Blind Motion Deblurring Via L-0 Sparse Representation
COMPUTERS & GRAPHICS-UK(2021)
摘要
The method of blind deblurring based on machine learning can effectively deal with the blurred images in the real world. However, existing multi-level architectures can lead to problems such as the inability to reserve edges, the expected introduction of artifacts and ghosts when deblurring. Multi methods have found that using L-0 norm to realize image sparse representation can help keeping main structure of images. In this paper, an edge extraction module based on L-0 sparse representation is proposed to preserve the edge of images, which is embedded in a multi-scale recurrent network(SRN). When the current scale transmits information to the next scale, edge enhancement is performed using the edge extraction module. Furthermore, considering the correlation among pixels and the correlation among channels, we introduce dual-attention mechanism into the encoder-decoder structure. The deblurring experiment was carried out on the GOPRO dataset. Comparing with 5 state-of-art methods qualitatively and quantitatively, the experimental results show that the proposed method can better preserve the image edges and effectively avoid the artifact of the image. And the peak signal-to-noise ratio of the proposed method are improved compared with other methods. (C) 2021 Elsevier Ltd. All rights reserved.
更多查看译文
关键词
Image deblurring, Scale-recurrent network, Dual-attention mechanism, L-0 norm
AI 理解论文
溯源树
样例
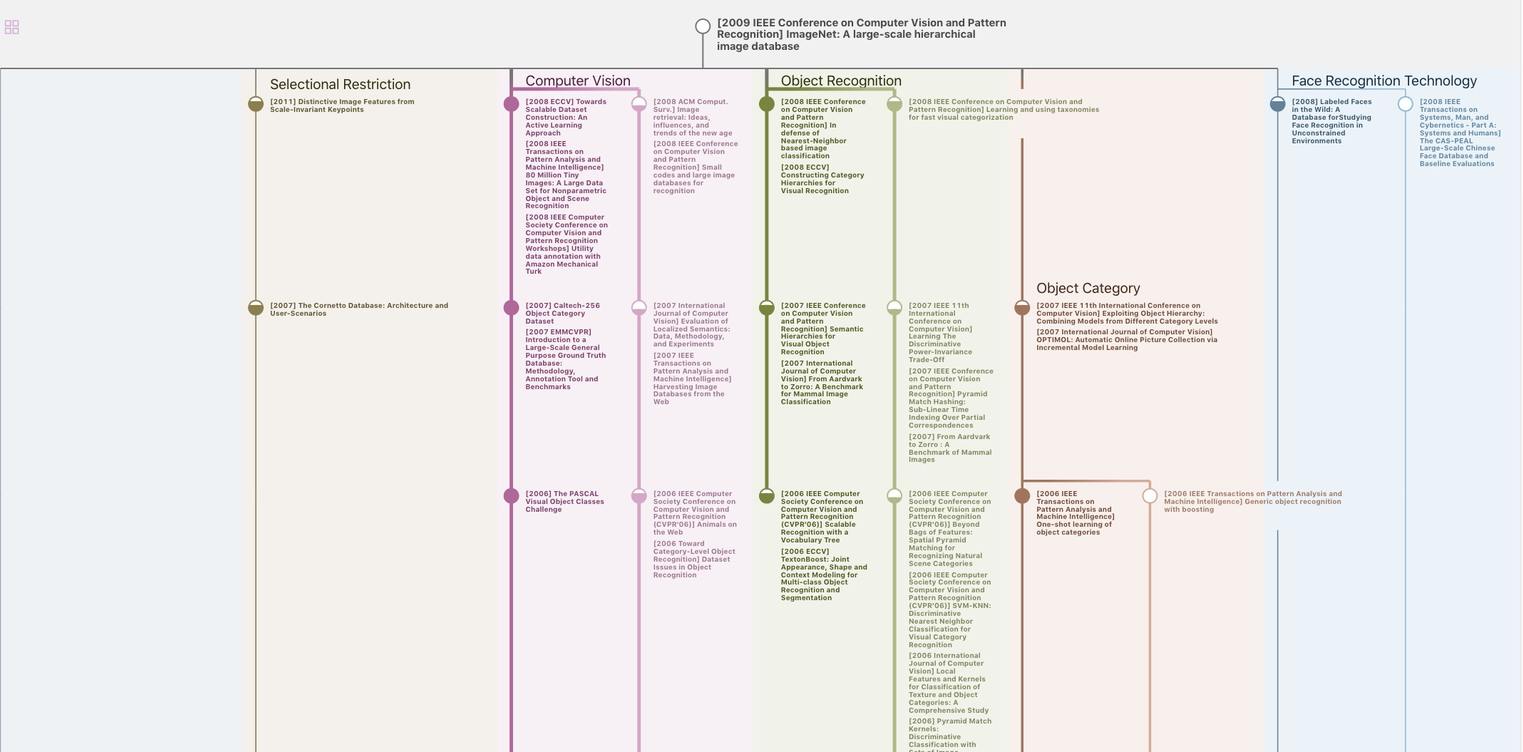
生成溯源树,研究论文发展脉络
Chat Paper
正在生成论文摘要