Grammatical Evolution For Constraint Synthesis For Mixed-Integer Linear Programming
SWARM AND EVOLUTIONARY COMPUTATION(2021)
摘要
The Mixed-Integer Linear Programming models are a common representation of real-world objects. They support simulation within the expressed bounds using constraints and optimization of an objective function. Unfortu-nately, handcrafting a model that aligns well with reality is time-consuming and error-prone. In this work, we propose a Grammatical Evolution for Constraint Synthesis (GECS) algorithm that helps human experts by synthe-sizing constraints for Mixed-Integer Linear Programming models. Given relatively easy-to-provide data of avail-able variables and parameters, and examples of feasible solutions, GECS produces a well-formed Mixed-Integer Linear Programming model in the ZIMPL modeling language. GECS outperforms several previous algorithms, copes well with tens of variables, and seems to be resistant to the curse of dimensionality.
更多查看译文
关键词
Mathematical programming, Model acquisition, Constraint learning, High-level modeling language, Operations research
AI 理解论文
溯源树
样例
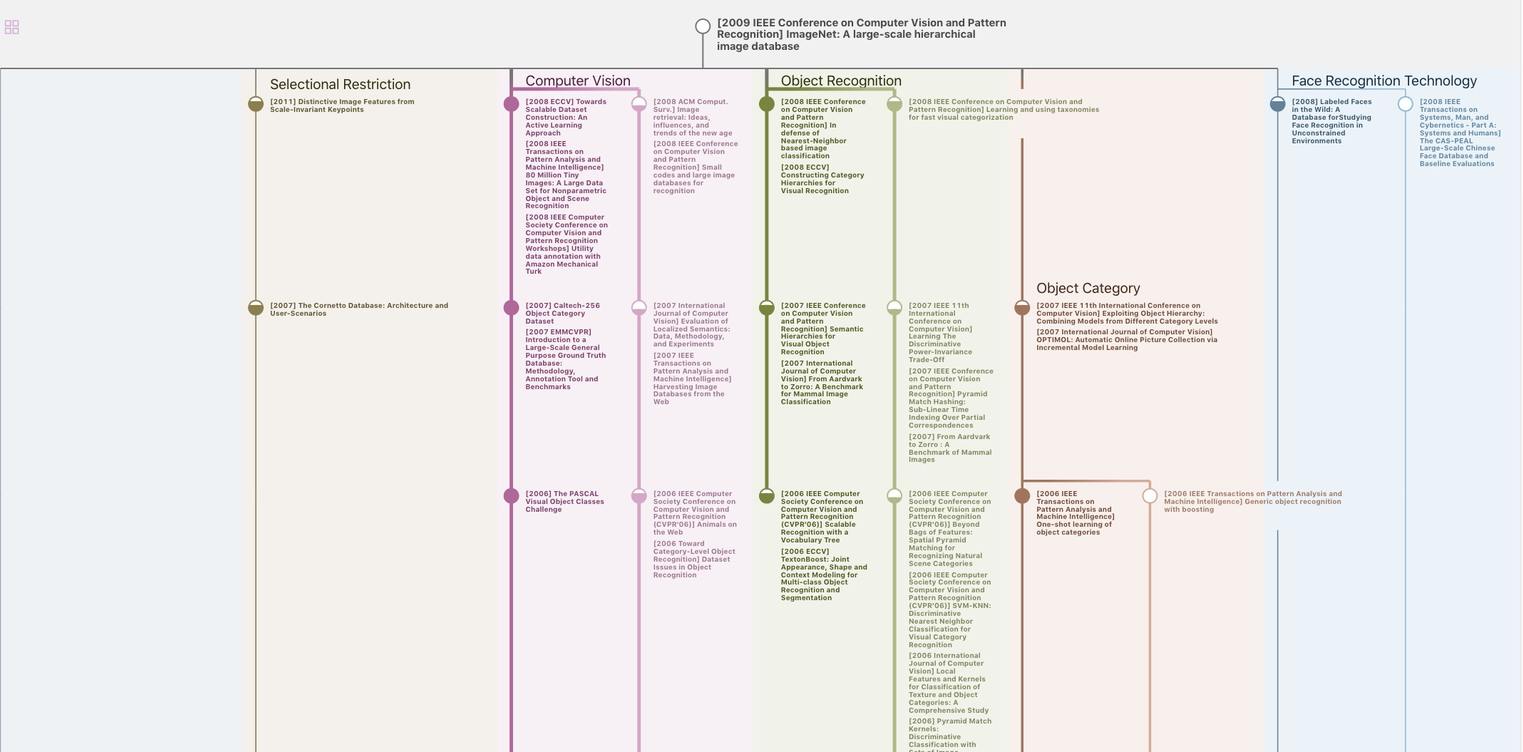
生成溯源树,研究论文发展脉络
Chat Paper
正在生成论文摘要