Intermediate-term Results of a Prospective, Multicenter Study on Remote Programming Sacral Neuromodulation for Refractory Overactive Bladder.
Translational andrology and urology(2021)
关键词
Sacral neuromodulation (SNM),remote programming,overactive bladder (OAB)
AI 理解论文
溯源树
样例
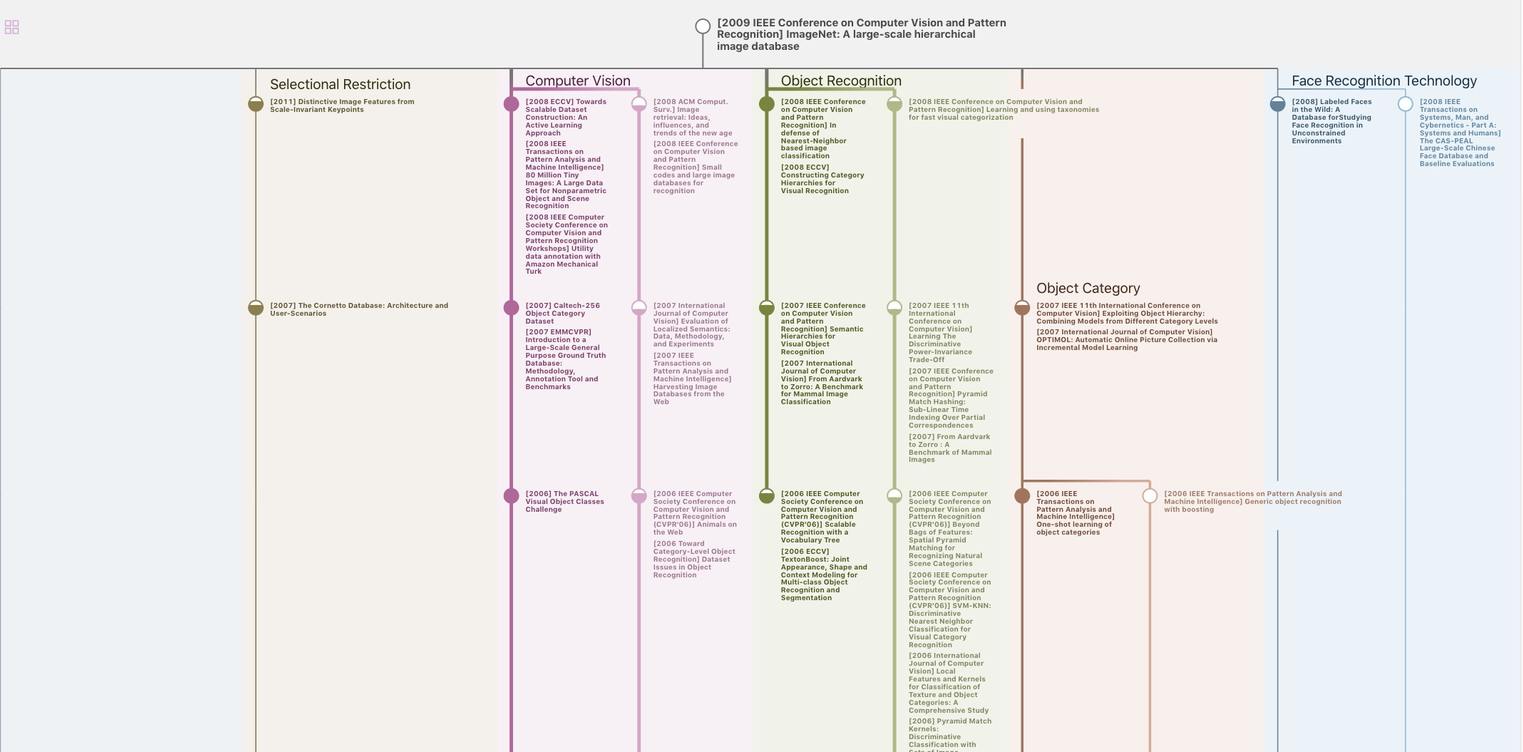
生成溯源树,研究论文发展脉络
Chat Paper
正在生成论文摘要