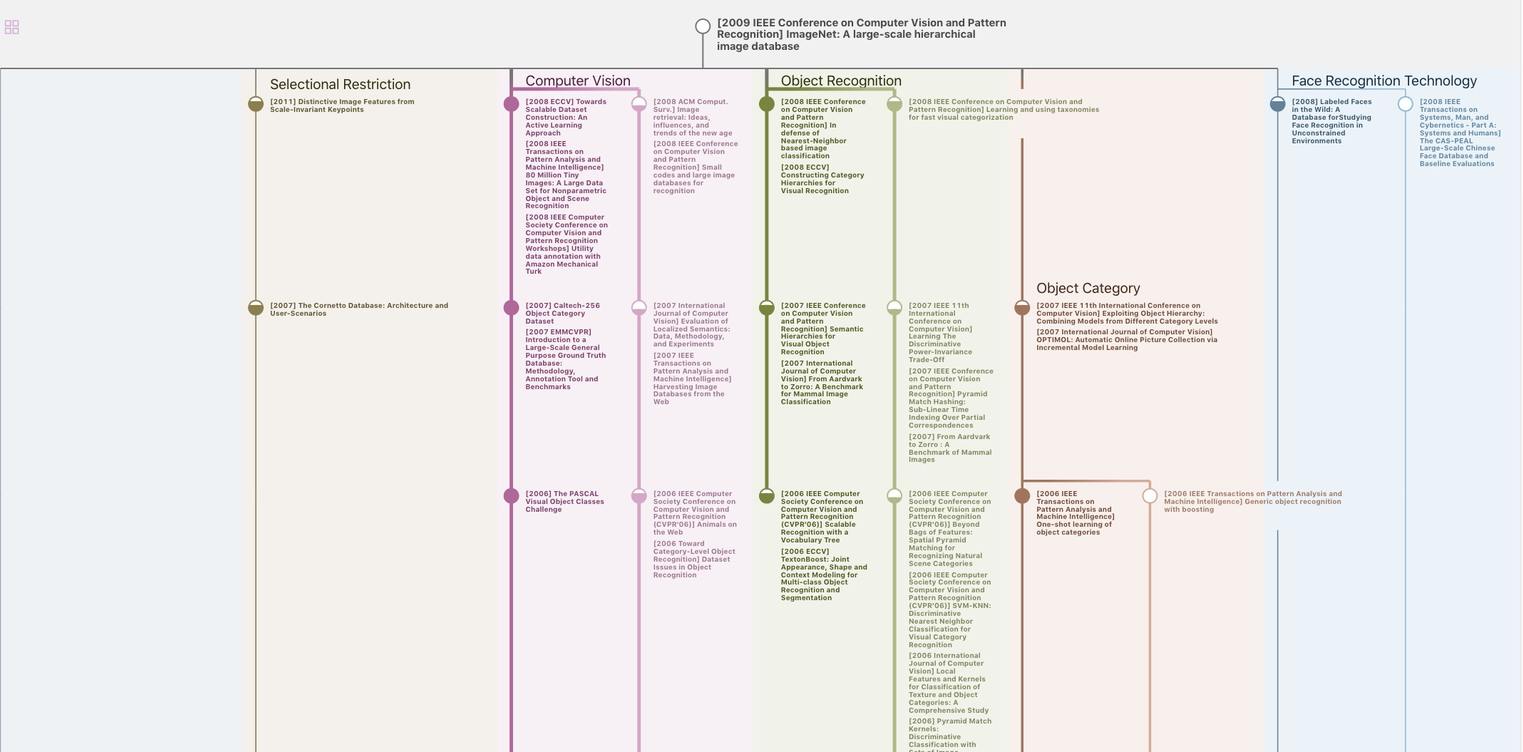
生成溯源树,研究论文发展脉络
Chat Paper
正在生成论文摘要