Automated Extraction Of Physical Parameters From Experimentally Obtained Thermal Profiles Using A Machine Learning Approach
COMPUTATIONAL MATERIALS SCIENCE(2021)
摘要
Computational modeling is playing an increasingly important role in designing novel experiments and enhancing existing ones. However, these models require input parameters that are sufficiently accurate in order for the predictions to be quantitatively reliable. Frequently, some of these parameters are not directly measurable or have a large uncertainty. We propose a machine learning approach to automatically extract these uncertain or unknown parameters indirectly from experimental measurements that depend on the combination of parameters in a complex manner. The algorithm iteratively refines the possible range of each parameter based on the mean and standard deviation of the sampling simulations that are at the 10th percentile or lower in error until the convergence criteria is met, which is followed by a multivariate quadratic fit and minimization. To demonstrate its applicability, we apply the algorithm to determine physical parameters during the fitting of temperature profiles extracted from in situ synchrotron measurements of an optical floating zone furnace used for hightemperature crystal growth. We built a thermal transfer model in COMSOL Multiphysics (R) software that considers an Al2O3 sample that is heated by a power source and thermal conduction and cooled due to natural air convection and thermal radiation. A set of experimentally measured steady-state temperature profiles of a heated Al2O3 sample is used as training data to determine the parameter set using the algorithm, which resulted in a close match. Using this parameter set, we also simulated the time-dependent temperatures, which yielded good agreement to the corresponding experimental measurement. We conclude that the steady-state temperature profiles suffice as training data, eliminating the need for additional in situ measurements of the dynamic experimental state for robust model parameterization.
更多查看译文
关键词
Machine learning, Iterative method, Optimization, Heat transfer, Parameterization, Optical floating zone
AI 理解论文
溯源树
样例
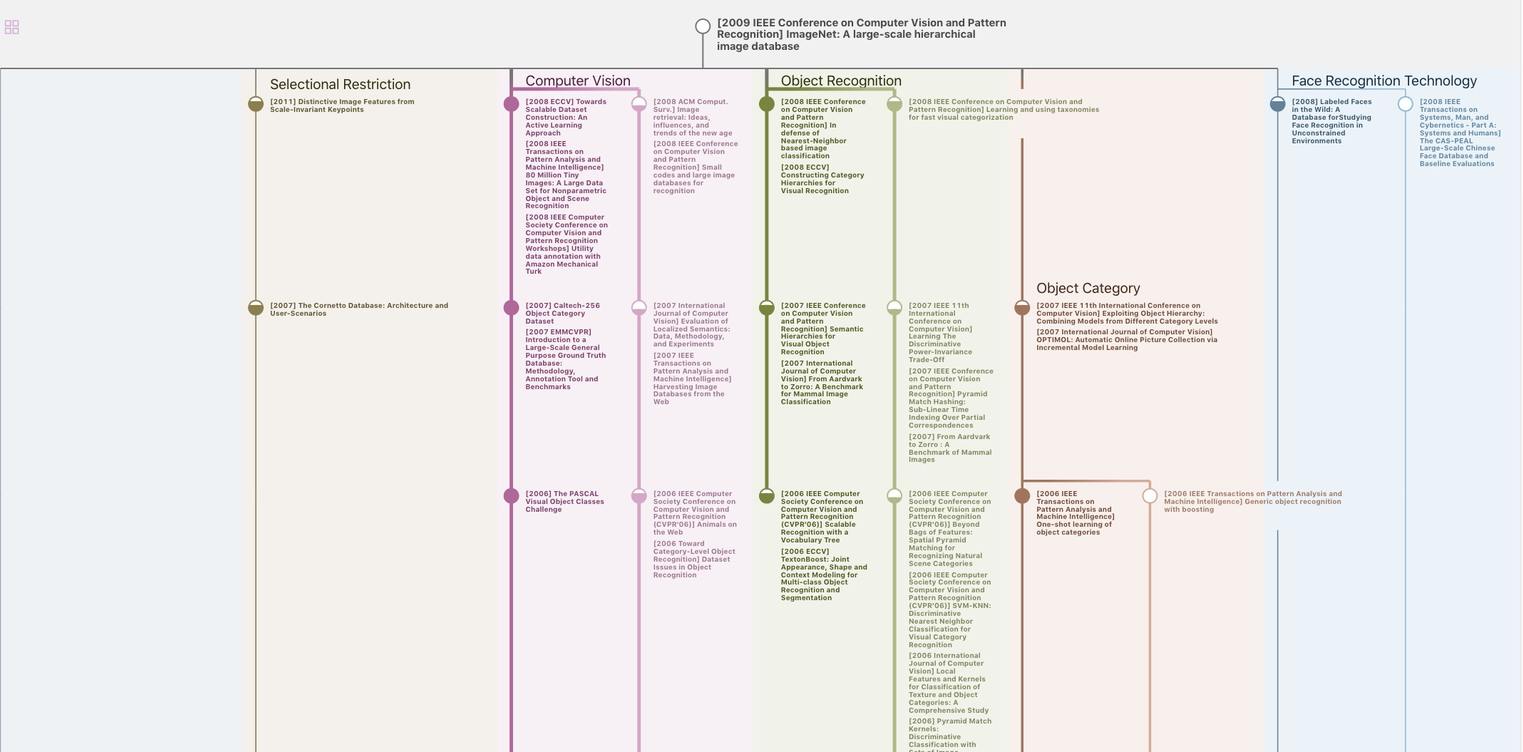
生成溯源树,研究论文发展脉络
Chat Paper
正在生成论文摘要