Improving paragraph-level question generation with extended answer network and uncertainty-aware beam search
Information Sciences(2021)
摘要
Question Generation (QG), which aims to generate a question given the relevant context, is essential to build conversational and question–answering systems. Existing neural question generation models suffer from the inadequate representation of the target answer and inappropriate techniques to reduce repetition. To address these issues, we propose an Extended Answer-aware Network (EAN) which is trained with Word-based Coverage Mechanism (WCM) and decoded with Uncertainty-aware Beam Search (UBS). The EAN represents the target answer by its surrounding sentence with an encoder and incorporates the extended answer to paragraph representation using gated paragraph-to-answer attention to tackle the problem of the inadequate representation of the target answer. To reduce undesirable repetition, the WCM penalizes repeatedly attending to the same words of different time-steps in the training stage. The UBS incorporates an uncertainty score into beam search to alleviate text degeneration and reduce repeated copying words of the paragraph. Experiments on two benchmark datasets demonstrate the effectiveness of our methods of paragraph-level question generation. Specifically, our model has achieved 4.2% and 27.2% improvement over BLEU-4 compared to the best paragraph-level QG baseline in SQuAD and NewsQA datasets respectively.
更多查看译文
关键词
Question generation,Attention network,Coverage mechanism,Beam search
AI 理解论文
溯源树
样例
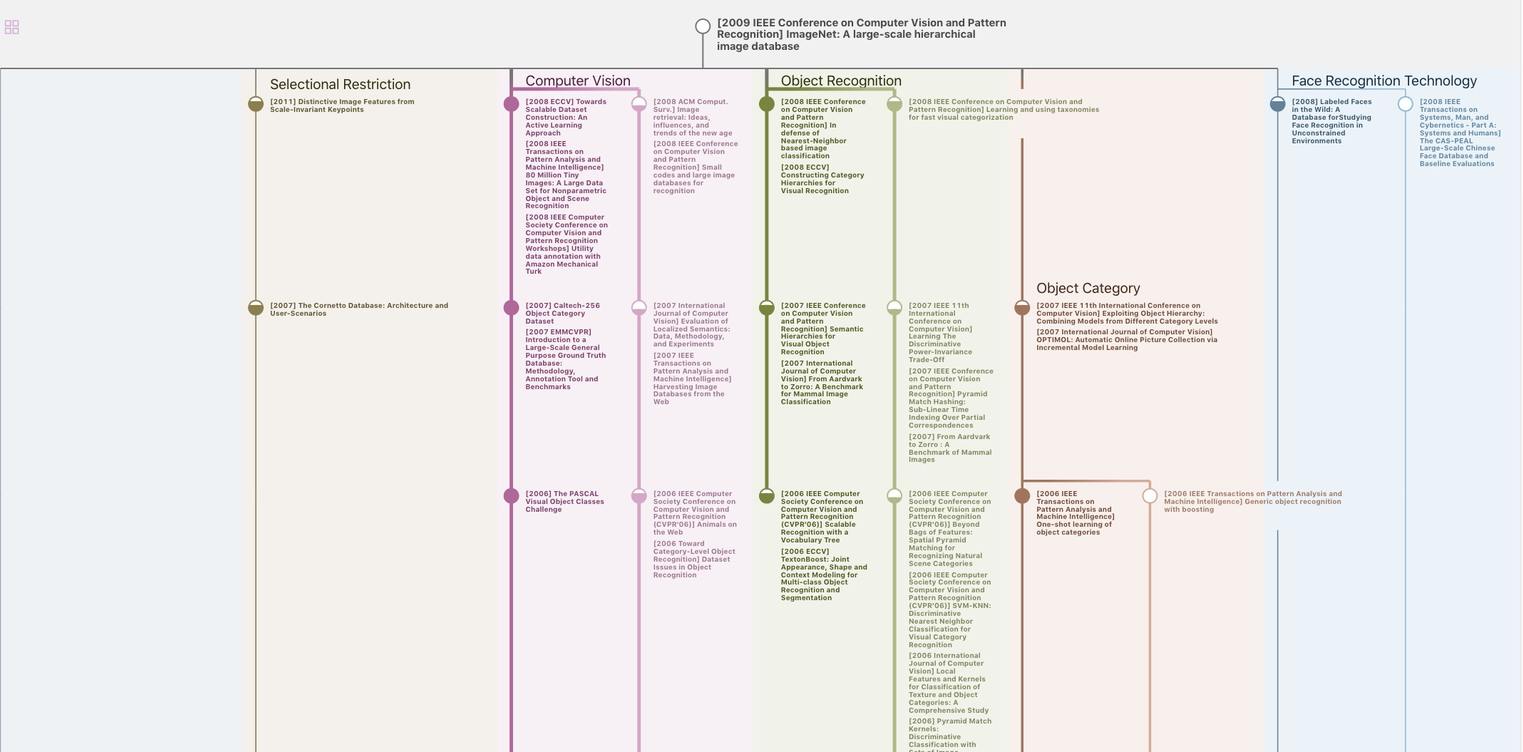
生成溯源树,研究论文发展脉络
Chat Paper
正在生成论文摘要