An Adaptive Multilayer Feature Fusion Strategy For Remote Sensing Scene Classification
REMOTE SENSING LETTERS(2021)
摘要
Remote sensing scene classification (RSSC) is one of the fundamental and challenging tasks for remote-sensing understanding and interpretation. How to construct discriminative features is crucial for scene classification. It is generally acknowledged that integrating convolutional features from different layers can significantly improve scene classification performance. However, some existing methods directly concatenate the high-layer and low-layer features without considering feature redundancy, semantic ambiguity and background noise, resulting in sub-optimal performance. In this paper, inspired by the attention mechanism, we propose a simple but effective strategy to fuse different convolutional features after feature selection operation, instead of directing concatenation. The basic idea of the feature selection operation is that we need to fuse those low-layer features, which are consistent with high-layer semantics. In particular, the proposed strategy is flexible and can be embedded into many neural architectures. Experimental results on two benchmark datasets demonstrate that the proposed method can obtain more valuable features and achieve competitive performance than other scene classification approaches.
更多查看译文
关键词
Multilayer feature fusion, feature selection, remote-sensing scene classification (RSSC), convolutional neural networks (CNNs)
AI 理解论文
溯源树
样例
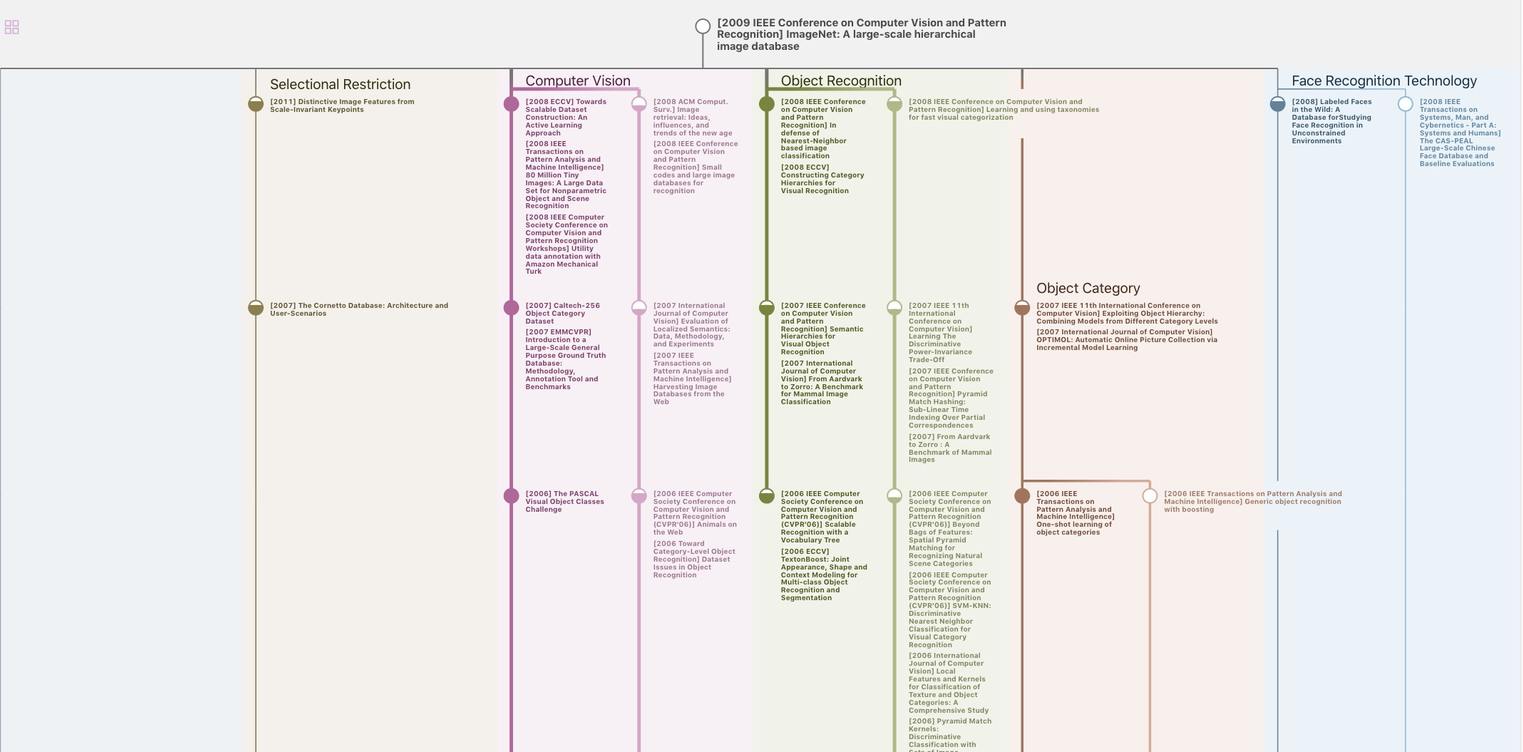
生成溯源树,研究论文发展脉络
Chat Paper
正在生成论文摘要