Online Zero-Cost Learning: Optimizing Large Scale Network Rare Threats Simulation
IEEE Transactions on Mobile Computing(2023)
摘要
To provide fast and accurate risk evaluation on network rare threats, importance sampling (IS) is widely used in the rare threat simulation; however, it becomes costly to deal with
many rare threats
simultaneously. For example, a rare threat can be the failure to provide quality-of-service (QoS) guarantees to a critical network flow. Considering network providers often need to deal with many critical flows (i.e., rare threats) simultaneously, if using IS, network providers have to simulate each rare threat with its customized importance distribution individually. To reduce such simulation cost, we propose an efficient mixture importance distribution to simulate multiple rare threats, and then formulate a mixture importance sampling optimization problem (MISO) to select the optimal mixture. We first show that it is challenging to locate the optimal mixture for the “
search direction
” is computationally expensive to evaluate. We then formulate an online learning (OL) framework to estimate the “
search direction
” and learn the optimal mixture from simulation samples of threats. And our OL framework has a “
zero learning cost
” as the samples generated in the learn phase can be reused to provide accurate estimation on the rare threats. We develop two multi-armed bandit OL algorithms so as to: (1) Minimize the sum of estimation variances with a regret of
$(\ln T)^2 {/} T$
; and (2) Minimize the simulation cost with a regret of
$\sqrt{\ln T {/} T}$
, where
$T$
denotes the number of simulation samples. We demonstrate the versatility of our method on different network applications. When compared with the uniform mixture IS, our method reduces cost measures (i.e., sum of estimation variances and simulation cost) by as high as 61.6 percent in the Internet backbone network scenario.
更多查看译文
关键词
Large scale network rare threats simulation,mixture importance sampling,online learning
AI 理解论文
溯源树
样例
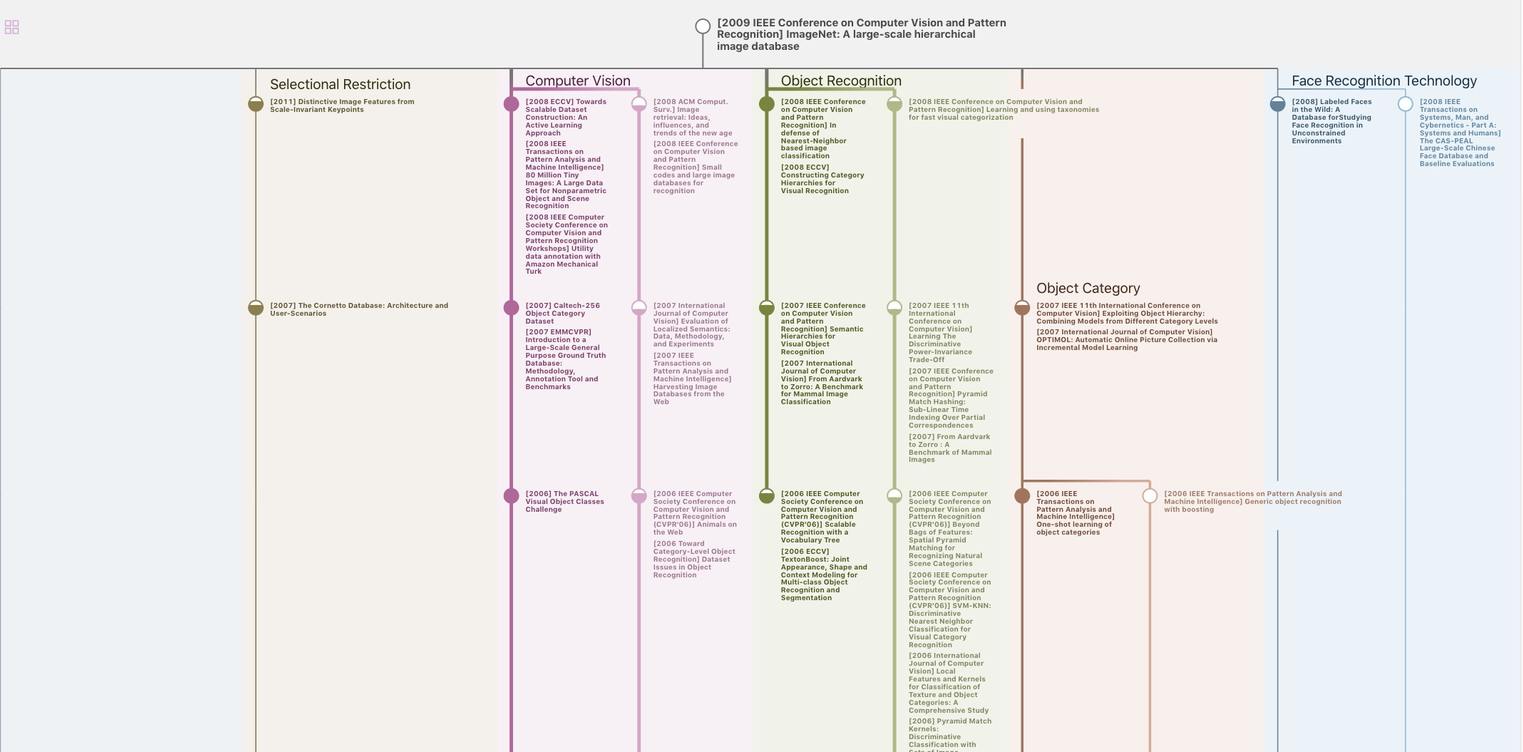
生成溯源树,研究论文发展脉络
Chat Paper
正在生成论文摘要