A Supervised Similarity Measure For Link Prediction Based On Knn
INTERNATIONAL JOURNAL OF MODERN PHYSICS C(2021)
摘要
As a research hotspot of complex network analysis, link prediction has received growing attention from various disciplines. Link prediction intends to determine the connecting probability of latent links based on the observed structure information. To this end, a host of similarity-based and learning-based link prediction methods have been proposed. To attain stable prediction performance on diverse networks, this paper proposes a supervised similarity-based method, which absorbs the advantages of both kinds of link prediction methods. In the proposed method, to capture the characteristics of a node pair, a collection of structural features is extracted from the network to represent the node pair as a vector. Then, the positive and negative k-nearest neighbors are searched from existing and nonexisting links, respectively. The connection likelihood of a node pair is measured according to its distances to the local mean vectors of positive and negative k-nearest neighbors. The prediction performance of the proposed method is experimentally evaluated on 10 benchmark networks. The results show that the proposed method is superior to the compared methods in terms of accuracy and stableness.
更多查看译文
关键词
Complex network, link prediction, node similarity, k-nearest neighbors
AI 理解论文
溯源树
样例
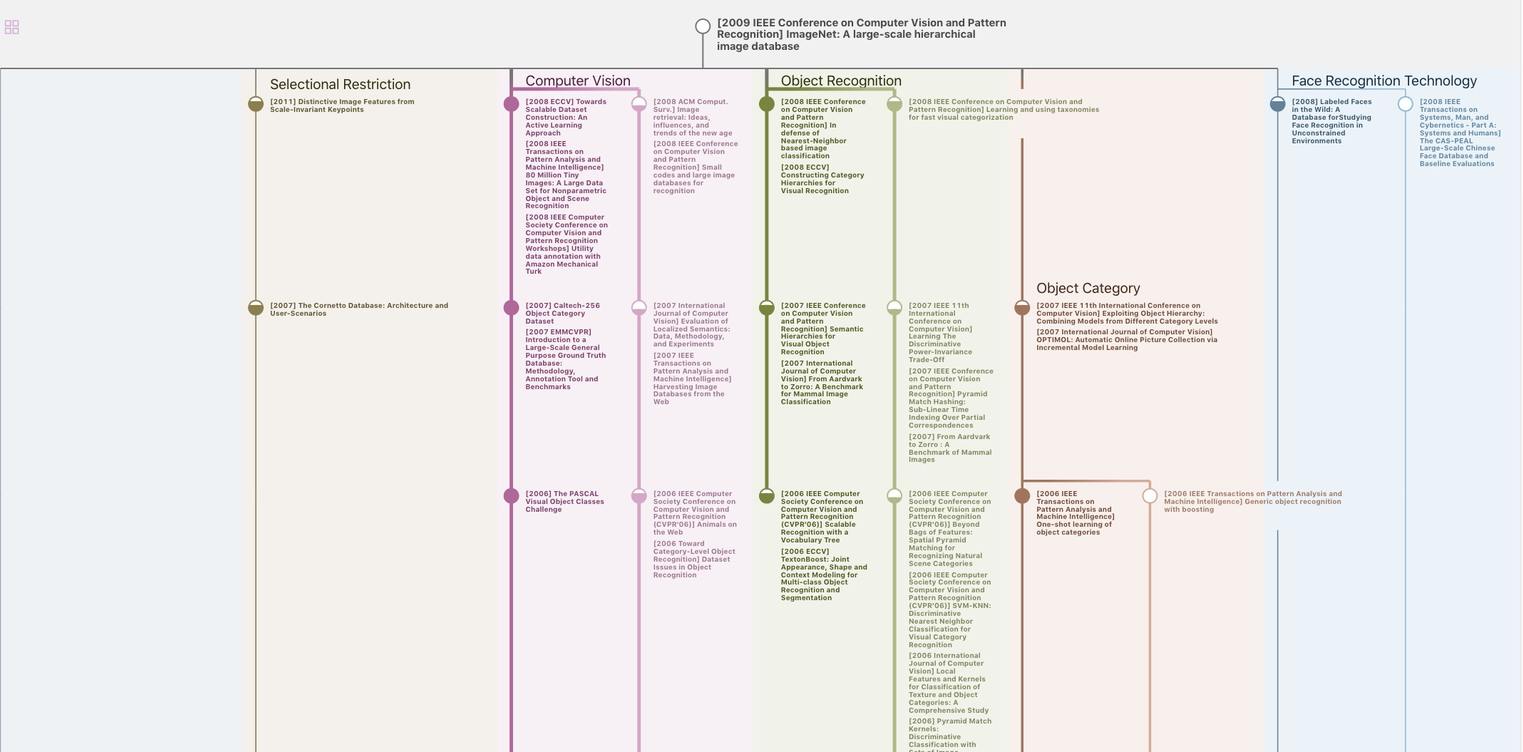
生成溯源树,研究论文发展脉络
Chat Paper
正在生成论文摘要