Optimizing Spatial Shift Point-Wise Quantization
IEEE ACCESS(2021)
摘要
It is no longer an option but a necessity to enhance the efficiency of deep learning models regarding energy consumption, learning time, and model size as the computational burden on deep neural networks increases. To improve the efficiency of deep learning, this study proposes a lightweight spatial shift point-wise quantization (L-SSPQ) model to construct a ResNet-like CNN model with significantly reduced accuracy degradation. L-SSPQ adds efficiency with the last linear layer weight reduction technology to SSPQ, which combines compact neural network design and quantization technology. To reduce weight and optimize performance, the learning time and system-required resources in the L-SSPQ are minimized. Accuracy could be improved with the warm-up interval and a step-size optimal value, both of which are hyper-parameters of the cosine learning rate. A two-stage optimization method that divides quantization learning into two steps is applied to further minimize loss. The size of the proposed L-SSPQ50 model is only 3.55 MB with an accuracy loss rate of 2.42%. This is just 3.56% of the size of ResNet50. In addition, the L-SSPQ50 score was 1.318 for information density, surpassing the SOTA models, including MobileNet V.2, MobileNet V.3, ReActNet-A, and FracBNN.
更多查看译文
关键词
Quantization (signal), Computational modeling, Biological system modeling, Convolution, Deep learning, Data models, Training, Compact neural network design, quantization, lightweight modeling, convolution neural network
AI 理解论文
溯源树
样例
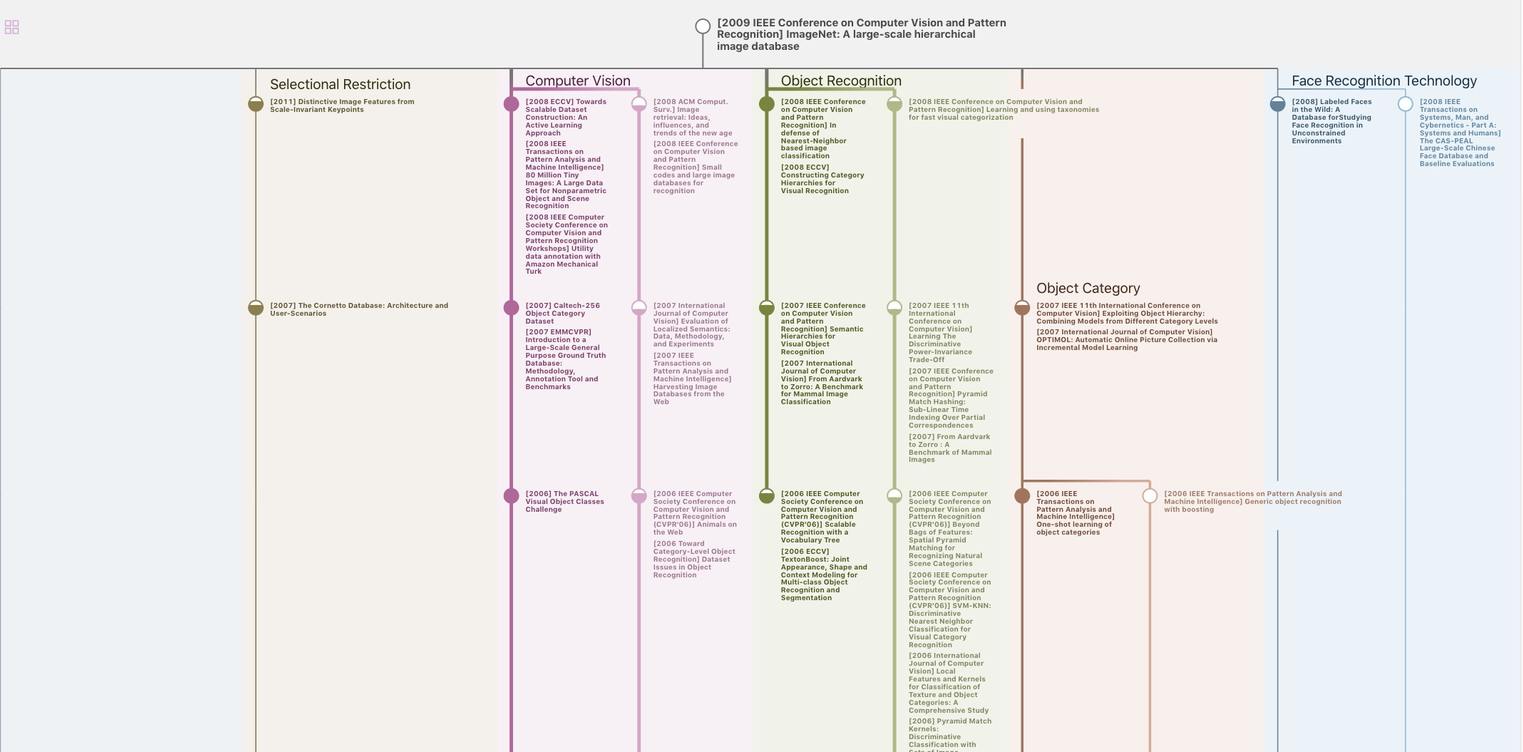
生成溯源树,研究论文发展脉络
Chat Paper
正在生成论文摘要