An unsupervised data mining strategy for performance evaluation of ground source heat pump systems
SUSTAINABLE ENERGY TECHNOLOGIES AND ASSESSMENTS(2021)
摘要
This paper presents an efficient data mining strategy to reveal the operational problems of ground source heat pump (GSHP) systems. Symbolic Aggregate approXimation was employed to identify typical daily load patterns and provide a reference for data partition. Kernel Density Estimation was used to evaluate overall system performance in different operation patterns identified. To find the root causes of inefficient operations, a customized association rule mining model was developed to discover the associations among different attributes. Lastly, the inference tree technique was applied to demonstrate the discovered associations for better comparative analysis. This strategy was evaluated using one-year operational data of a GSHP system. It was found that only 25% of the operations of this GSHP system in cooling seasons during 00:00-06:00 were considered as energy efficient, while above 79% of the operations in heating seasons were considered as energy efficient. Excessive power consumption of the water pumps due to improper control resulted in poor system COP (i.e. less than 2.0 or even 1.4 in cooling seasons and less than 2.1 in heating seasons). The results showed that this strategy can efficiently discover useful information from the operational data and identify energy conservation opportunities of GSHP systems.
更多查看译文
关键词
Data mining,Symbolic Aggregate approXimation,Association rule mining,Ground source heat pump,Sustainable development
AI 理解论文
溯源树
样例
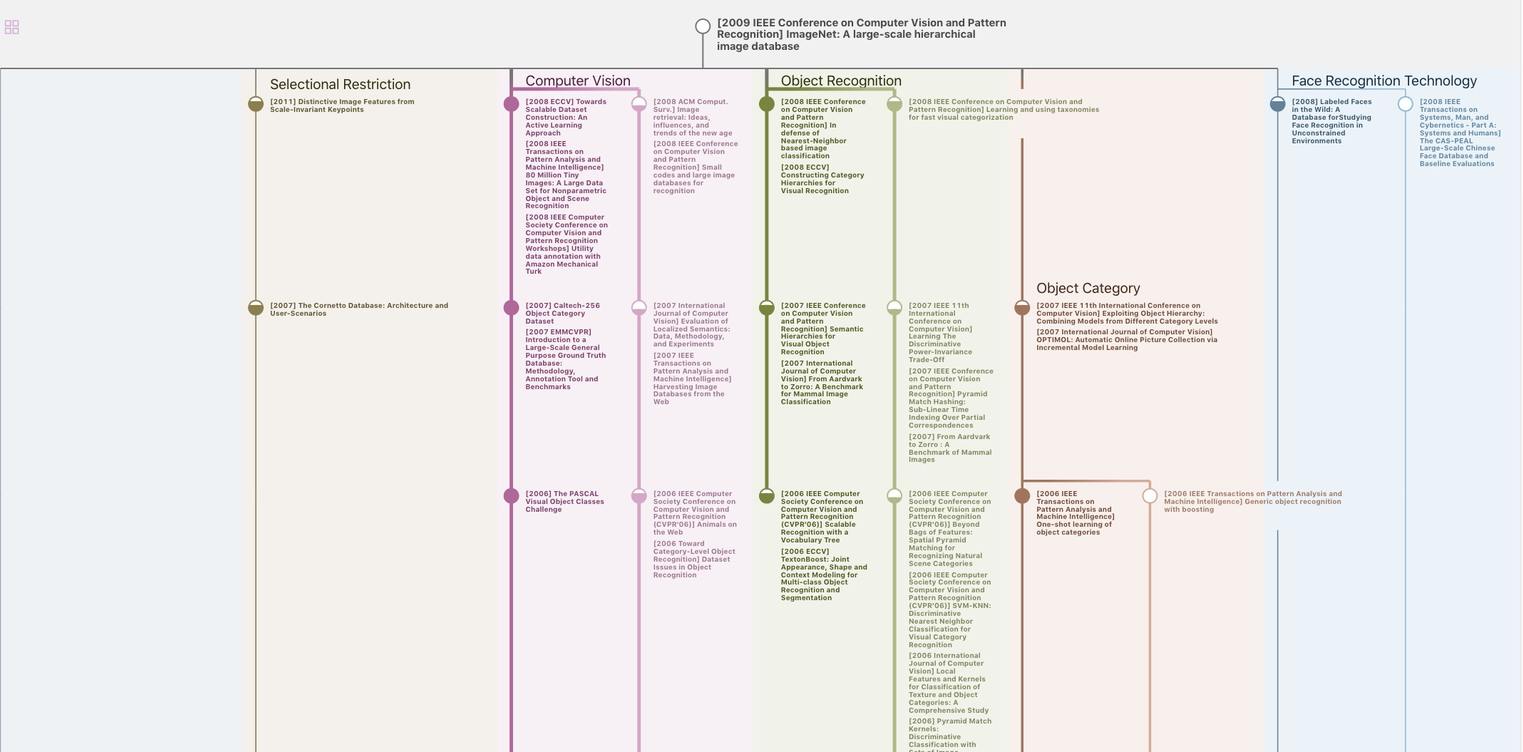
生成溯源树,研究论文发展脉络
Chat Paper
正在生成论文摘要