Multi-modal global surveillance methodology for predictive and on-demand characterization of localized processes using cube satellite platforms and deep learning techniques
Remote Sensing Applications: Society and Environment(2021)
摘要
Cube satellites (CubeSats) present a unique platform for monitoring localized processes anywhere within the Earth's surface or atmosphere using novel data analysis techniques. A CubeSat system equipped with adequate sensors and data analytics capabilities can provide an autonomous characterization surveillance method for the phenomena of interest. CubeSats are advantageous over conventional satellites in certain remote monitoring applications because of their reduced costs and higher simplicity due to the availability of commercially-off-the-shelf components. The CubeSat surveillance system developed in this paper focused on phenomena of interest surrounding the nuclear fuel cycle in support of nuclear non-proliferation and emergency response. To observe the phenomena, a constellation of 3U and 6U CubeSats deployed from the ISS with adequate components was chosen. Four different sensor configurations were identified for remote sensing: panchromatic/multispectral in the visible and near-infrared spectrum, multispectral in infrared spectrum, hyperspectral in infrared spectrum, and multispectral in ultraviolet spectrum. While a panchromatic/multispectral sensor configuration has CubeSat flight heritage at the required spatial resolutions, the other three sensor types need future development to meet signature and system requirements. Once each sensor onboard the CubeSat system collects data on a target of interest, the onboard computers would then apply the deep learning-based characterization methodology developed in this paper to identify phenomena. Four surrogate datasets containing representative simplified “images” were created for each sensor type to train the characterization methodology. A convolutional neural network was applied to each dataset and produced recall rates for the phenomena between 89.7% and 99.3% and precision rates between 92.3% and 99.9%. Each phenomenon's presence probability from each network is then combined into a final characterization solution for a target area. This paper covers multiple interdisciplinary areas to develop the foundation for a CubeSat surveillance system focused on phenomena surrounding the nuclear fuel cycle.
更多查看译文
关键词
Remote,Sensing,CubeSat,Nuclear,Surveillance,Characterization,Autonomous,Deep learning,Phenomena of interest,Convolutional neural network,Multi-modal
AI 理解论文
溯源树
样例
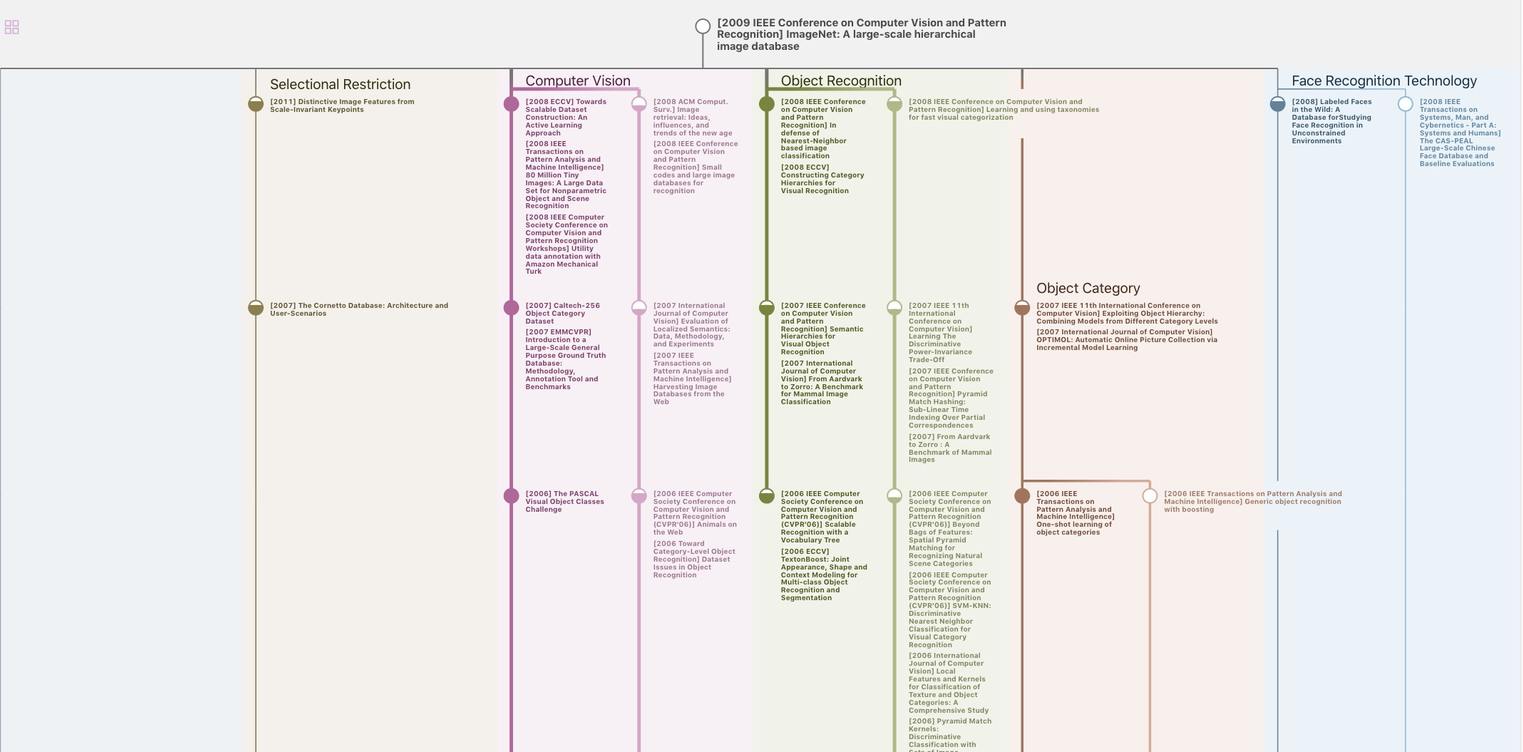
生成溯源树,研究论文发展脉络
Chat Paper
正在生成论文摘要