Evolving graph convolutional networks for neural architecture search
NEURAL COMPUTING & APPLICATIONS(2021)
摘要
As neural architecture search (NAS) becomes an increasingly adopted method to design network architectures, various methods have been proposed to speedup the process. Besides proxy evaluation tasks, weight sharing, and scaling down the evaluated architectures, performance-predicting models exhibit multiple advantages. Eliminating the need to train candidate architectures and enabling transfer learning between datasets, researchers can also utilize them as a surrogate function for Bayesian optimization. On the other hand, graph convolutional networks (GCNs) have also been increasingly adopted for various tasks, enabling deep learning techniques on graphs without feature engineering. In this paper, we employ an evolutionary-based NAS method to evolve GCNs for the problem of predicting the relative performance of various architectures included in the NAS-Bench-101 dataset. By fine-tuning the architecture generated by our methodology, we manage to achieve a Kendall’s tau correlation coefficient of 0.907 between 1050 completely unseen architectures, utilizing only 450 samples, while also outperforming a strong baseline on the same task. Furthermore, we validate our method on custom global search space architectures, generated for the Fashion-MNIST dataset.
更多查看译文
关键词
Neural architecture search, Graph neural networks, Ranking
AI 理解论文
溯源树
样例
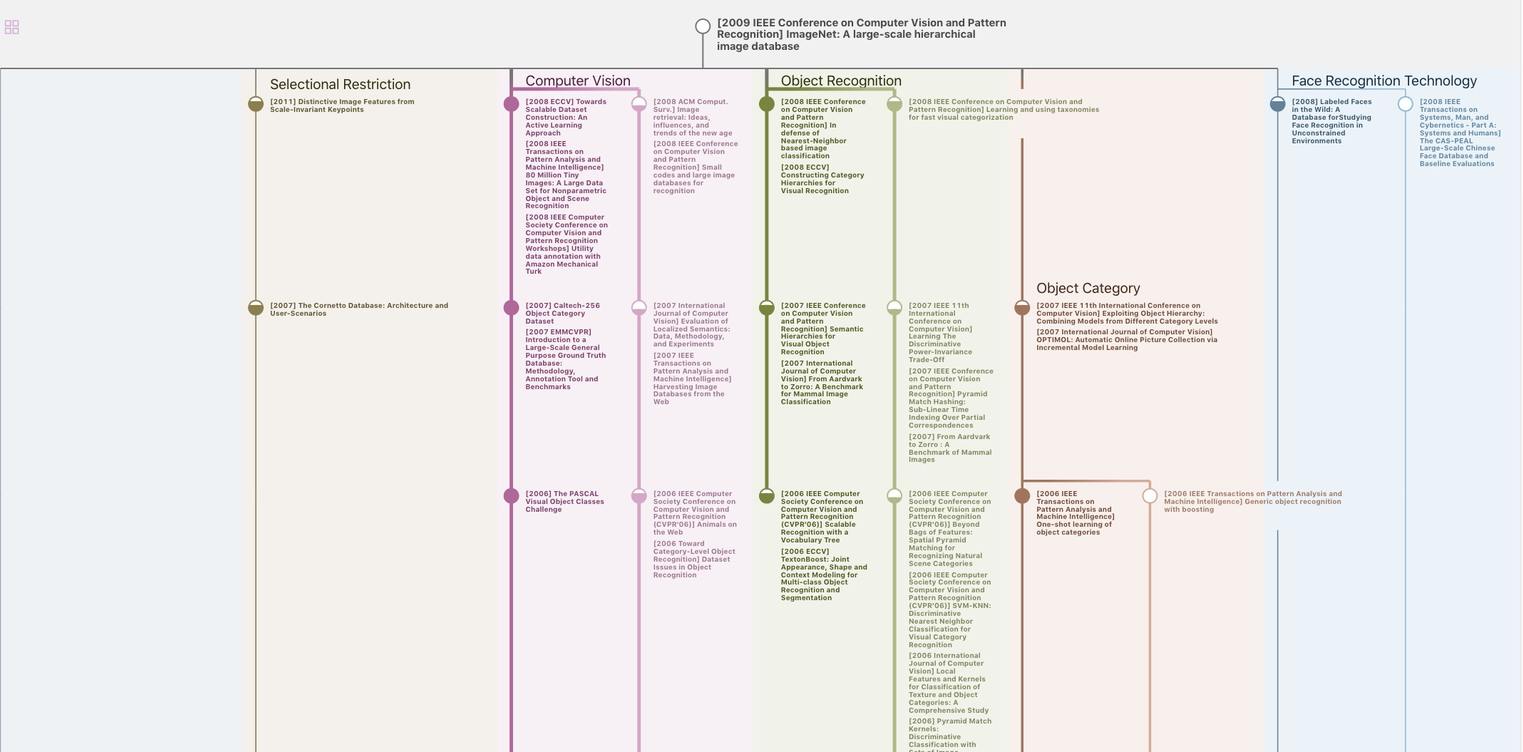
生成溯源树,研究论文发展脉络
Chat Paper
正在生成论文摘要