Graph Anomaly Detection Using Dictionary Learning
IFAC-PapersOnLine(2020)
摘要
Abstract Anomaly detection in networked signals often boils down to identifying an underlying graph structure on which the abnormal occurrence rests on. We investigate the problem of learning graph structure representations using adaptations of dictionary learning aimed at encoding connectivity patterns. In particular, we adapt dictionary learning strategies to the specificity of network topologies and propose new methods that impose Laplacian structure on the dictionaries themselves. In one adaptation we focus on classifying topologies by working directly on the graph Laplacian and cast the learning problem to accommodate its 2D structure. We tackle the same problem by learning dictionaries which consist of vectorized atomic Laplacians, and provide a block coordinate descent scheme to solve the new dictionary learning formulation. Imposing Laplacian structure on the dictionaries is also proposed in an adaptation of the Single Block Orthogonal learning method. Results on synthetic graph datasets comprising different graph topologies confirm the potential of dictionaries to directly represent graph structure information.
更多查看译文
关键词
anomaly detection, dictionary learning, graph Laplacian classification
AI 理解论文
溯源树
样例
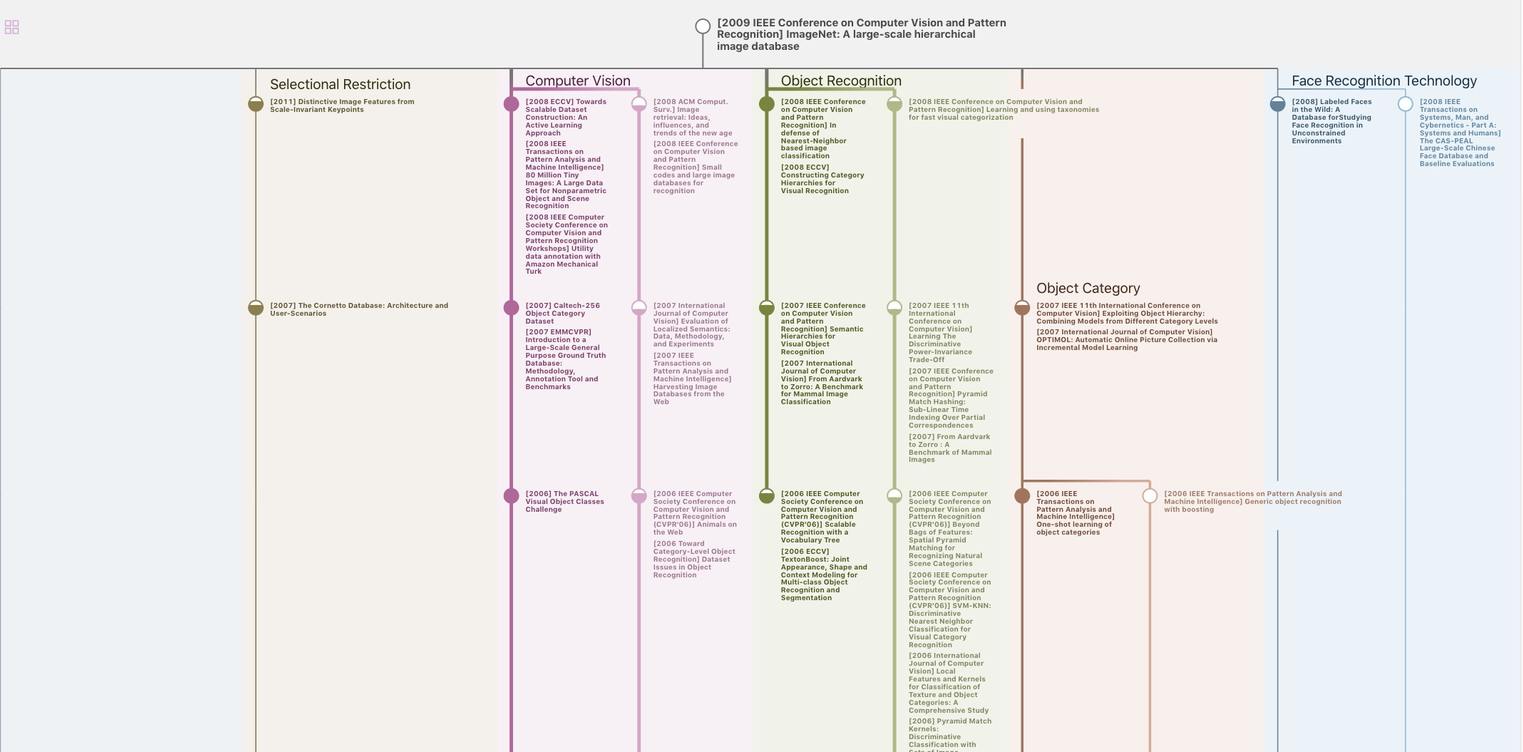
生成溯源树,研究论文发展脉络
Chat Paper
正在生成论文摘要