Land Cover Classification of Multispectral LiDAR Data With an Efficient Self-Attention Capsule Network
IEEE GEOSCIENCE AND REMOTE SENSING LETTERS(2022)
摘要
Periodically conducting land cover mapping plays a vital role in monitoring the status and changes of the land use. The up-to-date and accurate land use database serves importantly for a wide range of applications. This letter constructs an efficient self-attention capsule network (ESA-CapsNet) for land cover classification of multispectral light detection and ranging (LiDAR) data. First, formulated with a novel capsule encoder-decoder architecture, the ESA-CapsNet performs promisingly in extracting high-level, informative, and strong feature semantics for pixel-wise land cover classification by using the five types of rasterized feature images. Furthermore, designed with a novel capsule-based attention module, the channel and spatial feature encodings are comprehensively exploited to boost the feature saliency and robustness. The ESA-CapsNet is evaluated on two multispectral LiDAR data sets and achieves an advantageous performance with the overall accuracy, average accuracy, and kappa coefficient of over 98.42%, 95.15%, and 0.9776, respectively. Comparative experiments with the existing methods also demonstrate the effectiveness and applicability of the ESA-CapsNet in land cover classification tasks.
更多查看译文
关键词
Laser radar, Feature extraction, Remote sensing, Sensors, Task analysis, Labeling, Image sensors, Capsule feature attention, capsule network, land cover classification, land use mapping, multispectral light detection and ranging (LiDAR)
AI 理解论文
溯源树
样例
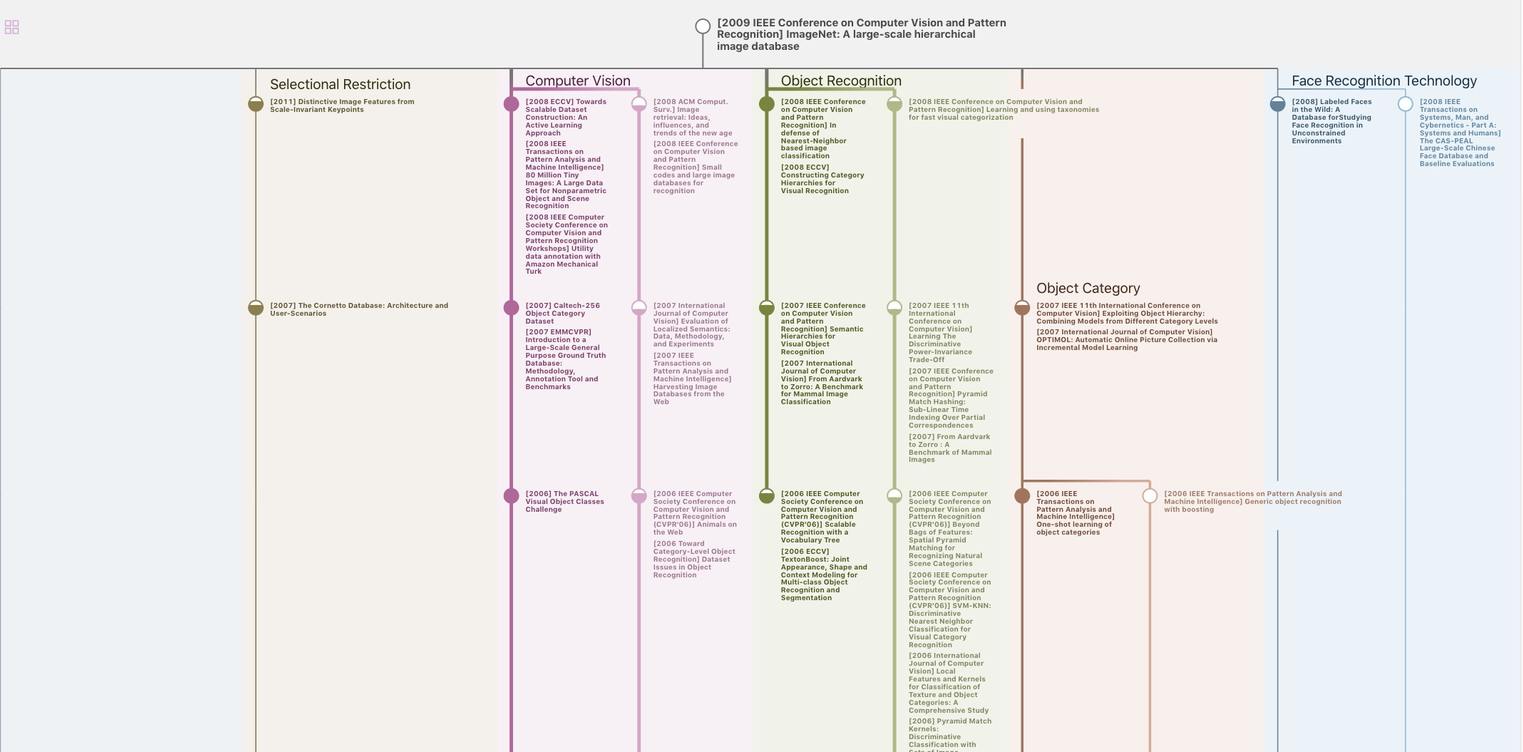
生成溯源树,研究论文发展脉络
Chat Paper
正在生成论文摘要