Tensor Rnn With Bayesian Nonparametric Mixture For Radar Hrrp Modeling And Target Recognition
IEEE TRANSACTIONS ON SIGNAL PROCESSING(2021)
摘要
To deal with the temporal dependence between range cells in high resolution range profile (HRRP), dynamic methods, especially recurrent neural network (RNN), have been employed to extract features for target recognition. However, RNN has difficulty in complex and diverse sequence modeling problems as it ignores non-stationary sequential relationship between time-steps by sharing same parameters among all time-steps. Given this issue, we propose tensor recurrent neural network with Gaussian mixture model (GmTRNN) for HRRP, not only making use of temporal characteristic but also modeling the variation among its patterns. Specifically, a novel tensor RNN is developed by extending all the parameters in the form of tensor to explore diverse temporal dependence between range cells within an HRRP sample, where a mixture model is introduced to determine the parameters of each time-step in tensor RNN. Moreover, to take advantage of Bayesian nonparametrics in handling the unknown number of mixture components, we further propose the tensor recurrent neural network with Dirichlet process mixture (DPmTRNN). For scalable and joint training of clustering and recognition, we present effective hybrid online variational inference and stochastic gradient descent method. Experiments on benchmark data, measured and simulated HRRP data demonstrate the the effectiveness and efficiency of our models and its robustness to HRRP shift.
更多查看译文
关键词
Tensors, Hidden Markov models, Recurrent neural networks, Radar, Data models, Target recognition, Indexes, Radar automatic target recognition (RATR), high resolution range profile (HRRP), Gaussian mixture model (GMM), Dirichlet process mixture, tensor recurrent neural network (TRNN), online variational inference, stochastic gradient descent
AI 理解论文
溯源树
样例
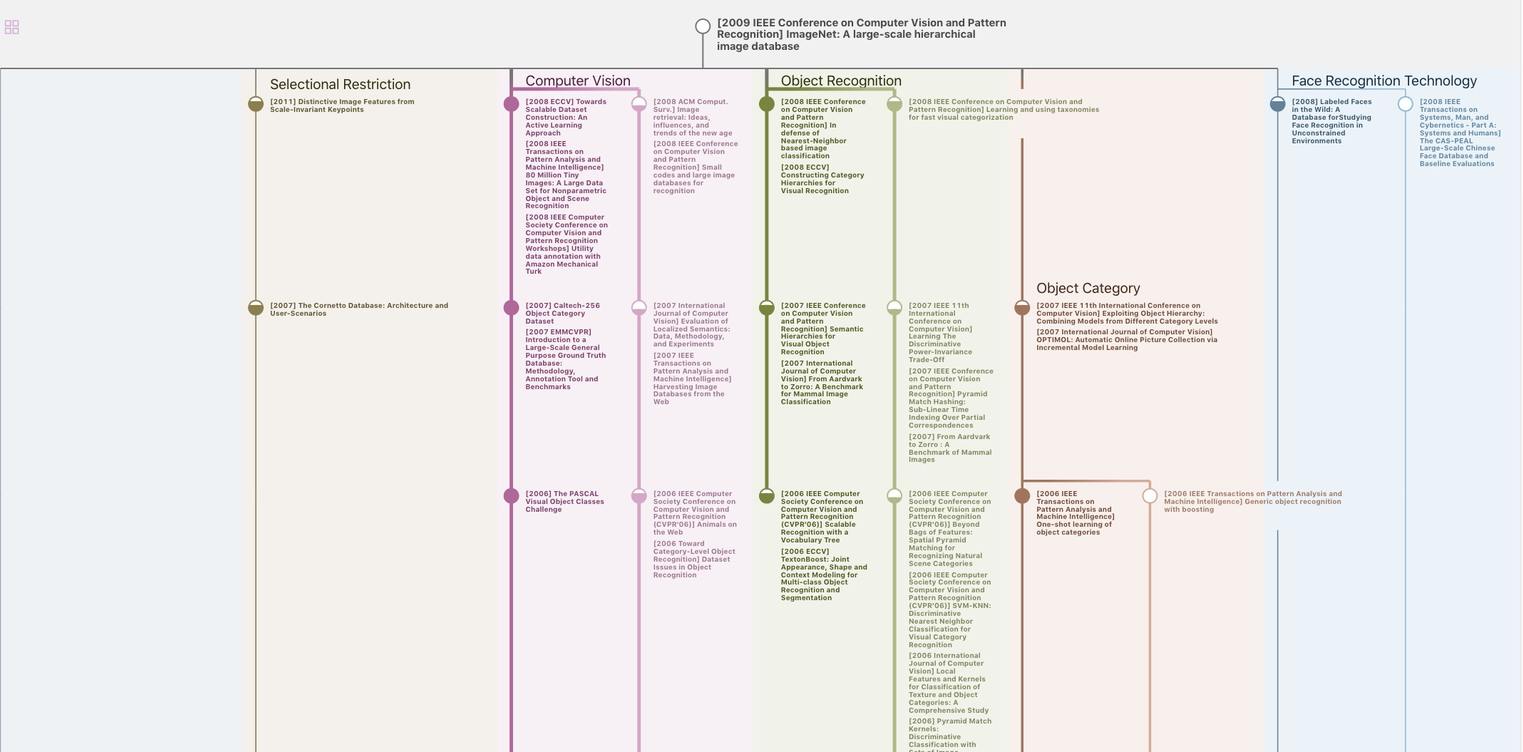
生成溯源树,研究论文发展脉络
Chat Paper
正在生成论文摘要